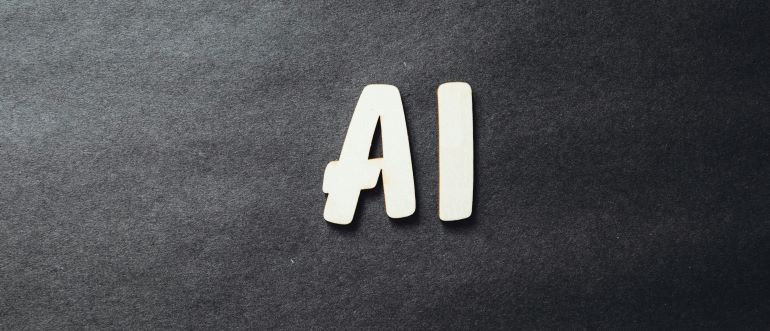
Companies leveraging AI-led processes are significantly outperforming their peers, with many reporting that investments in generative AI (GenAI) and automation are exceeding expectations.
Of the organizations canvassed in Accenture’s survey of 2,000 executives across 12 countries and 15 industries, nearly three quarters (74%) found their AI investments meeting or surpassing their anticipated benefits, and 63% plan to increase their efforts by 2026.
Companies that have fully embraced AI-led processes have nearly doubled in number, from 9% in 2023 to 16% in 2024.
According to the report these “reinvention-ready” firms achieve 2.5 times higher revenue growth, 2.4 times greater productivity, and 3.3 times more success in scaling generative AI use cases than their competitors.
Enabled by strong digital cores, these companies are applying AI across key business areas, including IT (75%), marketing (64%), customer service (59%), and finance (58%).
AI Integration Challenges Persist
Despite these advancements, many organizations still face challenges.
A majority (64%) are struggling to transform their operations, with 61% reporting that their data infrastructure is not yet ready for generative AI, and 70% finding it difficult to scale AI projects that rely on proprietary data.
Yaad Oren, managing director of SAP Labs U.S. and global head of SAP BTP innovation, said many organizations struggle to implement AI effectively due to three primary challenges: Poorly defined business problems, inadequate data quality and a lack of AI expertise.
“AI should be used with the purpose to solve business problems,” Oren said.
However, AI is not a one-size-fits-all solution, and businesses must clearly define their goals and implement the most relevant language models and data frameworks to achieve them.
One common issue is the tendency for companies to run too many AI projects simultaneously without proper planning.
“Proper prioritization and planning are keys to success,” Oren said. “AI initiatives should be strategically scaled rather than hastily executed.”
High-quality AI is only possible with high-quality data, but many organizations lack robust data governance frameworks.
Without clear data standards, metrics and reliable data, AI’s potential is severely limited.
“Many organizations lack the frameworks to enforce data standards and ensure data accuracy,” Oren noted.
Addressing the AI Talent Gap
He added the AI talent gap further complicates matters, with companies often struggling to find or cultivate the in-house expertise needed to manage AI systems effectively.
This shortage of skilled workers makes it difficult for organizations to identify appropriate AI use cases and integrate AI with existing infrastructure.
“The shortage of skilled workers makes it difficult to fully leverage AI capabilities,” Oren said.
From his perspective, addressing both data quality and the talent gap is essential for businesses looking to unlock AI’s full potential.
Rajeshwari Ganesan, distinguished technologist for Infosys, said a strong digital core and comprehensive data strategy are crucial for successful AI integration, providing the foundation for effective implementation and continuous improvement.
“A robust data strategy ensures data quality, accessibility and governance, enabling the creation of reliable AI models and insights,” she said.
The digital core supports seamless integration of AI technologies, facilitates continuous model evaluation and retraining and enables effective lifecycle management of AI models.
Together, they allow organizations to maintain model relevance, overcome integration challenges and adapt to changing business needs.
“This approach is essential for maximizing the long-term value of AI investments and maintaining a competitive edge in dynamic markets,” Ganesan said.
Ramping Up AI Readiness
Ganesan said to better identify and address gaps in AI readiness and avoid project failures, companies can adopt a bimodal approach consisting of an AI Foundry for rapid experimentation and innovation, and an “AI Factory” for scaling and engineering.
“This strategy allows organizations to explore cutting-edge AI technologies and develop novel use cases while ensuring that only thoroughly vetted solutions make it into production,” she said.
Implementing an AI Center of Excellence (CoE) to set the overall AI vision, develop best practices and provide guidance, along with an “AI Control Tower” for real-time visibility and decision-making, can further enhance AI readiness.