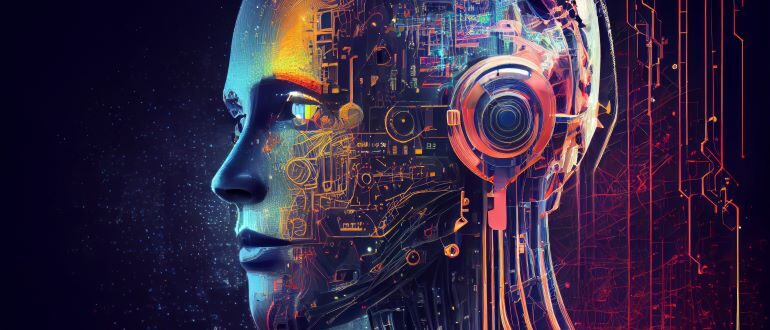
Artificial intelligence (AI) is one of the most transformative technologies of this generation, already reshaping industries through advancements in voice recognition, natural language processing (NLP) and content generation. To maximize AI’s potential, it is essential for companies to focus on high-impact use cases that enhance customer experience, generate revenue and reduce costs. Gartner predicts that by 2025, 30% of generative AI (GenAI) projects will be abandoned in the post-proof-of-concept phase due to poor data, unclear business value and misaligned strategies.
It’s imperative for chief technology officers (CTOs) and chief product officers (CPOs) to adopt a strategic, business-aligned approach to ensure AI initiatives deliver business value and drive sustainable competitive advantage.
Strategic Framework for Implementing AI Initiatives
The proposed framework for AI initiatives outlines a comprehensive, iterative process to implement AI initiatives effectively (Figure 1). The framework is divided into five phases:
- Define product strategy and objectives. The strategic framework is built on clear business objectives and a well-defined product strategy. Set objectives early to ensure that AI initiatives align closely with the company’s overall vision and goals. Establishing these clear, measurable targets ensures AI initiatives are guided effectively and focused on delivering value.
- Identify highly impactful use cases. Prioritize AI use cases that maximize customer value, generate revenue, open new markets and reduce costs.
- Develop a data strategy. Data is the foundation of machine learning (ML), and a strong data strategy is crucial for ensuring the accuracy, quality, performance, and scalability of AI solutions. Establish a robust data infrastructure that ensures data availability and quality, which are critical to AI success. A well-structured data strategy supports effective model training, enhances the reliability of AI outcomes, and prepares the organization for future scalability, making it a key component of the overall AI framework.
- Develop and implement ML models. Build and deploy ML models tailored to and designed to solve identified use cases.
- Evaluate, observe and optimize. Continuously monitor, refine and optimize models based on feedback and key performance indicators (KPIs) to ensure alignment with business goals.
Figure 1: AI Strategic Framework
The feedback loop of the process promotes continuous improvements that refine the strategies, data and model development. This ensures the AI system remains agile, adaptive and valuable.
Identifying Use Cases for AI Application
To effectively identify high-impact AI use cases, it’s important to blend customer-centered design thinking with a structured prioritization framework. The design thinking process encourages companies to empathize with user problems, define challenges, and engage in iterative ideation, prototyping, and testing. This divergent-convergent process ensures AI initiatives are focused on real-world customer needs and market demands, helping companies unearth valuable opportunities for innovation.
Once ideas are generated, the prioritization framework helps funnel those opportunities into actionable AI use cases by evaluating them based on five criteria: Impact and value, urgency, competitive differentiation, adoptability and feasibility.
CTOs and CPOs can consider five factors to determine which potential use cases will deliver optimal results:
- Impact and value. A worthwhile use case demonstrates high value to the customer and a meaningful impact on the company.
- Urgency. It illustrates a prevalent and immediate user and market need for a product.
- Competition. A beneficial use case builds sustainable competitive differentiation.
- Adoptability. The solution should satisfy a customer’s compliance, trust, explainability, ethics and accuracy requirements.
- Feasibility. A valuable use case has high technical feasibility for quality, accuracy, ethics, bias and security.
AI’s Evaluation, Observation and Risk Management
Legal and regulatory risks stem from evolving laws like the General Data Protection Regulation (GDPR) and the California Consumer Privacy Act (CCPA). Ethical and bias-related risks arise when algorithms unintentionally favor certain groups, leading to unfair outcomes. Intellectual property and copyright risks are also significant in GenAI, which may generate content based on copyrighted material. A robust AI governance framework—integrating risk management, compliance and ethical standards—is essential to mitigate these risks, foster responsible innovation and maintain stakeholder trust.
Risk type will vary by industry, however. For instance, data security and privacy are chief concerns in health care, while the financial sector contends with similar risks and concerns over compliance with industry regulations. Risk severity also fluctuates by industry. One 2024 study assessed emerging AI risks across 10 industries over the next decade. The analysis ranked IT services as the sector at the most risk while projecting that the health and pharmaceutical industry faced the greatest future risks between 2032 and 2034.
Microsoft lays out a framework for building AI systems ethically and safely while “guiding product development towards more responsible outcomes” with its Responsible AI Standard. The framework recommends several goals organizations can set when implementing AI systems as part of product development, such as data governance and management, including documenting data requirements as part of the AI impact assessment.
Data and AI Underpinning the Sustainable Competitive Advantage
A well-executed data and AI strategy can be harnessed as a flywheel for continuous innovation and long-term sustainable competitive differentiation. As more customers adopt AI-driven products, they generate valuable data, leading to improved algorithms and more efficient autonomous systems. This enhances customer outcomes, reduces costs through economies of scale, and strengthens the business’s negotiating power with vendors. The continuous feedback loop between data generation, data strategy, model improvement and operational efficiency creates a self-sustaining flywheel, helping companies build a durable competitive advantage over time. Solid, reliable data underpins successful AI-powered product development initiatives, ensuring ethical use and regulatory compliance and helping organizations from a competitive standpoint.
To drive successful AI initiatives, it’s critical for CTOs, CPOs and company leadership to adopt a strategic mindset that aligns AI efforts with core business objectives. This requires a focused approach to identifying high-impact use cases, ensuring AI resources are allocated effectively, and continuously refining strategies through data-driven insights. By following a structured framework, organizations can unlock AI’s full potential to innovate, optimize operations and stay ahead in a competitive market.