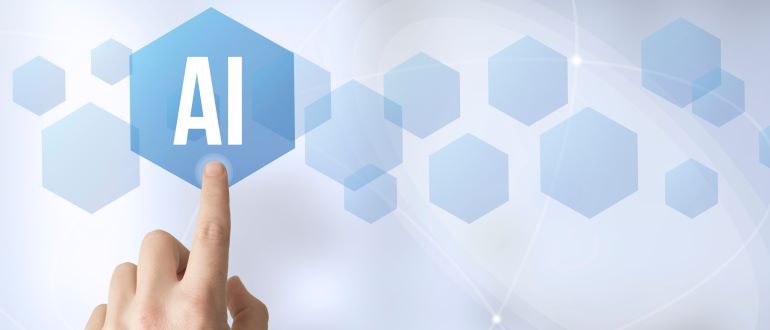
The enterprise benefits of generative AI are likely only limited by imagination. As we’ve previously covered, enterprises seek generative AI for content creation, systems automation, knowledge management, customer service, supply chain optimization and more. The rush is on because generative AI can gather new insights by analyzing existing patterns within data. For those organizations that successfully deploy generative AI, the new tools are being shown to help boost customer experience, enhance operational efficiency and drive growth.
However, deploying generative AI improperly can result in undesirable consequences. Perhaps the most problematic potential negative impact is the unauthorized disclosure of confidential information. This can lead to legal and security concerns, especially regarding data privacy. Additionally, if AI tools malfunction, they may create legal liabilities. Businesses must implement policies, processes and governance measures that optimize the upside while minimizing the business risks associated with AI tools.
We contacted experts to understand what steps enterprises must take to succeed.
Start small and pick the right pilot project
When selecting an AI pilot project, four tips include defining clear business outcomes, choosing the right AI approach that fits within their IT ecosystem, anticipating a learning curve, and understanding the difference between testing and production readiness. “To successfully adopt generative AI, [organizations] should start embracing the technology today, as it is not going anywhere. Leaders must step back and analyze what this technology can do for their organization and what the benefits are if they implement it. Targeted use cases will be key to early adoption,” advises Joe Atkinson, chief products and technology officer at PwC.
“In terms of strategies for generative AI success, we recommend that enterprises start small and focus on specific use cases that can provide immediate benefits,” continues Surya Sanchez, founder at DeepIdea Lab. “They should also invest in quality data and ensure that the AI models are continuously trained and updated to improve accuracy and reliability,” he adds.
On this point, Oleksandr Stefanovskyi, head of R&D at Intelliarts, agrees. “It’s recommended to focus on specific use cases where generative AI can be implemented in a particular business environment. Then, a company should consider building a minimum viable product with a trusted machine learning service vendor and then guide their decision-making based on customer feedback and financial outcomes. This strategy can help demonstrate the value of the technology and build momentum for broader adoption with low business risks,” Stefanovskyi says.
For longer-term success, enterprises must identify the areas that will provide the most intermediate value and invest in the necessary talent. “Depending on the results of generative AI technology usage in the short-term, further decision-making may focus on one of two approaches: Treating the existing technology implementation as a useful addition to the present IT infrastructure or expanding its inclusion in the business process more. In the latter case, it’s recommended to build in-house expertise in generative AI by hiring AI talent and providing training for existing staff,” adds Stefanovskyi.
Build a quality data baseline
Without a trove of quality data, no enterprise plans for a generative AI deployment are likely to succeed. Generative AI models can produce misleading results without accurate data, leading to poor decision-making. “Enterprises need to invest in quality data and ensure that the AI models are trained properly to avoid any pitfalls that could cost them success,” says DeepIdea Lab’s Sanchez.
One way to achieve the accurate and relevant data enterprises need is by using machine-learning-powered intelligent search services, such as Amazon Kendra. Such services source the most pertinent and enterprise-specific content for correct responses. And they can be secured through access control lists and integration with identity provider services. Another way to limit generative AI responses to enterprise data is through Retrieval Augmented Generation (RAG) techniques.
Intelliarts’ Stefanovskyi adds that it’s important to invest appropriately in data infrastructure, which, in addition to investments in data quality, includes building data pipelines and investing in high-performance computing resources. “Collaboration with industry partners and putting effort toward establishing best practices for technology adoption and evolution should also be the right call from the long-term perspective. In essence, the goal is to follow the natural trend of generative AI involvement rather than attempting to get the most out of it in the present day,” Stefanovskyi says.
Safeguard against bias and lapses in AI ethics
Enterprises can avoid bias in their generative AI projects by creating diverse and representative data sets and employing advanced bias detection and mitigation techniques. Transparency and explainability in AI models are also crucial. “We must overcome the public’s concerns about transparency and explainability to increase AI’s adoption,” says CF Su, VP of machine learning at Hyperscience.
“The importance of leading with an ethical framework cannot be overstated,” Su continues. “In the near term, enterprises should prioritize creating an AI ethics committee focusing on internal and external communication. Ethics committees help keep organizations honest when developing new technology and provide alignment on protecting the public against potentially harmful applications,” he says.
“It’s incredibly important that these committees engage with people outside the organization, from regulators to customers, to define rules that protect individuals and gather critical feedback. In the long run, leading with transparency will help to build public trust in your company and technology, which is the best way to ensure a strong customer experience,” adds Su.
Finally, when creating an ethics committee, leading with transparency–both inside and outside the organization–is essential to accelerate trust in your strategy. “Following the White House’s AI Bill of Rights as a guide is a solid first step. I’d recommend assessing the bill’s primary areas as a ‘sniff test’ to determine whether your company’s generative AI use cases are ethical,” says Su.Â
Secure the GenAI system and data
Enterprises must implement policies, processes and governance measures that minimize the business risks of using AI tools. This includes regular audits and monitoring of AI systems to assess their performance and identify potential security threats. Companies should conduct a risk analysis, evaluate potential risks, and ensure humans are involved in decision-making. Governance frameworks are needed to manage AI projects, tools, and teams to minimize risk and ensure compliance with regulations and guidelines.
Businesses must be proactive here, embed security and governance controls into their processes, and enhance their data loss protection controls at their endpoints and perimeter.
Develop an effective user interface
A good UI for generative AI should prioritize effective and efficient user experience. Ultimately, the UI should be user-friendly and intuitive, enabling users to interact with the generative AI easily. “The UI/UX must be very strong and differentiated. Usually, this means
substantial integration with the tools and processes already in place,” advises Brandon Jung, VP of ecosystem at Tabnine.
Perhaps the most important thing to remember is that AI isn’t always a replacement for human input. “We must remember that AI is not a replacement for people,” says Don Schuerman, CTO at Pegasystems. “Generative AI solutions can provide impressive outputs, but everyone is responsible for ensuring the results are explainable, approachable and maintainable. There must be human gatekeepers in place to assess the outcomes of the AI and ensure they are, in fact, in alignment with company goals.”