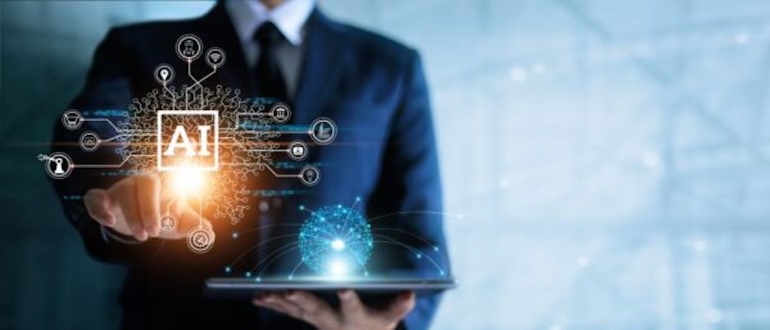
Generative artificial intelligence (AI) in the current technological landscape is a force to be reckoned with. GenAI technologies have moved beyond experimental curiosities to become critical tools for enterprise transformation. For instance, Marvel Studios uses generative AI to conceptualize new character designs and storylines, accelerating the creative process while keeping artists in the loop. NASA is leveraging AI-driven simulations to predict the weather in space and design more efficient spacecraft components. Amazon has rolled out AI coding tools to assist developers in writing code more efficiently, enhancing productivity while maintaining human oversight. From automated content creation to complex problem-solving, these technologies offer mind-bending capabilities that dramatically enhance operational efficiency, creativity and decision-making.
But, for those starting out, successful integration of GenAI will require more than just enthusiasm. Adoption demands a strategic, measured approach that balances technological potential with practical considerations.
Ins and Outs on the Ethical, Technical and Strategic Considerations
Organizations must conduct comprehensive risk assessments beyond surface-level technological considerations when considering implementation. This involves a deep dive into potential vulnerabilities surrounding data privacy, algorithmic bias and regulatory compliance. The most successful approaches take a holistic view that examines technological capabilities, broader organizational implications and potential transformative impacts.
During a recent appointment at a Fintech company, I instituted a deliberate separation of effort between the focus on developing commercialized AI-native products and transforming the company to be an AI-native operation. For the latter, one of the first steps was creating an AI integration plan that considered strategic company goals.
Developing an ethical framework is fundamental to responsible AI integration. Companies must establish clear guidelines that ensure these technologies are used responsibly, transparently and with genuine consideration for potential societal and organizational impacts. This means creating protocols that address complex concerns such as fairness, accountability and possible unintended consequences.
Infrastructure planning represents yet another dimension of generative AI adoption. Businesses need flexible, scalable technological ecosystems that adapt to rapidly-evolving AI capabilities. This demands an investment in cloud computing resources, sophisticated data management systems and agile technological architectures that can pivot and grow alongside emerging technological opportunities.
Successful generative AI adoption follows a measured, incremental strategy. Organizations should begin with carefully selected pilot programs demonstrating clear value while minimizing potential disruption. This approach allows controlled exploration and learning, providing opportunities to refine strategies and build institutional understanding before broader implementation.
Strategic Talent Development, Technology Selection and Risk Mitigation
- Talent development emerges as a crucial complementary strategy. Investing in training programs that help the existing workforce understand and effectively collaborate with AI technologies can transform potential technological challenges into organizational strengths. This means creating learning environments that demystify AI, build technological confidence and encourage innovative thinking.
- Technology selection requires prudent evaluation. Not all generative AI solutions are the same, and businesses must develop strict vendor and platform selection criteria. Considerations should include alignment with specific business objectives, proven reliability, comprehensive security features and demonstrated flexibility in integrating existing technological ecosystems.
- Businesses must remain vigilant about potential challenges. AI safety and data security demand rigorous governance protocols, including strict access controls, advanced encryption standards and comprehensive monitoring systems. Moreover, organizations must commit to ongoing algorithmic bias mitigation through regular system audits and a commitment to accuracy and fairness.
- A common blindspot is misjudging the risk controls. When implementing new systems, and in this case, successfully rolling out AI systems in a timely manner, it’s important to execute in a staged approach – one that allows innovation to continue whilst adequately mitigating risks. For many processes, transitioning to a partially AI-automated system with a human in the loop is often a better risk-managed approach than full automation. At the aforementioned Fintech company, automated processes were used with a human in the loop to ensure concerns related to regulatory compliance and governance protocols were addressed before generative AI was used to automate fully.
Looking toward the future, generative AI represents more than a technological tool. It reimagines how work can be conceptualized, executed and optimized. The most successful organizations view these technologies as collaborative partners that enhance human creativity and problem-solving capabilities rather than replacements for human intelligence.
Emerging trends suggest we are moving toward increasingly context-aware AI systems capable of understanding and responding to complex organizational dynamics with remarkable sophistication. The organizations that will truly thrive approach generative AI not as a technological add-on but as a strategic innovation and competitive differentiation.