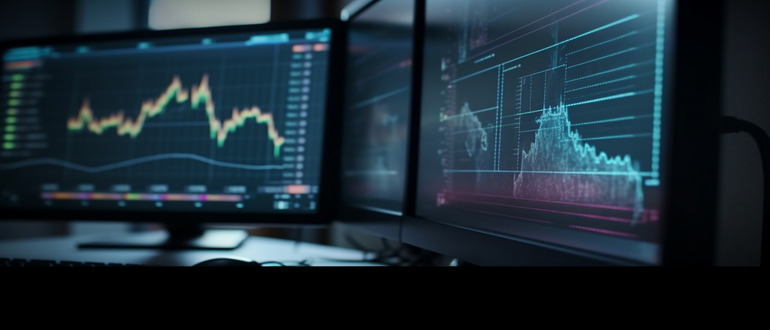
Vultr this week announced it is making available its first instances of AMD Instinct MI325X graphical processing units (GPUs) in its Chicago data center region using AS -8126GS-TNMR 8-U servers from Supermicro.
Given the cost and scarcity of GPUs, many organizations building and deploying artificial intelligence (AI) applications are anxious to gain access to GPUs from AMD to provide an alternative to NVIDIA. Vultr, which also offers NVIDIA GPUs, plans to eventually make AMD GPUs available across its global network, says Kevin Cochrane, chief marketing officer for Vultr.
It’s not clear how many organizations building and deploying AI applications will opt for AMD GPUs, but the company has a strategic advantage over rival providers of processors, notes Cochrane. Many organizations now have enterprise agreements for consuming cloud services based on AMD CPUs, which might be extended to include GPUs, he adds.
At the same time, many of the decisions concerning which class of processor should be used to deploy the inference engine that AI models invoke in production environments is shifting toward a centralized IT team that has often embraced platform engineering as methodology for building and deploying applications at scale, says Cochrane.
Additionally, the major hyperscalers are trying to entice IT organizations to rely more on the processors they have developed, he adds. That may provide some cost advantages but organizations are generally more wary today of becoming locked into a specific cloud computing platform, Cochrane highlights.
As such, many of them still tend to favor NVIDIA, AMD and Intel processors that can be invoked across multiple cloud computing environments. Those three trends collectively are reshaping how IT infrastructure in the cloud era is being managed, says Cochrane. “It’s really a perfect storm,” he adds.
It’s not clear to what degree GPU scarcity has been hampering the building of AI applications, but much of the scarcity can be traced back to chip manufacturing issues. The current level of demand for GPUs that are used in everything from gaming rigs and automobiles to HPC platforms and cloud services far outstrips the available supply. How long that situation may persist is unknown, but as other suppliers of GPUs enter the market, the cost of GPUs should eventually fall.
In the meantime, many organizations, depending on how much access to GPUs they have, are likely to find they will need to prioritize a handful of AI projects at the expense of others. As a result, it may be well into the next year before there are enough GPU infrastructure resources available to pervasively deploy AI applications.
Regardless of approach, the one thing that is clear is IT teams are getting savvier about how to rightsize AI models to the class of processors they might be best suited to run on, Cochrane says. The challenge, as always, is finding a way to monitor AI applications to ensure that whatever level of performance initially required will be achieved as the AI models driving them continue to grow and expand.