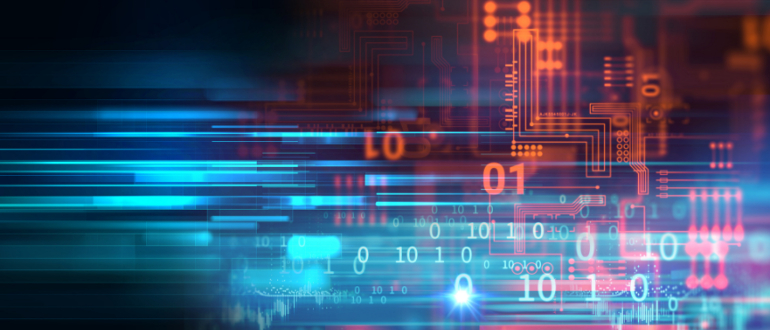
AI adoption has rapidly increased in the past year as it redefines and radically improves modern business operations across all verticals. Recent reports suggest that 35% of global companies already use AI within their organizations, while 50% plan to incorporate the technology in 2024. From sports strategies to health care, AI is at the forefront of innovation and problem-solving technologies today.Â
For instance, AI has helped the NFL improve player safety and assess risky plays, while chatbots built on LLMs help improve communication between doctors and patients. These advancements show how AI can surpass human potential and provide unparalleled opportunities in many fields.Â
However, AI is also the ultimate case of “garbage in, garbage out”. Without accurate and reliable data to work on, the technology can never reach its true potential. Â
Unlocking AI’s Power With Quality Data
Accurate and reliable data helps AI provide the best possible answers, while poor data quality can lead to distorted results. Gartner’s predictions reveal that poor data quality can cost companies $15 million annually. For the best results, Organizations must ensure that the quality of data consumed by their AI systems and applications is pristine.Â
AI systems enhance various organizational functions such as customer service through efficient chatbots and business process streamlining. These systems utilize identity data to accurately predict behaviors and assign access — a crucial component of preventing security breaches and ensuring compliance with regulations.
However, the decentralized nature of identity data presents its own set of challenges. It is often scattered across directories, databases and SaaS platforms throughout an organization. This fragmentation arises because different user groups such as employees, contractors and customers, have varying requirements, and identity data is stored in multiple locations, both on-premises and in the cloud. As a result, there is no single repository for all identity data.Â
When the input data is fragmented and outdated, it can lead to errors in AI processing. These errors, known as AI hallucinations, make decisions based on incorrect or biased data, and can lead to potentially severe financial and reputational consequences. Air Canada’s AI chatbot mistakenly advised a customer, leading to an unwarranted full-price ticket purchase under false refund expectations.
Implementing robust data observability is key to combating these issues. This involves ongoing data monitoring and analysis across an organization’s systems. Such oversight helps ensure the integrity and quality of data, identify anomalies and refine the management of data assets.Â
Decentralization makes data observability not just beneficial but essential; it enables comprehensive oversight across disparate data environments, ensuring that all identity data is accounted for and managed effectively.
Moreover, organizations should enforce stringent data hygiene practices. This involves more than just cleaning and organizing data. It requires setting up strict controls over how data is accessed and used. By adopting standardized and automated processes for managing data, businesses can reduce the risks linked to data mismanagement and create a strong foundation for their AI systems to function on accurate, reliable data.
Mastering Visibility in Identity Data
Gaining a clear view of how identity data flows through an organization’s information systems is essential. The transparency helps illustrate the paths and interactions of data, providing a critical foundation for effective AI operations. Achieving this often involves tackling the issue of data residing in separate silos, which obstruct seamless data analysis.
A practical solution is the adoption of a data fabric strategy. This strategy integrates different data sources into a unified, accessible framework, allowing the data to stay in its original system. Consider a crucial legacy system holding key identity data necessary for day-to-day operations. Through a data fabric, AI systems can access and utilize this data without altering the legacy system, thereby maintaining operational continuity and enhancing data utility for AI tasks.
Furthermore, the technique of virtualizing data from various sources is crucial in this context. It helps create a centralized view of identity data, effectively addressing data duplication issues — where the same data appears in different formats across multiple systems. This unified approach simplifies data management and boosts AI functions’ precision in tasks ranging from user personalization to security monitoring.
This strategy compels organizations to reevaluate their data collection, storage, and usage practices. Such a restructured data environment enables AI systems to make informed decisions based on a thorough and updated understanding of identity data, thereby driving more reliable and effective AI outcomes.
Enhancing AI Output With Automated Data Processes
After ensuring data visibility, organizations should standardize and automate identity data management. This standardization enhances the effectiveness of AI and machine learning applications by guaranteeing that data is consistently accessible, reliable and uniform. It drastically reduces the likelihood of errors during AI decision-making processes.
Adopting effective data management practices also significantly aids organizations in complying with global data protection regulations, such as GDPR in Europe or CCPA in California. High data hygiene standards ensure that personal information is handled carefully, safeguarding privacy and reducing the likelihood of incurring penalties.
Preparing identity data for AI involves data staging, cleansing and correlation. Staging organizes data into a structured format readily processed by AI systems. Cleansing removes any inaccuracies or irrelevant details, ensuring the remaining data is immaculate. Correlation then connects related data from various sources, giving AI a complete view of the information.
Automating these steps brings considerable benefits. It accelerates the data preparation process, which can be labor-intensive and minimizes human error, maintaining the data’s integrity and consistency. This uniformity is vital for managing access controls, where AI systems determine user permissions based on precise identity data.
To implement these processes effectively, organizations need to assess their current data management strategies, pinpoint areas for enhancement, and integrate technologies that support automation. This includes deploying an identity data management technology to seamlessly connect with existing infrastructures, automate policy enforcement and adapt to evolving data trends.Â
Future Proofing for Long-Term AI DevelopmentÂ
As data protection standards evolve, maintaining robust data management systems becomes crucial. Organizations with effective data visibility and hygiene protocols are well-prepared to adjust to new legal demands and sustain their compliance over the long term.
To summarize, effective data management is crucial for optimizing AI’s potential. Prioritizing data observability and hygiene enhances AI effectiveness and ensures compliance, protecting against security risks and regulatory challenges.