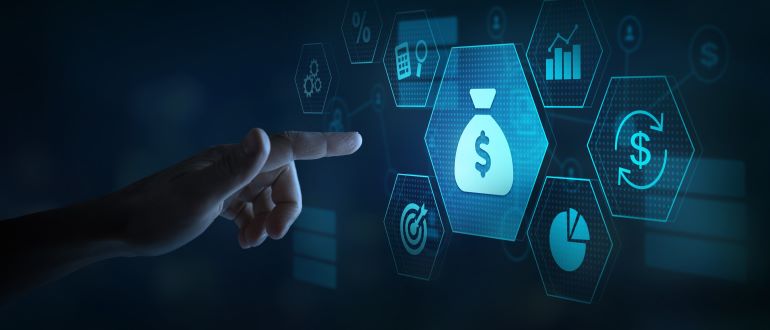
Businesses are grappling with increasing software costs, with nearly 80% reporting higher expenses over the past year, according to a Forrester report.
The surge in costs is largely attributed to the integration of new features, particularly AI capabilities–two-thirds of surveyed technology leaders identified AI tech as a primary factor in the cost increase.
More than 80% of tech leaders anticipate that adopting generative AI features within SaaS products will further inflate software expenses next year, prompting many organizations to seek strategies to control cost creep.
To combat rising prices, businesses are leveraging their purchasing power, conducting more frequent audits and exploring open-source alternatives where feasible, Forrester noted.
However, managing these expenses is complicated by contract complexities and the challenge of predicting future needs.
Nearly half of organizations struggle with future-proofing their software plans, and many find it difficult to adjust existing contracts or escape vendor lock-in, even when they have a clear strategy in place.
“Forrester’s view is that as software increasingly offers AI, prices may increase disproportionately to the value received and won’t show a positive ROI everywhere,” the report said. “AI value in software will need to be carefully monitored and weighed as it evolves.”
Jim Liddle, chief innovation officer, data intelligence and AI at Nasuni, said to find a balance between innovation and budget, organizations must be willing to prioritize specific areas of the company and/or projects that will provide the greatest return and commit to investment in those areas.
“The challenges many organizations face is trying to spread the investment equally across the company and not target specific areas that will deliver the most benefit,” he said.
Liddle said when considering an AI project or investment, organizations need to first consider if the project is a use case that needs or can leverage AI, because not all AI use cases are necessarily going to generate hard ROI (i.e., cost saving or revenue increases).
“The key is for organizations to focus their efforts on soft ROI for initial AI projects, as this will steer organizations toward a sustainable, hard-ROI-oriented deployment of GenAI in the years ahead,” he said.
Daniel Avancini, chief data officer at Indicium, said he agreed IT leaders should proceed carefully and stop short of hiring new AI tools or paying for advanced AI features before understanding the underlying ROI.
“Even if there is real value to be made with AI, it won’t be the same for each company, and it pays to implement controlled experiments using classical A/B testing and other techniques to understand the value x cost balance of AI in general,” he said.
He noted navigating long-term contracts and vendor lock-in is a particular challenge for organizations, especially on productivity tools that are very hard to migrate and that often change terms in favor of more expensive plans.
“I wouldn’t jump into any long-term Gen AI contract before assessing its value internally and waiting some time for it to mature,” he said. “There are areas that companies can build their own tooling or agents and experiment with it, but that is not always the case.”
Liddle cautioned GenAI and other large language models (LLMs) can also fail if not provided with the right data access.
“Investing in solutions that centralize and organize all types of data files and formats first can ensure teams are prepared to effectively train, test, and deploy AI models,” he said.