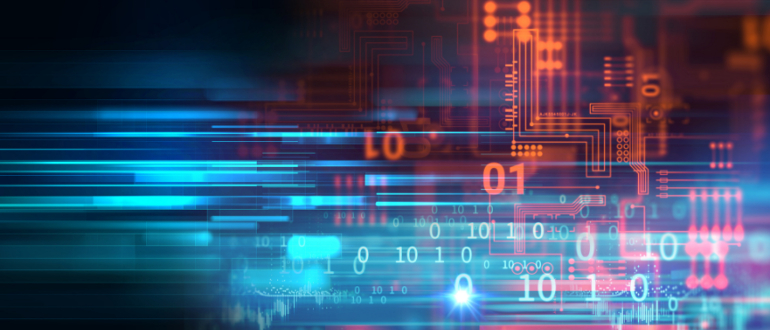
When it comes to business, generative artificial intelligence (GenAI) is more than the “new shiny,” it’s a competitive edge and a path to the future. It will create new written, visual and audio content in a snap. It can digest and summarize mounds of complex data, discovering valuable trends that would have been missed. It can generate code, handle repetitive tasks in the back office and out front and drive new levels of customer service.
A joint study by professors at America’s top business schools found highly skilled employees who use GenAI can experience as much as a 40% boost in performance over those who don’t. Not surprisingly, analyst projections are also promising as Gartner estimates by 2026, more than 80% of enterprises will be using GenAI APIs or AI-enabled applications. Bloomberg Intelligence expects the GenAI market to explode, growing from a market size of just $40 billion in 2022 to an astounding $1.3 trillion by 2032.
Yet, despite the rosy assessments, many businesses will find their GenAI journeys a difficult one, if they are even able to complete it at all.
Haste Makes Waste
Somewhere between 60-80% of AI projects are failing according to a majority of analysts and experts that follow the space. Many companies charged into the realm with all the haste of a miner running late to a gold rush. From unclear internal objectives to complex project management processes, a lot can go wrong when working with this nascent technology, and as a result, a lot of investments have been wasted.
Foremost, the success of a GenAI project relies on understanding the right use cases for your business and the quality of the data used. While it is not as time-consuming as traditional machine learning AI models cleaning data using complicated data processing pipelines, the old adage “garbage in, garbage out” still applies. Time should be invested in ensuring data sources are accurate, otherwise, unreliable outputs will render it useless. Further, the technology has proven so dynamic – and its uses so new and fast in coming – a knowledge gap has arisen. In attempting to overcome this, many common challenges have been identified.
The following looks at the top ones and how to sidestep any pitfalls.
Keep it Legal
What you can and cannot legally do with GenAI is still fuzzy as regulations are being debated to control its use. In March, lawmakers in the European Parliament approved a restrictive law called the AI Act. This will aim to put a halt to the most dangerous forms of AI, like public facial recognition, while mandating companies get approval for less harmful uses. The Act will more than likely go into effect this May. Meanwhile, U.S. President Joe Biden has instructed federal agencies to start creating AI regulations, but the executive order does not carry the force of the law.
This has created a lot of legal ambiguity about GenAI. Some companies feel uneasy spending tons of budget developing AI technology that could be throttled back by regulations or even outlawed after being released into the wild. Know where your AI dream stands, legally, before it becomes a nightmare.
Compute Adds Up
AI workloads consume far more resources than traditional ones, including compute power, bandwidth and storage. That’s because AI models must be trained on large datasets and that can get expensive. To counter this, companies enlist the cloud for AI and its better pricing, scalability, flexibility and easy access.
Still, it is easy for a business to lose control of cloud consumption and rack up costs. Intelligent technology can offer insights and tools to handle complex cloud challenges, effectively manage critical processes and control and optimize cloud spend. Such platforms support FinOps and can enhance your AI’s overall business value, which is vital for getting and keeping buy-in from higher-ups.
Data Quality and Explainability
Back to the aforementioned phrase “garbage in, garbage out.” Nothing could ring truer when it comes to data quality and AI. Bad data is the number one issue with these projects. It is not just that outputs could end up being wildly wrong. There can sometimes be a black box element to GenAI, and if results cannot be explained or you are unable to identify the source of data to affirm its legitimacy, you have a big problem.
Since the big data boom, organizations have been working to straighten out their data sets so they can build even more ambitious projects on top of them. This is paying dividends for those companies as the above-average data quality puts them in a stronger position to quickly apply GenAI and capitalize on opportunities.
Close the Gap
In a survey of U.S. executives by KPMG, less than half felt their company had the tech, talent and management capabilities for AI success. Further, they felt a minimum of six to 12 months was needed just to understand, evaluate and develop an AI plan. Companies with mature processes and skilled pros in place can execute fast and put distance between competitors. This could turn into an insurmountable gulf for those new to the game, especially when talent with the right skills is hard to find and going to the highest bidder.
You have to close that knowledge gap. So, when considering a cloud management and optimization platform, see if the provider has the architects, knowledge transfer and resources to help determine your AI readiness. And be sure they can identify company-specific use cases and will work collaboratively with you on customized solutions.
Hope and a Way
There is hope and a way to supplement your own capabilities. Hyperscalers like Amazon Web Services and Google are creating GenAI-focused programs, and vetted partners are available for assistance. Use these resources to create AI strategy, clarify specific business objectives, gather and refine data, guide feature development and more. Build the foundation for your own GenAI innovation and the results will come.