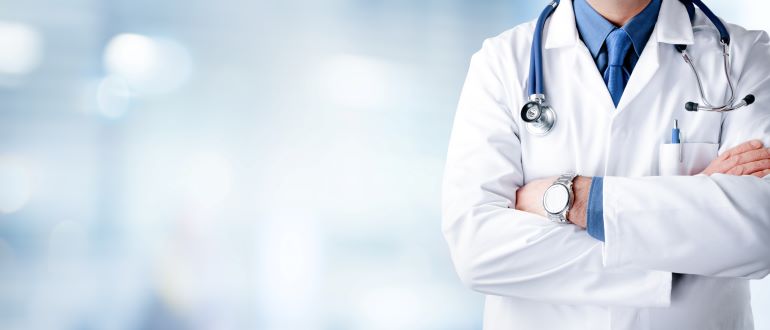
The health care market has been inundated with new AI solutions, and to be sure, some of these solutions apply AI meaningfully and effectively; however, there are just as many solutions that promise to revolutionize care delivery and administration. In reality, many of these solutions amount to little more than using AI-powered chatbots to marginally reduce manual workflows and overhead, while charging customers exorbitant sums for the service.
The trend of AI companies moving into health care and health care companies moving into AI – fueled by large tech investments – is clear evidence of AI’s massive potential. Yet even the most well-established AI companies have struggled to adapt their solutions to the complexities of health care. ChatGPT is widely regarded as the most advanced generative AI available to the public, yet when researchers for JAMA Pediatrics recently put ChatGPT to the test, the program incorrectly diagnosed an astonishing 83% of pediatric cases.
These low-ROI applications make it harder for legitimate AI solutions from established health care AI organizations to gain a foothold in the market. And this pattern of overpromising and underdelivering will inevitably engender skepticism among health care leaders, which in turn will hamper adoption of AI-enabled solutions across the industry.
To effectively utilize AI in health care, health care leaders first need to understand what AI is capable of and, just as important, what it isn’t. A more complete understanding of AI is essential to help the health care industry separate truth from hype. Provider organizations in particular need to be very careful in how they apply AI, especially in regard to patient care. But there is a sweet spot for AI in the health care continuum: To address the overwhelming administrative burden many organizations are wrangling with. AI has tremendous potential to help with administrative simplification, risk adjustment and value-based care.
Much of the buzz surrounding health care AI is centered on the potential of generative models. Boston Consulting Group claims that generative AI can read and analyze MRIs, diagnose conditions, create personalized treatment plans for patients, improve health care data interoperability, and even support population health initiatives.
The potential for these applications certainly exists, but there are some significant hurdles to be cleared before the health care industry can meaningfully apply generative AI with little to no human input. Generative AI is expected to make mistakes as it learns; these mistakes are, in fact, how the AI learns. Other industries can afford to take a trial-and-error approach, but that isn’t the case in health care, where the stakes are much higher, and failure can be fatal. Care providers have a legal and moral duty to protect the lives of their patients, and there is simply no room for error.
Despite these challenges, the imperative to adopt AI in health care is clear. The industry’s move towards value-based care requires massive amounts of patient/member and population data to be effective. The more data health care organizations collect, the more administrative work is needed to manage it, and the more the industry spends on administrative tasks. A recent report from the Council for Affordable Quality Healthcare (CAQH) found that the health care industry spent $60 billion on administrative tasks in 2022, an $18 billion increase from 2021.
Most patient data is unstructured (images, chart notes, non-OCR PDFs and faxes, etc.) and is not easily organized or pulled into health care systems for further analysis. As a result, valuable insights about patients/members and populations often remain inaccessible to health care organizations. Administrative overhead can significantly be reduced by applying AI to help make human workers more efficient. Generative AI can deliver contextual data to provide details and assistance to dramatically decrease administrative tasks.
Health care organizations also operate across disparate systems, which exacerbates the challenge of data sharing. The insights providers and health plans exchange with one another are often fragmented and incomplete, which in turn creates friction between partner organizations. Overcoming these obstacles is vital for success in value-based care.
Effectively harnessing and applying data-derived intelligence is one major hurdle for the health care industry. Another is the need to ensure the fidelity and accuracy of that data. AI models are only as accurate as the data they are trained on; health care organizations must prioritize data fidelity to optimize the accuracy of AI-driven insights.
The road to meaningful AI adoption in health care is a long one. Nevertheless, the health care industry’s data problem underscores the urgent need for AI support. By prioritizing data accuracy and interoperability, stakeholders can begin to unlock the potential of AI in health care. As it always has, the industry will continue to evolve.
Reshaping the future of health care delivery and administration will require a responsible and judicious application of AI solutions. And while AI can augment the work humans do, it is important to remember that AI alone is not an acceptable substitute for human interaction and clinical evaluation.