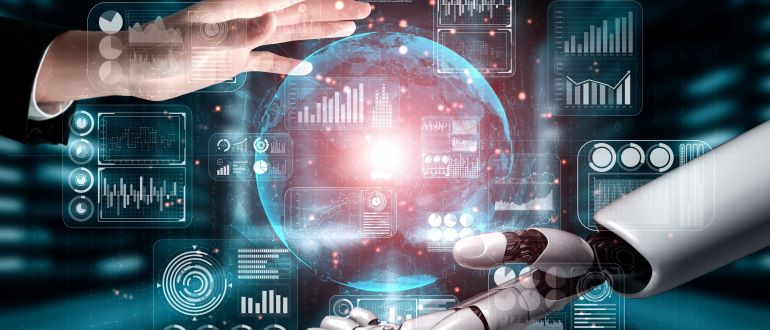
In a digital and global marketplace, businesses of every size will need to enter the generative artificial intelligence (GenAI) race to remain relevant or risk losing their customers to more tech-savvy competitors. GenAI and large language model (LLM) tools allow businesses to reinvent their products, customer experience, support, operations and software.
Still, there is significant concern about how GenAI will impact the workforce, possibly eliminating roles within organizations. For example, a recent American Staffing Association report found that nearly three-quarters of approximately 2,000 American adults (74 percent) said they fear that ChatGPT and other generative AI tools will result in higher rates of underemployment by taking jobs away from humans, as these technologies continue to take on more prominent roles in the workplace. “Workers are trying to figure out what the rise of artificial intelligence means for their careers,” said Richard Wahlquist, CEO of the American Staffing Association. “Employers must take responsibility for helping their workforce navigate and evolve to meet rapid changes in the economy through training and upskilling. Further, it’s imperative that organizations communicate with employees as AI technology is deployed to set expectations and provide transparency.”
Cybersecurity, DevOps, and other technology leaders can not only play a key role in integrating GenAI and LLM tools within their organizations but also can educate the workforce on the many benefits of the technology. Instead of considering what AI could potentially take away, it’s more important to look at the value it can provide in the work environment.
Always Evolving, Always Expanding
The GenAI and LLM market is rapidly expanding. A recent Bloomberg Intelligence report projected that the GenAI market could grow to $1.3 trillion by 2032. “The world is poised to see an explosion of growth in the GenAI sector over the next 10 years that promises to fundamentally change the way the technology sector operates,” said Mandeep Singh, senior technology analyst at Bloomberg Intelligence and the report’s lead author. “The technology is set to become an increasingly essential part of IT spending, ad spending, and cybersecurity as it develops.”
The impact these technologies ultimately have, however, will depend on how the technology continues to evolve. The market’s scope is certainly increasing, with LLM models such as GPT-3, DALL-E, Stable Diffusion, LLaMa, and Claude becoming more sophisticated at generating synthetic media—images, video, audio and text, for instance. Such developments have unleashed the potential to develop new applications across industries. Meanwhile, the quickly improving capabilities of LLM models such as GPT-3 allow for more natural language processing and generation, with common use cases including content creation, conversational AI for chatbots and code generation. While concerns around bias, misinformation and legal issues remain, GenAI and LLMs can potentially transform other areas, such as design, content creation, customer service, entertainment, healthcare and education. Overall, GenAI and LLMs are gaining capabilities that can change how media, content and data are produced and consumed across various segments and parts of the economy while these tools’ full scope and potential are still unfolding.
These and other emerging technologies are progressing at a dizzying pace. What is cutting-edge today may be obsolete in six months. This reality underscores the importance of building, experimenting and failing fast with GenAI. The only way to keep up is through continuous experimentation. Failing fast provides learning opportunities from previous experiments and allows for finding valuable use cases early. Much of the progress with AI comes through trial and error, and testing different approaches exposes what GenAI can and can’t do well, at least at a given moment. This process helps an organization determine where to focus efforts for business impact. While the aforementioned questions still surround GenAI, safe and controlled experimentation in low-stakes environments helps uncover potential issues early. Ultimately, the accelerated pace of progress and uncharted nature of GenAI make this experimental mindset critical. Low-risk cycles of building, testing, and failing foster faster innovation, better products, and informed adoption.
Finding the Right Implementation Approach
It is important for organizations to adhere to best practices as they develop an effective GenAI and large language model strategy. This starts with conducting research and closely tracking the latest developments in GenAI to understand emerging capabilities, limitations, and use cases. Study what leaders are doing and establish a research and development unit focused on GenAI.
When crafting a GenAI implementation approach, start small. Identify a few high-potential pilot areas to deploy GenAI that solve specific problems versus broad initiatives and expect to go through multiple iterations of prototypes and pilots to refine generative AI applications. Establish an AI Center of Excellence, create tiger teams that can quickly experiment to determine the feasibility of a product or solution by assessing pros, cons, and risks; focus on quick wins; and train technical teams on GenAI best practices. Institute guidelines for usage, ethics, security, privacy, and compliance tailored to the organization and use cases.
GenAI is designed to alter work or, more accurately, to augment the work of humans. As such, technology leaders need to be prepared for shifts in workflows, roles, and cultures; manage organizational change and develop runbooks where applicable. As GenAI continues to become part of the workflow, regularly assess performance metrics and realign strategies as the technology and the organization’s needs evolve.
GenAI and LLMs are still developing and gaining capabilities to change how media, content and data are produced and consumed across different segments and diverse parts of the economy. The key to successfully integrating GenAI and LLM technologies ultimately lies in balancing structure with agility and learning, thoughtfully building GenAI expertise and applications while allowing room for experimentation and iteration as the technology matures.
About the Author:
Arundeep Nagaraj is a leader of solutions architects and developer experience engineering. With more than a decade of experience working with global teams, customers and developers, Arundeep has a track record of success leading cross-functional teams, managing complex projects, and delivering products that exceed expectations. Connect with Arundeep on LinkedIn and Twitter.
The opinions expressed in this article are those of the author. They do not purport to reflect the opinions or views of his employer.