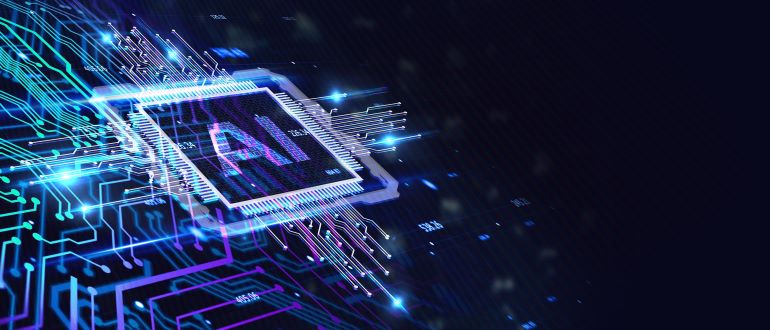
The rise of GenAI and its breakthrough benefits turned heads around the world. However, concerns about bias and the absence of explainable AI (XAI) remain. AI risks increase exponentially if those developing AI don’t master how to build and train bias-free models, and companies cannot explain to consumers how these models make decisions that impact their daily lives. If the tech industry cannot master this, how can consumers trust our AI-driven decision-making processes?
Luckily, all hope is not lost. AI informed by reputable sources and properly designed can be unbiased and even outperform humans in some arenas of decision-making, resulting in more equitable (and innovative) global industries.
Illuminating the Black Box
There have been instances of AI models spewing unhinged remarks, and Big Tech shrugged when prompted for answers. Why does this happen? Many powerful GenAI tools are trained on a massive corpus of data from the deep depths of the internet. If your brain went to questionable Reddit threads, you’re onto something. While this training data allows these models to understand colloquial language, it also welcomes bigotry.
Explainability is integral to promoting transparency and trust in AI systems. AI engineers can create explainable AI by limiting the possible responses an AI can provide by providing pre-approved answers based on reputable sources. They must also be diligent in modeling good behavior when training AI and identifying and removing foul play in the data set. That way, leaders can assure consumers that their AIs’ decision-making processes are top-tier, which is crucial when a model informs life-changing decisions.
An obvious question is: Does restricting responses also restrict the full potential of the AI model? Thankfully, the answer is a resounding no for those chasing unbiased and explainable yet robust AI models. Limiting responses does not limit the rewards. When AI is explainable, companies can enjoy a lot of critical business benefits:
- Less risk: Take, for example, highly regulated industries like finance, health care, and aviation. AI cannot be a black box for these industries– explainability is crucial to privacy and safety.
- Increased revenue: McKinsey reports that companies are more likely to grow their annual revenue by 10% or more when implementing XAI. Similarly, IBM found that users of its XAI platform saw their model accuracy improve by 15-30% while seeing $4.1-$15.65 million more in profit.
- More trust and adoption: McKinsey also reports that explainability is vital to building trust – and therefore increased adoption – of AI.
Retrieving the Right Results
As mentioned, one key to an unbiased AI is restricting inputs to limit the number of potential answers. Most enterprise applications deploy AI models to solve specific problems, so restricting them is logical and necessary. Here’s where developers must decide between retrieval-based AI and GenAI models. Retrieval-based AI proves to be the most beneficial in most instances. The main difference between retrieval-based AI solutions and GenAI solutions is that retrieval-based models pull only from a pre-developed, pre-approved set of responses, while generative AI creates net new responses.
Using the retrieval method, AI developers can ensure their pre-approved responses are not influenced in unwanted ways. In doing so, an AI reviewing patient symptoms and providing medical advice provides helpful input based on a list of pre-approved reputable medical journals, rather than giving false advice or sharing biased advice scraped from a 1950s textbook found in a random internet archive. This is not a case where you want something new; instead, you want something proven.
Modeling Good Behavior
Restricting an AI model’s output is essential to creating a robust and unbiased AI model. However, some other crucial measures should also be considered, such as modeling good behavior. The step may resonate with parents who know that teaching the next generation requires a lot of practicing what they preach by abiding by household rules and routines and reinforcing expectations with positive language. They are right on the money to draw this comparison.
For application engineers developing a chatbot, modeling good behavior might mean compiling hours of past interactions that their organization deems as the highest standard of customer experience and ensuring that the AI observes and learns from these examples. That way, the bot an engineer is building for an airline or insurance company learns from the call recordings that ended with a happy customer rather than the customer service agent losing their cool.
Eradicating Bias in AI
Avoiding and eradicating bias in an AI model calls for two critical ingredients: Leaving your own bias at the door and recognizing and correcting bias in an algorithm’s training data set.
These two steps are, again, a bit like bringing up small children. Children are sponges, taking in the world around them and spewing out everything they hear (often to their parents’ dismay). If a child curses, you start biting your tongue. You correct the behavior and track down the TV shows, movies, and music your kid is exposed to that are supplying these unwanted words and cut off access. Similarly, an AI is only as good as the data it is trained on, the sources that fuel it, and the human overseeing this training. Therefore, as an AI engineer, you must approach AI training equitably, and as a tech leader, it’s your duty to vet and assess prospective hires who build AI, especially if you’re serving critical industries like finance, real estate, healthcare etc.
If you’re as certain as possible that the human involved in AI training is unprejudiced, recognizing the bias in your model and correcting it is your next paramount step to fine-tuning your model. Once you understand the biases present in your AI models, adapt them and make them unbiased by changing the training data that you fed into it or measuring the outputs of standardized sets of questions.
Forging the Future of AI for Good
It’s easier said than done: Complex models present in modern-day applications make it difficult to trace decision-making processes, and the opacity of many AI models can lead to biased outcomes. But companies must provide illumination of what’s going on in the “black box” that often surrounds their AI’s inner workings and carefully address the bias that may be present in these models. Now more than ever, the tech industry must change our approach to AI model development to eliminate issues before they arise. Those who do will enjoy endless advantages and forge a new standard for ethical yet cutting-edge artificial intelligence that makes the world fairer.