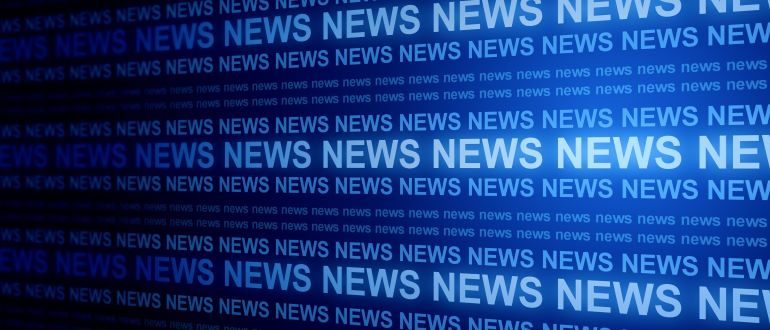
BMC Software this week at its Connect 2024 conference previewed a pair of tools that promise to make it simpler to ensure high-quality data is only used to train artificial intelligence (AI) models.
Control-M Data Assurance, available in beta, defines dozens of data quality metrics that can be used to inspect and evaluate their data in a way that enables organizations to adopt and maintain best DataOps practices that enable organizations to manage data in a more agile fashion.
At the same time, BMC previewed Metadata Navigator with Gen AI, a tool that makes it simpler to discover relevant data by leveraging large language models (LLMs) to provide descriptions of data based on the metadata that has been exposed.
Company CTO Ram Chakravarti said it becomes apparent that AI models are only as good as the quality of the data used to train them. Organizations are realizing they need to revisit the way data is managed in the age of AI as they look to aggregate data of varying quality that is distributed across an organization in a variety of unstructured, semi-structured and structured formats, he added.
Much of the structured data is well managed but, in many cases, the unstructured data needed to train AI models resides everywhere from on individual PCs to any number of cloud services, noted Chakravarti. Rather than hoarding all that data in a data lake, BMC is working toward making it simpler to identify the data that is most relevant to the business, he added.
That’s critical because IT teams need to be able to easily find and then aggregate the minimum amount of data required to manage the data needed to train AI models that are trained to automate workflows in specific domains, Chakravarti noted. In fact, the size of those AI models will be substantially smaller than the general-purpose AI models that have been trained by, for example, OpenAI, while at the same time generating output that is more reliable, he said.
The challenges associated with unlocking value from data have, in the last few months, diminished the halo effect AI has enjoyed, as organizations realize the level of investment required to operationalize AI, said Chakravarti. DataOps presents an opportunity to rein in the costs in a way that ensures that only high-quality AI models are deployed in production environments.
Overall, organizations need to have a more deliberate strategy for operationalizing AI, noted Chakravarti. Rather than taking and pursuing Big Bang projects, organizations need to plan for the long term. Additionally, they need to keep track of the pace of AI innovation, because by the time they might build and deploy their own AI model, those same capabilities might become widely available in an IT platform provided by a vendor.
Regardless of approach, the success of any AI initiative, as always, depends on the quality of the data used to train the models that drive it. The challenge is not only discovering that data should be used to train an AI model, but also ensuring that it securely arrives wherever an AI model is being trained.