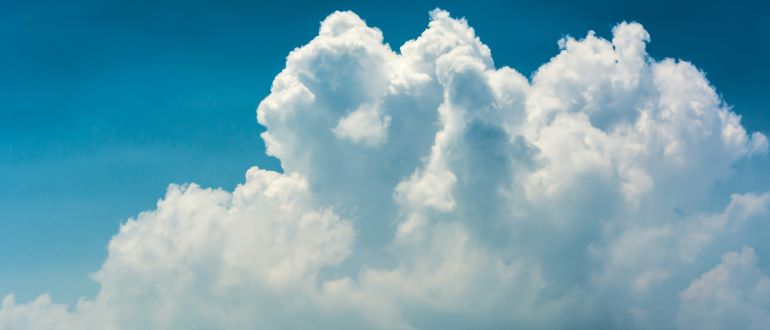
With its potential to revolutionize data analysis, amplify the capabilities of the workforce and automate intricate processes, AI has exploded over the past year. But this surge in adoption of AI tools and training models is creating unprecedented demands and new requirements for computing resources. At the same time, the global nature of digital business and differentiation in the cloud market have created a realization that companies, especially large enterprises, will be operating in a multi-cloud environment regardless of whether they want to or not — and they need new ways to manage the complexity of provisioning connectivity, deploying apps and managing connectivity across multiple clouds.
As these forces build momentum, a new paradigm for multi-cloud networking is emerging to streamline management of disparate IT infrastructures.
The Challenges of Building a Network for AI
While most people are familiar with large language models like ChatGPT and Bard that can generate outputs across a spectrum of human and machine languages, we are just beginning to see the proliferation of more specialized AI applications that employ those capabilities. Pharmaceutical companies may use AI trained on internal research data for quality control. A telecommunications company may deploy chatbots across multiple regions and languages.
Whatever AI is used for, the challenge of provisioning connectivity, maintaining consistent security, and managing application services across different environments and requirements persists.
AI is resource intensive, requiring more GPU processing from infrastructure-as-a-service providers like AWS and Microsoft Azure. Chasing GPU capacity means companies are more dependent on multiple hyperscalers to provide those resources.
There are also latency and bandwidth requirements for the AI user experience that can only be met through localized deployments. To provision retail stores or edge devices with new AI applications, the processing power should ideally be nearby.
At the same time, AI inferencing relies on training data located in a centralized data lake. An AI application still needs to learn and draw from its centralized data source and continually maintain that connectivity. AI apps must be deployed near customers to minimize latency, and this often requires different cloud regions to ensure performance while also ensuring that training data is accessible to large language models at all times.
The Burden of Complexity
For NetOps, DevOps and SecOps teams, AI is another workload that must be managed across multiple locations and systems. But an AI application may have thousands of deployments and microservices in containerized environments that are combining to create one customer facing app — meaning there are a lot of endpoints, nodes and connections that must be maintained to truly have a consistent security posture and user experience.
Maintaining a highly complex application that draws resources from different cloud silos can become a huge time sink that also creates risk in terms of ensuring correct and consistent configurations in different tools. Traditional implementation of a complex environment like this requires long integration cycles across vendors — each with their own set of configuration interfaces and settings — and that complexity increases with the number of deployment locations.
Once an AI inference application is in production, NetOps, DevOps and SecOps teams need to ensure that the user experience is protected across all of those network elements, and that they can scale up or down as required. Inconsistent models to move services also means more integration as the services evolve.
As all this complexity grows, it leads to cumbersome and disjointed operations with discontinuous visibility. And this creates friction, balloons costs and exposes the organization to risk.
A Unified Approach to Management, Security and Visibility
All of this is driving the need for a true multi-cloud networking platform that can abstract multiple cloud management processes into a single interface to deploy and manage highly distributed applications without slowing down for costly integration and deployment cycles. Whereas traditional provisioning and setup can take several weeks to connect multiple locations to their data sources, with a centralized management tool, the entire process can happen seamlessly through a single pane of glass.
With this approach, an application can be deployed anywhere in the world in minutes, instead of the weeks it might take to go through multiple provisioning and configuration processes. Connectivity to data sources in any cloud is seamless, and caching of data can easily be routed closer to the actual AI models.
A uniform management plane across clouds can also facilitate sophisticated policy controls with centralized visibility and analytics. Instead of having to manage through individual cloud portals, which can require different teams with different skillsets, a single view can provide visibility through the entire application stack. Third-party security tools like firewalls, bot defense and distributed DDoS attack defense can also be deployed and managed centrally to work alongside the management and connectivity plane.
The end results are that customers are able to access and use AI applications without worrying about their data being compromised, and they can have a consistent experience regardless of where they interact with the app.
With a unified multi-cloud networking approach, instead of scaling up brand new teams to handle exponential complexity in the age of AI, your NetOps, DevOps and SecOps teams can focus on delivering new experiences and adding value to the business. There is transformative potential harbored in the convergence of AI and multi-cloud networking to carve pathways toward a smarter, more efficient and highly adaptive future.