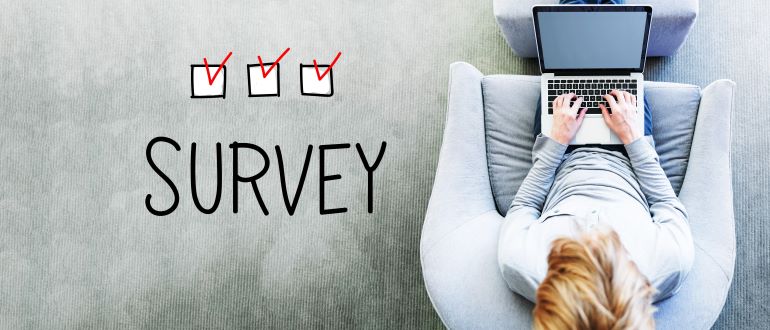
While many organizations are enthusiastically investing in generative artificial intelligence (AI), a global survey conducted by Lucidworks suggests many of them are leaping before looking.
While 93% of respondents said their organization will increase investments in generative AI in the next 12 months, the global survey of 6,000 employees involved in best practices finds that on average only 7.5 of the 80 known best practices for employing generative AI have been adopted.
Roughly 96% of executives and managers involved in AI decision processes from technology or data science departments are actively prioritizing generative AI investments, the survey also finds. Global business leaders are predominantly directing those toward improved customer experience, automation and efficiency, and overall business operations.
Companies in the entertainment, technology and consumer products industries are frontrunners in plans to increase generative AI spending, followed closely by construction/real estate, financial services and transportation.
Technology and entertainment/media companies are the most advanced practitioners of generative AI, with 21% being classified by Lucidworks as “AI Leaders,” double every other industry.
The professional services, government, hospitality and tourism sectors are lagging in their adoption of generative AI, each with fewer than 6 out of 80 best practices deployed.
The survey notes that organizations that do not plan to increase investments in generative AI have quadruple the number of concerns involving security, accuracy, transparency and job displacement than those who do plan to invest. In general, most organizations are plowing ahead despite the many concerns they have, noted Chris Cottle, VP of marketing for Lucidworks. “For every one thing they are excited about, there are three to four issues they are worried about,” he adds.
It’s still early days as far as adoption of generative AI is concerned but many organizations are trying to determine to what degree they can extend existing large language models (LLM) versus possibly building their own. On the one hand, there are already more than 200 LLMs that organizations can extend using a vector database that enables them to compare new data to the results provided by an LLM that is trained on older data, also known as “grounding.” Alternatively, it’s becoming easier to build LLMs on a narrow base of data that generates more accurate results than a general-purpose LLM such as ChatGPT.
In the short term, however, many organizations are finding it easier to build the code and platforms needed to extend LLMs versus hiring a data science team to build one.
The next challenge will then be determining how best to make generative AI accessible alongside existing tools such as search engines, notes Cottle. In many cases, organizations are starting to use generative AI to provide a natural language interface (NLI) to respond to queries alongside a search engine capable of surfacing related product information from a catalog, says Cottle.
Regardless of the approach, the survey makes it clear generative AI will be pervasively adopted in a way that will cause a sea of changes to occur across multiple industries, he adds.
The challenge now is where best to apply generative AI to not only remain competitive but also hopefully gain a strategic advantage.