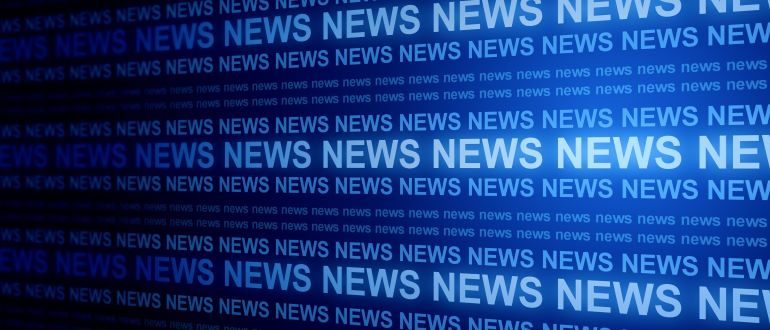
Datadog today made generally available an ability to observe large language models (LLMs) that IT teams can use to monitor, for example, latency, token usage, exposure of sensitive data and toxicity.
Announced at its Dash 2024 conference, LLM Observability also makes it possible via a single dashboard to monitor the entire LLM toolchain to, for example, identify the root cause of a hallucination that is generating incorrect outputs.
In addition, LLM Observability can also be used to safeguard applications against prompt hacking using the Datadog Sensitive Data Scanner.
Finally, it enables IT teams to visualize the quality and effectiveness of LLM conversations to surface early indications of potential drift, regardless of whether the LLM is originally provided by OpenAI, Anthropic, Azure OpenAI, Amazon Bedrock or Vertex AI.
The LLM Observability service will initially most likely be used by data engineers that are tasked with managing and governing LLMs, says Yrieix Garnier, vice president of product for Datadog. However, in time LLMs will become part of the IT fabric that is managed by DevOps and IT service management teams as usage of LLMs in production environments increases, he adds. “It’s still very early,” says Garnier.
The one thing that is certain is those teams will be increasingly augmented by artificial intelligence (AI) agents that will be optimized to perform a range of tasks, including optimizing usage of large, medium, small and domain-specific language models. After all, many applications only require access to a small subset of data compared to the data that has been used to train an LLM, notes Garnier.
Less clear is to what degree AI might drive a wave of consolidation across the various silos that make up an IT organization today. In the meantime, however, the level of scrutiny being applied to how LLMs are operationalized is only going to increase in the months ahead. Everyone from auditors to shareholders will want to know how organizations are safely operationalizing generative AI in a way that is cost effective.
That latter issue is especially challenging given the current cost of invoking an LLM at scale. Organizations over time are likely to switch out language models to reduce those costs by, for example, opting to embed an open source language model in an application rather than making external application programming interface (API) calls to an external service. Each organization will need to continuously monitor those prices versus value metrics as usage of various AI models grows, notes Garnier.
Regardless of the path forward, observability will be essential. The issue then becomes, once that level of visibility is achieved, determining to what degree to automate management processes versus relying on a set of manual processes still managed by some type of IT professional. In theory at least, AI models should be able to manage and govern other AI models. However, each organization will need to determine how much faith to place on AI models that because of the way they are trained might tend to drift beyond the scope of their original mission.