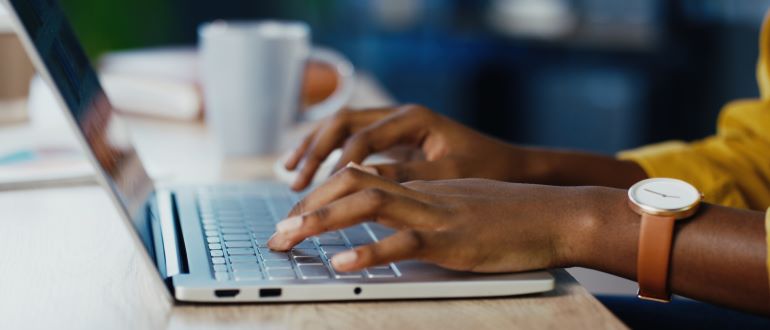
Vultr has added support for the AMD Instinct MI300X accelerator along with associated ROCm programming models, tools, compilers, libraries and runtimes for building applications to its cloud computing service.
Kevin Cochrane, vice president of marketing for Vultr, said the graphical processor units (GPUs) from AMD now provide organizations with a viable alternative to GPUs from NVIDIA that are in short supply.
That’s critical, because AI and other high-performance computing (HPC) initiatives are being held up, simply because organizations don’t have enough access to GPU infrastructure, he added.
Vultr already offers a wide range of infrastructure services, including access to Kubernetes clusters that have become a de-facto standard for running AI workloads. “We’re known for being cloud-native specialists,” says Cochrane.
Each organization will need to determine what degree they will require GPUs versus traditional CPUs to run AI workloads, noted Cochrane. For example, AI workloads that only need to process workload in a batch mode might be more cost-effectively handled by CPUs, rather than GPUs that are better suited for meeting real-time application performance requirements, he notes.
Additionally, GPUs, depending on the use case, may serve up more accurate outputs based on their ability to process data in parallel.
Cloud service providers will, of course, play a crucial role in enabling AMD to compete against NVIDIA.
AMD GPUs can match the price/performance capabilities of NVIDIA, but the company still needs to further develop the ecosystem needed to foster development of AI applications that take full advantage of its GPUs, says Daniel Newman, CEO of The Futurum Group. “They need more with their partners,” he says.
Like other cloud service providers, Vultr provides organizations with access to GPUs from NVIDIA and its rivals. Many organizations will invariably wind up consuming a mix of a class of processors depending on their requirements and preferences.
It’s not clear to what degree GPU scarcity has been hampering the building of AI applications, but much of the scarcity can be traced back to chip manufacturing issues. The current level of demand for GPUs that are used in everything from gaming rigs and automobiles to HPC platforms and cloud services far outstrips the available supply. How long that situation may persist is unknown, but as other suppliers of GPUs enter the market, the cost of GPUs should eventually fall.
In the meantime, many organizations, depending on how much access to GPUs they have, are likely to find they will need to prioritize a handful of AI projects at the expense of others. As a result, it may be well into 2025 before there is enough GPU infrastructure resources available to pervasively deploy AI applications. Alternatively, the next generation of CPUs might also be able to efficiently run some subset of the inference engines that organizations need to deploy AI models, especially as smaller more domain-specific AI models become available.
In fact, a recent Vultr survey of 1,000 senior technology executives with artificial intelligence (AI) expertise that are employed by mid to large enterprises finds that, on average, organizations have already deployed 150 various types of AI models in production environments.
Regardless of the infrastructure employed, however, it’s no longer a question of whether to build and deploy AI models as much as it is how many and how soon.