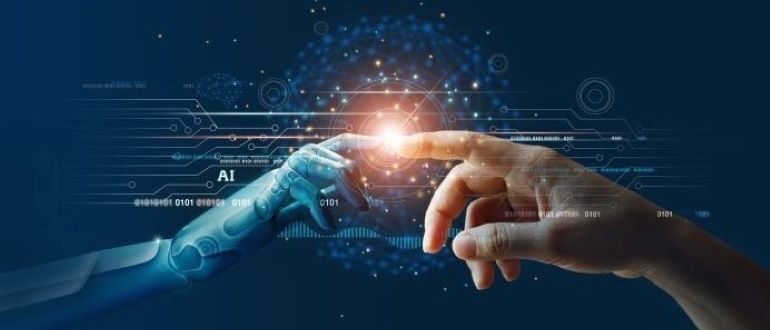
AI has been sweeping through the business world like a landslide and by now the inevitable transformation has become apparent even to businesses that are far removed from all things tech. However, for tech companies, AI integration has become an acute topic. The decision to adopt AI into operations and processes of such an established domain as software development is not easy for any company.
There are both challenges and opportunities, and as many ups as there are downs. AI integration offers the potential to revolutionize business processes, enhance decision-making and roll out innovation. At the same time, it is still largely a gray area with regulations that are barely emerging and break-neck development speed that is sometimes too fast to keep pace with. Embarking on this journey requires careful planning, strategic implementation and a clear understanding of the potential impacts on workflows and organizational culture. In this article, we discuss challenges, best practices and actionable tips on how to safely adapt AI into business.
Challenges Companies Face with AI Integrations
The journey towards AI integration is still largely a way of pioneers. Its challenges range from technical and infrastructural issues to ethical and organizational concerns. Companies often struggle to identify the right AI solutions that consider both their business needs and operational limitations. Then there is also the task of navigating the complex AI marketplace, selecting between custom-built solutions and external providers. Moreover, concerns about data privacy, security and ethical use of AI add layers of complexity to the integration process. And of course, the novelty of such epic proportions simply scares many people. There’s the need to overcome resistance to change within the organization. Let’s take a look at some of the formidable challenges AI integration poses for businesses.
- Technical and Infrastructural Challenges
To train AI models effectively, so that their outputs actually add value and not nonsense, large amounts of high-quality data are required. Many companies find it difficult to provide or to even evaluate whether the data is of sufficient quality. Data that is siloed, incomplete or of poor quality can hinder the performance of AI systems. Another issue is the ownership and rights to the use of data. There is a lot of discourse about AIs being trained using copyrighted, private or otherwise protected data.
Another technical challenge is the process of integration with existing systems and IT infrastructure of the business that wants to adapt AI. It usually requires additional resources and the process itself is quite complex.
Let’s not forget that since AI integration basically revolutionizes businesses on many levels, it needs to be scalable so that it can support business growth. This also becomes a challenge due to technical limitations and costs.
- Organizational and Cultural Challenges
Like any innovation that requires drastic and quick changes, AI transformation poses a specific type of challenge businesses have to deal with. For instance, change management. The introduction of AI can lead to significant changes in business processes and employee roles. Managing this change and overcoming resistance from employees who fear job displacement or significant alterations in their work routines is definitely a feat.
Another aspect of cultural challenges is the inevitable skill gap. Like we have already mentioned, AI development is extremely fast-paced and the majority of people across all business spheres are already out of the loop with it. There is often a gap between the skills available within an organization and the skills required to develop, deploy and maintain AI solutions. This gap can slow down AI adoption and limit the effectiveness of AI initiatives.
- Ethical, Legal and Privacy Challenges
AI systems can extrapolate biases if they are trained on biased data. Ensuring AI systems are fair and unbiased is a significant challenge that requires careful selection of data that you feed to the AI and objective analysis of outputs. Human-in-the-loop practices help to tackle it, however, questions of privacy and legal use of AI remain. Balancing the use of data for AI with privacy concerns and compliance requirements is literally a battlefield in today’s digital landscape.
Companies also must untie a tight knot of ethical considerations around the use of AI, including the potential for surveillance, manipulation and other harmful uses. And of course, there’s always the good old concern that is being blown up by mass media everywhere that AI will substitute human labor and become a cause of mass layoffs.
- Strategic and Operational Challenges
Last type, but far from the least. Since the AI sphere is still largely a novelty, many businesses literally tread in the dark when they are trying to identify use cases and measure ROI from the AI integration. There isn’t a lot yet to reference, and long-lasting effects of AI integration for anyone are yet to reveal themselves.
Dealing with these challenges may be intimidating for any business. We at Solvd already have accumulated experience with AI transformation for our own processes and for our customers and have elaborated a few actionable tips and best practices. Applying these principles helps you to make your AI transformation smooth and effective, saves you time and valuable resources and allows you to escape common pitfalls.
Best Practices of Implementing AI Integration
Successful AI integration hinges on adopting a strategic approach that encompasses several best practices:
- Start Small, Start Simple
Begin with pilot projects that address specific use cases. This allows for the testing of AI solutions on a manageable scale before wider implementation. This also helps you to showcase AI capabilities early on to stakeholders and employees of all levels in your company.
- AI Integration needs to align with Business Objectives
From the very conception, AI initiatives need to be aligned with the company’s overall business goals. Any AI enhanced processes must add value to the business and support its general development.
- Adapt a Culture of Knowledge Sharing and Learning
Encourage a culture that strives to experiment and values continuous learning. Upskilling and reskilling employees to work effectively with AI technologies is crucial. Empowering young professionals with internal projects is a great way to successfully upskill your staff and apply a sandbox approach for safe experiments.
- Develop Clear AI Policies and Guidelines
Establishing clear policies and guidelines around the ethical use of AI, data privacy and security is essential for building trust and ensuring responsible AI integration. Such guidelines should be implemented with a constant feedback loop, revised and updated regularly and shared and promoted across your company with a mandatory acknowledgement.
- Use a tandem of Subject Matter Experts and AI Facilitators
When working on enhancing your workflows and processes with AI, use a two-sided approach. On the one hand, AI implementation should be driven by a facilitator trained specifically in the use of AI. On the other hand, subject matter experts should take active part, offering insights from the point of view of their domain knowledge and insider view of the workflows and processes you want to upgrade with AI.
So, what kind of business processes can benefit from AI in a way that is both effective, but also safe, ethical and focuses on boosting human effort rather than substituting it? Let’s take a look at a particular example.
An Example of a Task that Can Be Boosted with AI Help
As an example, let’s take a look at a common task performed by BI analysts: Creating a user story. This task is on the one hand, standardized and repetitive, since user stories are something created en masse. On the other hand, it incorporates a huge amount of research, structuring and analysis. The 80%-20% rule applies here. 80% of the time working on this task, a BI analyst usually does preliminary work. And only 20% of the time they actually write a user story. Business analysts must gather requirements, identify potential issues and document specifications. With the help of AI, this process can be greatly enhanced. AI-powered tools can automate prep work, analysis and evaluation of various factors, ensuring they are complete, consistent and free of errors.
Provided that the AI workflow is well-programmed and the AI is specifically trained, a human business analyst in this case acts largely as a supervisor. This not only speeds up the development process by reducing the amount of repetitive manual work. It also frees the BI analyst for more sophisticated tasks and enhances the quality of the output by minimizing the potential for human error factor.
Conclusion
The integration of AI into business operations presents a promising avenue for companies to enhance efficiency, foster innovation and gain a competitive edge. While the journey is complex and features many gray areas, adopting a strategic approach and working with trusted experts saves you from many pitfalls.
By starting small, aligning AI initiatives with business objectives, fostering a culture of learning and developing clear policies, companies can navigate the challenges and harness the full potential of AI to enhance their business processes.