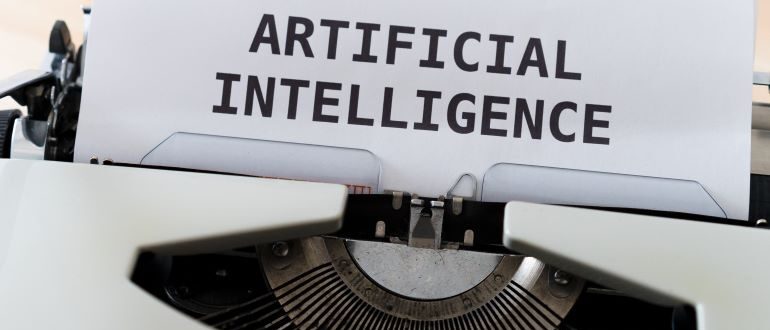
Artificial intelligence has taken the world by storm, revolutionizing several industries with its promise of greater efficiency. However, critics have pointed out several concerns with AI technology, not the least of which is its potential to create biased results. Thus, many leaders in the artificial intelligence industry are shifting their focus to ensuring the fairness and inclusivity of algorithms and models.
Understanding the Source of AI Bias
One important thing to note about artificial intelligence is that it is, as of now, entirely dependent on the data it is fed during its training process. Currently, AI cannot synthesize “novel” data — it can merely synthesize conclusions based on pre-existing data — but this has led to some complaints about AI’s tendency to offer inaccurate, discriminatory, or otherwise inappropriate responses.
Artificial intelligence, therefore, has an inherent bias brought about by the data from which it is sourced. For AI models used for important use cases, such as recruiting bots that sort through applicant resumes or even legal AI models used to help judges make sentencing decisions, this inadvertent perpetuation of biases in data can create unfair, harmful outcomes for marginalized groups.
Another challenge the artificial intelligence industry faces is a lack of algorithmic transparency, as many users do not understand how the models they use come to their decisions. This ambiguity is due to a combination of factors, including intellectual property concerns and the complexity of the underlying technology. Many of these algorithms are so complex that the average user cannot understand them, and developers are worried that those who do understand them will attempt to steal and replicate their technology.
However, developers can increase the transparency and interpretability of their models and output without compromising the integrity of their IP rights. Developers need not reveal aspects of the underlying technology that could be replicated, but they should disclose what data the model pulls from, what user data the model collects, and the justification for any decisions the model issues. This would allow users to understand what process was used to inform the model’s decision and adjust accordingly if necessary.
Fighting Back Against AI Bias
One way in which artificial intelligence leaders are striving to combat the potential bias of their models is through bias detection programs, though it is essential to note that these bias detection measures have shortcomings of their own. Many of these programs use an algorithm to detect outputs that may be considered “biased,” but these algorithms also have shortcomings. In setting the parameters for “bias,” those developing and training the model introduce their own biases.
Perhaps the most extensive way AI developers can avoid biased output in their models is through ongoing monitoring. In most cases, it is not a matter of if an artificial intelligence will exhibit bias, but when.
Thus, a system of checks and balances is necessary to prevent these biased outputs from having a disproportionate impact. Chiefly, an AI model should not be used to make essential decisions, but rather should issue a suggestion and supporting evidence the human user can then use to inform their decision.
Still, once the models reach a certain user base, it is difficult for the developers to continuously monitor the output of the AI for potential bias. In these cases, users become an invaluable resource.
AI developers would be wise to incorporate a robust feedback system, allowing users to report when they notice or experience biased responses. The developer team can then take these reports and investigate what happened to enable the system to exhibit these biases.
Proactively Addressing AI Bias
That being said, the best way to prevent biased output by artificial intelligence models is to take a proactive approach and attempt to avoid bias in the first place. Although it is impossible to eliminate bias entirely, there are ways to minimize it.
For one, the development team should be as diverse as possible, with developers representing different backgrounds, ensuring they bring a wide variety of perspectives to the table. This helps prevent the model from replicating homogenous views and perspectives.
The data a model uses as its source should also be diverse and rich in representation. If a model only pulls its data from a single source, its output will automatically and consistently reflect the biases and inaccuracies of said data. However, by sourcing its data from multiple points, a model can cross-reference information to better represent accuracy and quality decision-making. While it won’t be perfect, there will be less likelihood of an egregious error. There is a broader concern around the ethical use of data which potentially also impacts bias in data, so data itself should be ethically sourced with full regard to the rights of the owners.
There is no denying artificial intelligence’s potential as a revolutionary technological innovation, but developers and users must be responsible about how they train their models to prevent the inherent biases from having unfair outcomes. While it may not be possible to completely eliminate AI bias, steps can be taken to identify and mitigate these biased responses when they arise.