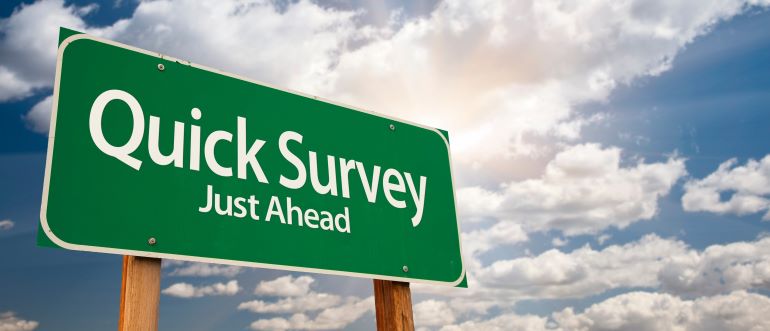
A survey of 1,026 U.S. business and technology executives published today by PwC finds more than half (55%) work for organizations that in the last 12 months have invested to some degree in artificial intelligence (AI), with more than half (54%) reporting their company has currently implemented generative AI.
Overall, 89% said they plan to increase their IT budgets over the next 12 months, with 58% also planning to prioritize investments in AI, the survey finds.
However, only 7% of respondents said their company consistently gets value from their emerging technology and GenAI investments.
The survey makes clear that business leaders appreciate the potential AI has to transform business workflows, says Emmanuelle Rivet, US TMT and global technology leader at PwC. Less clear is to what degree they are motivated by a general fear of the being left behind, versus truly appreciating all the potential risks, she notes. “There’s a lot of promise and peril,” she says.
In general, half of respondents said they strongly agree that their company’s emerging tech strategy is integrated with the company’s business strategy, with more than a quarter (28%) of all respondents having at least 20% of their employees involved in emerging technologies as part of their primary job function.
According to the survey, the primary eight emerging technologies identified as having the most impact are AI, Internet of Things (IoT), blockchain, augmented reality, virtual reality, advanced robotics, quantum computing and neuromorphic computing.
The one thing that is certain is that in terms of interest, generative AI is separating itself from other classes of emerging technologies. Arguably, no other emerging technology in recent memory has seen a similar spike in the number the experimentation projects that have been launched. There are, of course, already plenty of uses cases involving predictive AI, but expectations for productivity gains enabled by generative AI are considerably higher.
The challenge given some of the well-known limitations of generative AI is where best to apply it today. Platforms such as ChatGPT that were trained using massive amounts of conflicting data collected from across the Web are prone to hallucinations. There are plenty of instances where ChatGPT can be used to craft better emails and generate summarizations but any process that requires high levels of accuracy may not lend itself as well to a general-purpose platform without, at the very least, finding ways to extend the capabilities of the underlying large language model (LLM) used to build ChatGPT.
Conversely, organizations may decide to customize a different foundational LLM that, for their use case, may prove to be more accurate. Some may even decide to build their own LLM as the cost of training an LLM eventually declines.
The biggest issue, however, may simply be finding and retaining the talent required to build, deploy and maintain AI applications. It typically requires a small army of data scientists and engineers working alongside DevOps and cybersecurity professionals to safely build and deploy applications based on AI models.
Regardless of the approach, however, the AI genie is clearly now out of the bottle. The issue is now determining how best to apply AI, given the limited resources at hand, in a way that returns the most value to the business.