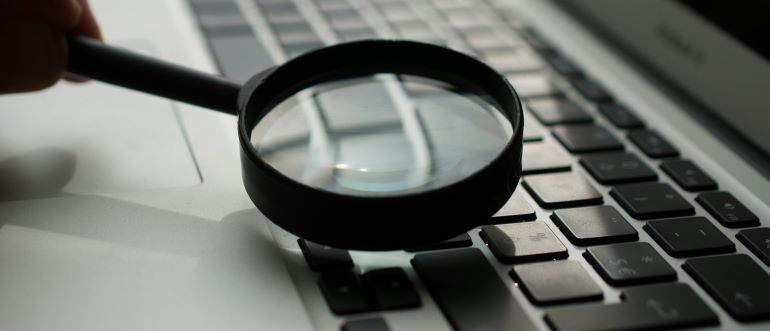
The pace of innovation in generative AI remains swift. Major tech giants like Microsoft, Amazon and Meta have significantly increased their investments in this category.
Despite this, LLMs (large language models) continue to have major issues. Just a few include hallucinations, bias, toxic content, latency, security and copyright infringement. Then there are the problems with the unpredictability of LLMs when it comes to generating responses.
It’s true that there are ways to help mitigate the issues, such as fine-tuning models and RAG (retrieval augmented generations). But they are far from foolproof.
This is why observability has become critical for successful deployment of generative AI applications.
“At the end of the day, ITOps teams need to be certain that their current systems are able to handle GenAI tools,” said Karthik SJ, who is the GM of AI at LogicMonitor. “With observability technology, you’ll be able to be more competitive, efficient and scale faster. Without it, best of luck.”
Explainability
A challenge with observability is that many of the top LLMs are closed source and only accessible via APIs. That is, there is little transparency or explainability for the responses.
Even open source models can be difficult to evaluate. After all, they can be massive and complex. There may not even be complete transparency. An LLM developer, for example, may not provide access to the training data.
“This is why you need to think more along the lines of input and output mappings,” said Kristof Horompoly, who is the VP of AI Risk Management at ValidMind. “It should give some idea of how the model is either being consistent, or its performance is improving or deteriorating. There also needs to be some sort of a benchmark to check model performance, and one way to do that is to create a set of golden prompts for the use case.”
Toolsets
Observability tools allow for collecting large amounts of useful data from cloud and on-prem LLMs, such as with logs, metrics and traces. There are many effective proprietary and open source systems available. Many are general-purpose solutions, but there are also those that are built primarily for generative AI applications.
“AI observability enables organizations to quickly respond to shifts in data patterns, model drift, or environmental changes,” said Ashan Willy, who is the CEO at New Relic. “This agility is crucial for maintaining the effectiveness of AI applications over time.”
In terms of generative AI, it’s crucial that an observability tool allows for real-time intervention and moderation. This could include libraries of guardrails, such as to detect potentially harmful content and hallucinations, prompt injections and use of personally identifiable information (PII). There should be an easy way to customize the system for policies and notifications.
Best Practices
While generative AI is in the nascent stages and is dynamic, there are some best practices for observability that are emerging. For example, observability should not be done as an afterthought. It should be a part of the process of reviewing new generative AI projects. “This should include identifying participants and understanding the data being used to ensure that each project demonstrates ROI and handles sensitive data appropriately,” said Venky Veeraraghavan, who is the Chief Product Officer at DataRobot.
Next, it’s important to build a feedback loop in the generative AI applications. For example, this is what ChatGPT does with its thumbs-up/thumbs-down icons for responses. This can provide invaluable data to refine the application.
Finally, there will likely be more of a need for having humans-in-the-loop.
“Unlike AI, humans can grasp the full nuance and context behind AI-generated outputs, allowing them to detect subtle issues and anomalies that automated systems might overlook,” said Guru Sethupathy, who is the CEO and co-founder at FairNow. “Their expertise ensures that AI operates within its intended guardrails, promptly identifying and resolving problems that could otherwise escalate. Keeping humans in the loop is essential for building trust in AI systems.”