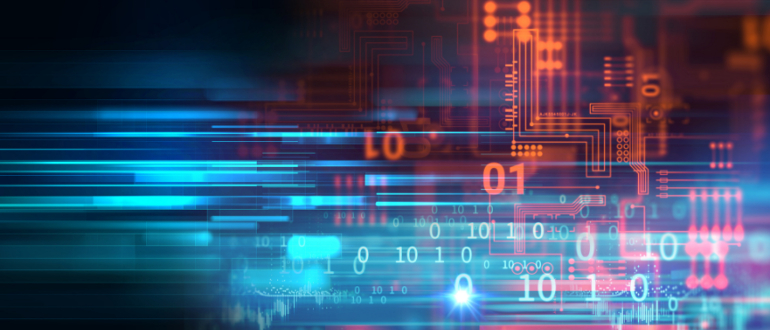
According to the 2024 AI & Information Management Report, 80% of organizations will expand their use of AI in the coming year. Still, Gartner has found that only 2% have reached the level of digital workplace maturity (which includes things like data governance) needed for successful AI implementation.
Without strong data governance, AI systems could expose an organization’s inherit data security risks by accidentally sharing critical information or producing unreliable results because data is out of date or irrelevant. All this leads to a high risk of poor decisions, inefficiencies for users and lack of trust in AI systems.
To achieve maximum efficiency, productivity and accuracy from AI tools, while also ensuring security and regulatory compliance, organizations need strong data governance strategies that leaders can follow:
Establish Clear Data Management Practices
AI models require vast amounts of high-quality, relevant data to perform effectively. Poor data quality can lead to inaccurate predictions and flawed insights, leading to decisions that may harm your business or customers. This is also why Gartner predicts that about 30% of generative AI (GenAI) projects will be abandoned after proof of concept by the end of 2025, with poor data quality as one of the reasons.
Good business decisions are made from good information — so before implementing AI, make sure you know what you’re working with. Start by conducting a discovery and analysis exercise to identify the ‘state’ of your content. This is to understand what data exists, how it is organized, as well as whether or not it’s in active use. Removing all the redundant, obsolete and trivial (ROT) data in the workspace is a crucial part of this process, ensuring data integrity when using AI, regardless of whether it is for processing large volumes of data or just using a basic GenAI prompt.
Moreover, having a data lifecycle management process – proper content management across the entire workspace container through regular data audits, consistent removal of ROT and implementation of automated lifecycle policies – is vital. By ensuring that data is appropriately categorized, retained and disposed of when no longer required for business or regulatory purposes, organizations can avoid holding onto too much or too little information, which is crucial for AI accuracy.
Implement Effective Data Access and Security Measures
In the era of AI, controlling access to sensitive data while ensuring that the right people have the information they need is necessary. Proper workspace governance helps organizations maintain security and comply with regulations, while continuing to ensure ongoing collaboration.
To ensure ethical and responsible use of AI, strengthening governance controls before using AI and GenAI applications is a must. In line with increasing excitement around AI, 29% of organizations plan to change how they manage data and run governance strategies. However, planning isn’t enough, implementation of strong policies is a must to ensure successful outcomes. Workspace admins can implement role-based access controls to manage who can access sensitive data, ensuring that data privacy policies are adhered to throughout the AI deployment process. They should also regularly audit access logs and permissions, ensuring policies remain in place and no unauthorized or external users can access workspaces. An automated approach can assist with this, ensuring that governance policies are applied consistently, reducing compliance risks and increasing operational efficiency without burdening knowledge workers.
By embedding these controls into the AI workflow, organizations can protect sensitive information and maintain compliance with regulatory requirements, thereby reducing risks associated with data breaches and misuse.
Foster a Culture of Data Accountability
For AI initiatives to succeed, organizations must cultivate a culture where everyone values, understands and uses data responsibly. Data governance is not just about policies and technologies; it’s also about fostering a culture of accountability within the organization. From top executives to frontline workers, everyone should understand the importance of data governance and their role in maintaining it. In fact, Gartner recommends the practice of “adaptive governance,” which suggests a fluid data governance strategy, allowing executives in the organization to distribute authority on how data and tools are governed.
Once clear data governance policies have been developed, organizations must cascade this information to all employees, ensuring they receive proper training on best data governance practices. When everyone in the organization is committed to data governance, it becomes easier to ensure data integrity and maximize the potential of AI technologies.
As organizations rush to embrace AI technologies, robust data governance ensures the accuracy and reliability of AI outputs and mitigates risks associated with data breaches and regulatory non-compliance. As we move further into the AI era, organizations that prioritize and invest in comprehensive data governance strategies will be better positioned to harness the full potential of AI, driving innovation, efficiency and competitive advantage in an increasingly data-driven world.