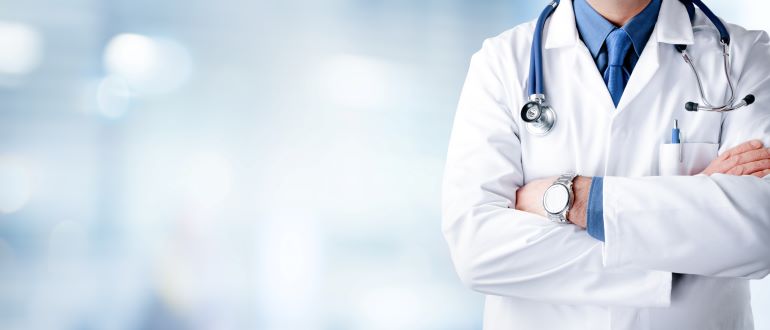
The explosion of artificial intelligence (AI) is transforming the health care landscape, and widespread adoption is only expected to increase. From streamlining administrative tasks to optimizing patient engagement, the development of new AI tools is on the rise for a variety of reasons. However, as adoption continues to increase, it’s up to the leaders of health care organizations to develop thoughtful and responsible AI-based products.
While working in AI sounds exciting, a lot must go on in the kitchen before the final dish is cooked to perfection. Here are some critical lessons I’ve learned during my journey leading AI initiatives and through my research in this area that may be valuable for other health care technology business leaders:
Define a Clear Purpose and Vision
While it may sound cliché, it has never been more important to have clarity of purpose when launching an AI product. The potential impact of AI is profound, but the technology comes with its own risks, including its tendency to amplify biases and provide inaccurate information in the form of “hallucinations.” Unless we as leaders set a high purpose and standard for quality and accuracy, we run the risk of developing an ineffective product. And while this may be a learning moment in other fields, it is particularly risky in an industry such as health care where these technologies may have a direct impact on patient lives.
AI is an entire world in itself. As we brainstorm the type of products we can create and the vast number of potential use cases, we could easily have hundreds of innovative ideas. The wide array of opportunities makes it even more critical to set concrete parameters for evaluating use cases and ensuring your team is focusing on top-priority projects.
Prioritize Hiring the Right Talent
To boost efficiency and maximize operations whenever possible, it is critical to establish the right team backed by strong expertise. The key is to ensure your team has the necessary skills needed for building your relevant product.
Creating the dream team, however, goes beyond just functional skills. Maintaining the right internal attitude across your team is just as integral to a project’s success. In the case of AI, an emphasis on resilience, adaptability and agility is key. Generative AI-based development is still in its infancy, and we have only just scratched the surface. During this critical experimental phase, organizations must be prepared to switch strategies as needed. For example, a team could be working for months in a certain direction, either gathering data or training an AI model believing that it would lead to the right outcome, and then may end up having to completely abort the current process when it doesn’t yield the relevant results. Considering the fast-paced and dynamic nature of the health tech industry, team members should remain nimble and not lose hope when the initial shine of AI is gone and the rubber meets the road.
Focus on Product Vision Before Tech Enthusiasm
Many AI projects begin from the tech Proof of Concept (POC)— an internal experiment designed to test the feasibility of an AI product for its intended use. When the POC is solid, the project team then considers how to effectively productize it. However, jumping straight into this process may not have the intended value for your organization, or your customers.
As my colleague and AI product director Qing Xia has explained, “Starting with tech POC can create issues, as a cool new technology may not fit into the workflows of customers and deliver value. It should be the other way around: Determine product vision and optimal user workflow, and then design the corresponding AI POC to prove feasibility.”
Reframe How You Approach Agility
The agile methodology of software development first caught fire in 2001, quickly becoming popular and replacing the more traditional waterfall development methodology to accelerate development. More recently, the new “agile” became the old “waterfall,” with many companies planning several product releases throughout the year with minimum flexibility.
Given AI is still in the early experimentation phase, organizations may no longer be able to follow this process. Instead, developers should prioritize one to two product releases with flexibility built-in to keep pace with the current market.
Engineering Your Prompt and Leveraging the Right Data is Key
Abraham Lincoln has been quoted as saying “If I had eight hours to cut a tree, I will spend seven hours sharpening my axe.” Effectively engineering your prompt — the process of designing inputs for your AI tool that will produce optimal outputs — is crucial for getting accurate and relevant responses. Going back to Point 1 on setting your purpose: It is very important to define what your accuracy standards are and help the team deliver to those standards.
The key to accuracy is to avoid ambiguity and provide context and perfect examples. Using large amounts of data during initial prompt engineering can be costly. Instead, start by fine-tuning your prompt with smaller data sets, then gradually increase the volume to cover all use cases. Developers should also make sure to reserve a sufficient portion of fresh data for testing and validation that hasn’t been used for training the model.
Always Center Around the Customer
Although this may be an age-old saying, no AI project can be successful without first recognizing that you are addressing a genuine problem and that your customers are willing to pay for a solution.
Engaging customers throughout the entire development process — from conception to commercialization — is key to creating value. Leveraging relevant customer feedback during the pilot and beta phases before launch is one simple way your team can help build your product to the customer’s requirements.
Strive for Continuous Knowledge
AI is a new field for everyone. No question is dumb. As a business leader myself with limited technology or coding experience, it was initially challenging to understand the backend of how this works. For example, when do you use NLP vs LLM? To remain competitive and enable success, developers and leaders should keep a pulse on the latest happenings within the market and learn how to adapt and utilize the latest technology accordingly.
By following these best practices, health care tech organizations can innovate responsibly, with the end-user in mind. Organizations that prioritize thoughtful, responsible development and implementation will not only create impactful AI-based tools, but also have the opportunity to set new standards for how our healthcare providers operate and deliver quality care.
The views expressed in this article are the personal opinion of the author and do not necessarily reflect the views or positions of the author’s employer, Modernizing Medicine, Inc.