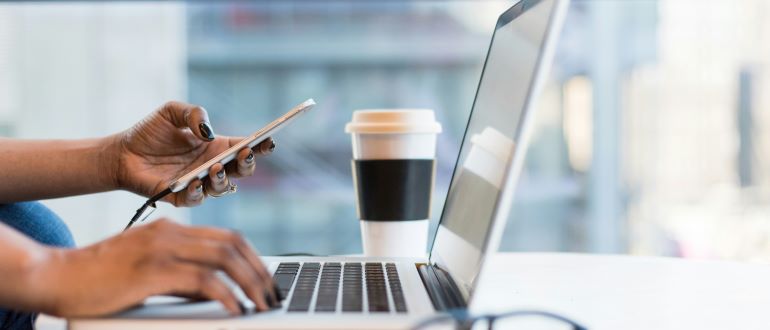
Generative Artificial Intelligence (GenAI) is likely the most impactful new technology we’ve seen in decades — and user adoption and market growth statistics support this. ChatGPT was the fastest-growing consumer app of all time with 100 million users in two months, and GenAI’s market is expected to reach $1.3 trillion by 2032, according to Bloomberg Intelligence. Applause’s recent GenAI survey, conducted in March 2024, revealed that over a third of respondents already use a GenAI application on a daily basis.
GenAI is an exciting technology, but it also comes with risks. Examples of bias, toxicity and inaccurate responses have been widely reported by the media. Companies cannot assume that existing testing processes for mobile and web are enough to account for these new challenges. Given the rate of change in the market, companies need to both plan ahead and make sure their testing plan meets the complex needs of GenAI applications.
New Use Cases… and Risks
The arrival of GenAI marks a transition away from traditional AI, which is often used in predictions, to using AI to create new content entirely. We are moving to a co-pilot era in which humans are leveraging GenAI to boost productivity. Some key use cases in this area driving market growth include:
- Summarization: Processing unstructured natural language data to create summaries
- Search: Conversational search ability allows users to refine results
- Text-to-text: Assisting with content creation from blog posts to poems
- Text-to-image: Applications that create images and videos based on text
- Text-to-code: Assisting in the writing of new code for software development
Generative AI can amplify risk already in applications, while also introducing new risks. These include:
- Inaccurate or inconsistent responses
- Biased, harmful, toxic responses
- Misuse of GenAI applications to create misinformation, deep fakes, etc.
- Regulatory compliance issues for data and personal information in different geographies, which remain in flux and evolving
- Legal and security risks such as copyright infringement, breaches of privacy and missing attribution
To mitigate these risks, organizations developing GenAI applications should involve humans in testing. Further strategies are discussed below.
Testing GenAI: Best Practices
While the process of building and deploying GenAI applications remains quite new, industry best practices are already emerging that can both help mitigate the aforementioned risks while also ensuring quality. Some of the top best practices include:
- Altering application testing and feedback processes: Unlike the QA and testing process for other applications like mobile and web, GenAI applications that leverage large language models (LLMs) require a different approach. Organizations need to design a testing program specific to LLMs that encompasses detecting and mitigating inaccurate responses and addresses harmful content, privacy and copyright issues.
- Creating an experienced and diverse testing team: When it comes to testing your GenAI application, diversity is important. This is because content that may not be considered biased by one group may actually offend or even harm another group. A testing team should represent different ages, genders, races, ethnicities and abilities to increase the likelihood of different forms of bias being recognized.
- Testing Different Aspects of the Application: Testing a GenAI application should include a focus on three main areas: User interaction, identifying functional bugs, and collecting feedback related to user experience. Several different testing methods to help cover these bases include:
- Natural Usage. This approach asks testers to use an application just as they would in the real world.
- Adversarial Testing. Asks testers to try and generate biased or inaccurate content.
- Directed Prompt Testing. A specific set of guidelines for testers to use different prompts to stress test a GenAI application.
- Feedback Collection: Different levels of feedback can be given by a tester for any given application. For GenAI applications, feedback should be collected related to:
- An application’s response: Was it accurate, toxic, biased, etc.?
- Tester/User Experience: Satisfaction, trust, issues, limitations with using the application.
- Feedback over time: Tracking GenAI application performance over time to see how it may change with new features or data added in, etc.
- Testing for Accessibility and Inclusivity: For all digital applications, GenAI or not, they should meet accessibility standards. Testing for accessibility and inclusivity creates a better overall user experience for everyone, not just people with disabilities.
The Rise of Red Teaming
Red teaming is commonly used in cybersecurity to identify vulnerabilities. The process involves a team of experts executing tests to see how well defenses hold up and where weaknesses may lie.
In GenAI, red teaming can be used to identify responses that are inaccurate, biased, unsafe or inaccurate. In a systematic adversarial approach, human testers identify issues that developers can use to retrain models and develop rules to mitigate the issues from reoccurring.
Testing for the Future
While the full potential of GenAI applications has yet to materialize, the best way to ensure these applications meet that potential faster is to test them properly.
By testing applications in areas related to accuracy, accessibility, privacy, etc., organizations can mitigate the risks and concerns associated with GenAI and accelerate the rate at which it can bring positive and useful impact to businesses and consumers alike.