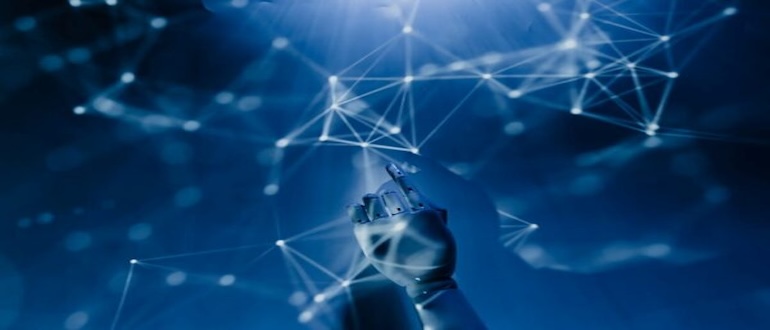
Leaseweb Global this week expanded the range of graphical processing units (GPUs) available across its cloud services to include additional options from NVIDIA that don’t require organizations to make lengthy contract commitments.
The L4, L40S and H100 NVL GPUs from NVIDIA being added are rapidly becoming mainstays for organizations that are both training AI models and deploying AI inference engines, says Eli Lahr, a senior solutions engineer for Leaseweb.
The overall goal is to provide IT organizations with another option for hosting AI workloads at a time when GPUs from NVIDIA are still relatively scarce, he adds.
Additionally, those offerings will provide a more flexible alternative to larger hyperscalers that typically require organizations to make GPU consumption commitments for a full year. Many organizations want to be able to consume GPUs based on per hour or even a per second basis, he notes.
In general, organizations are still experimenting with AI applications, so making those kinds of consumption commitments simply isn’t practical, Lahr says.
Finally, not every AI workload is created equal. A small language model (SLM) isn’t going to require the same level of GPU resources as a large language model (LLM), notes Lahr. “Not everything needs the full power of the latest GPUs,” he adds.
The degree to which the scarcity of GPUs is holding back deployment of AI applications is difficult to assess but there is little doubt that many organizations are considering their options. At the same time, more organizations are closely monitoring advances in AI training that suggests the total cost of building and deploying AI applications may be about to rapidly decline.
Additionally, virtual GPUs will play a larger role in helping organizations reduce the total cost of AI, Lahr says.
Larger organizations often have enterprise license agreements with one or more hyperscalers that require them to consume a specific amount of infrastructure within a given 12-month time period to ensure discounts are applied. The degree to which those agreements can be applied to consumption of GPU resources will vary from one cloud service provider to the next. Smaller companies, however, will require more flexible options that might be offered at lower cost per instance.
The one thing that is clear is AI requires a significant amount of infrastructure expertise to rein in costs. Unfortunately, data scientists and application developers often have a tendency toward consuming cloud resources without fully appreciating the costs that might be incurred. Theoretically, as more organizations embrace best FinOps practices to optimize their cloud costs, many of those concepts will be applied specifically to AI – but in the short-term, adoption of FinOps remains uneven.
Fundamentally, it’s still a matter of economics as demand for GPU capacity generated not just by AI applications but also electronic vehicles and gaming systems far exceeds the available supply which is constrained by the current amount of manufacturing capacity available in Taiwan. In fact, one concern is to what degree geopolitical tensions might result in reduced access to the raw materials needed to manufacture GPUs.
In theory, it’s probable that all these concerns are temporary as other manufacturing options are explored but given how fragile the GPU ecosystem is, organizations would be well advised to hope for the best while planning for the worst.