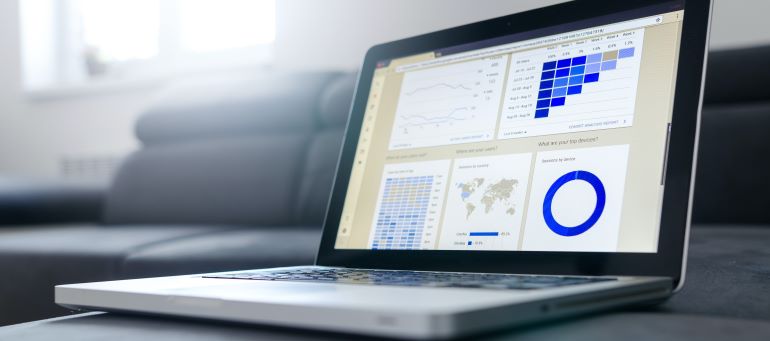
There is little doubt that AI is one of the most transformational technologies since the invention of the internet. PWC projects that by 2030, AI alone will provide a $15.7 trillion boost in GDP globally. Much like the internet, AI has evolved rapidly, and while there have been growing concerns about its societal and economic implications, it’s already being used for a variety of functions ranging from customer service and content development to recruitment and performance management.
This innovation has also led to worldwide expansion of AI safety watchdog organizations and the creation of new regulations such as the E.U.’s AI Act. In the U.S., a Senators’ legislative plan has proposed $32B in annual AI spending, pending future regulation, indicating promise of more to come in the U.S. This may leave organizations wondering: When is data a liability?
According to a recent study, all respondents who are adopting or plan to adopt GenAI have encountered challenges, with 49% in the U.S. claiming the quality of data is at the top of the list. This research supports that it’s nearly impossible for businesses to use AI to gain actionable insights from outdated data. Bottom line: Organizations must prioritize organizing and classifying data from its creation and initial storage to when it becomes obsolete and in need of permanent removal. This will not only help to limit their liability but also to derive the value they expect from AI.
Beware of the “Data Lake” Trap
First and foremost, AI is only as good as the data supplied, underscoring the importance of data classification and data lifecycle management. One trap many organizations fall into is accumulating a growing set of old or unnecessary data in a centralized repository or “data lake,” which, in addition to putting their business at risk of security breaches due to an expanded attack surface, also jeopardizes their AI efforts. Beyond the need to put systems in place to minimize the attack surface of these data lakes, there is also the need for real business value to be derived from this data for AI-driven projects. The algorithms and machine learning techniques that power AI require quality input in order to have quality output.
Throwing vast amounts of data into an AI model will only provide value if it is fed with recent and relevant data, not data from 10 years ago or regarding customers that have churned. In fact, feeding a large language model redundant, obsolete and trivial (ROT) data will diminish the return on that endeavor – garbage in, garbage out. Plus, the more data a Large Language Model (LLM) AI engine is fed, the more expensive it is to train. So, not only would this lead to bad results, it will cost more to generate. As organizations adopt AI to optimize their business processes and operations, they’re going to quickly realize that the only value they get out of training LLMs is by putting in actionable data. This is why effective data lifecycle management and data protection policies, including protocols on regular data sanitization, are critical for maximizing ROI from AI initiatives.
Making the Most of Your AI Investment
There are a few ways for organizations to improve the ROI of their AI efforts and optimize operational efficiency. These include:
-
Adoption and review of an effective data classification system – Building a strong foundation for data management begins with prioritizing data classification, or the process of categorizing data for the purpose of its storage, sorting and retrieval for future use. Essential steps for this process should include:
-
Labeling and defining sensitive and highly valued data.
-
Uncovering where the data lives and who has access to it.
-
Classifying data based on its value to the organization and assigning classification levels accordingly.
-
Implementing appropriate security controls and measures to ensure integrity, including sanitizing the data and certifying data removal.
-
A plan for regular monitoring and evaluation of security controls.
-
-
Following data lifecycle best practices, cleaning-up “data lakes” and eliminating ROT data – Old data can’t pose a risk if it isn’t there, so having a clear policy for managing how and what data is stored will save time and reduce costs down the road. This is where data management best practices come in, explicitly spelling out what data the organization has collected, including its value, where it’s stored and when it needs to be permanently erased. A recent study we conducted highlighted that just 55% of organizations can boast a mature data classification model that determines when data has reached end-of-life. This leaves room for improvement, with only half of respondents determining when to dispose of cloud-stored data. It’s also worth noting that 28% of respondents said they use the blunt approach of automatically setting a data expiration date, which is simple but ineffective. Relying solely on an expiration date ignores what kind of data it is, what it’s worth, or the risk of it getting into the wrong hands. Data sanitization protocols should include verification and a report in addition to a certified erasure algorithm.
-
Creating AI employee training programs and policies to guide the use of generative AI (GenAI) and foster a culture of innovation. One of the biggest security threats within an organization is often its employees that either haven’t received proper training or aren’t adhering to company policies. Employees can inadvertently be putting the organization at risk when using sensitive data in a GenAI tool like ChatGPT, which can now give third party access to this information. Ensuring that all team members within the organization understand the nuances of how data is stored can help mitigate the human element of risk. Organizations can make sure this training happens not only for new hires, but is refreshed for all employees on a regular basis to keep them apprised of company AI policies, as well as new techniques and tools.
-
Updating security policies to address and mitigate threats – Organizations at a minimum should be reviewing their data protection and security policies on an annual basis. That review should be looking at what policies they have in place today, which methods are being used to protect data and what are the erasure protocols in place. Unfortunately, those algorithms change more quickly than some organizations realize, as do sanitization standards. For instance, while the Department of Defense (DoD) 5220.22-M sanitization method has been common practice in the U.S., it’s a standard that was written before SSD technology existed, and has since been superseded by more modern standards from the National Institute for Standards and Technology (NIST). The annual review is an opportunity to look at the latest developments from NIST as well as the new IEEE 2883 standard.
There are many important reasons why organizations need to delve deeper into how their data is being managed, ranging from keeping up with compliance mandates to addressing continually evolving data security threats. However, in looking beyond legal obligations, a key business consideration is the impact of data clutter on AI initiatives.
In calculating AI’s Return on Investment (ROI), the value can only be reaped if the data quality is robust. Before making a significant investment in AI, organizations should take a close look at their data management and data classification systems.