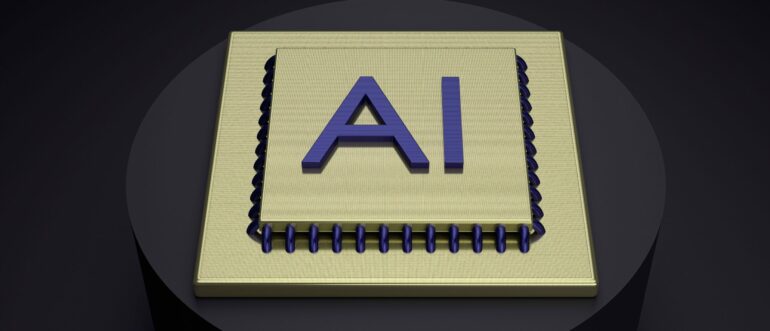
As businesses embrace generative AI (GenAI) technologies, they face heightened risks of accruing technical debt, particularly those moving quickly to adopt platforms and large language models (LLMs).
These were among the findings of a Gartner study, which suggested that by 2028, more than half of enterprises venturing into custom large language model development may halt their initiatives due to the substantial costs, complexities and technical debt incurred.
The report noted enterprises starting from scratch bear acute technical debt risks, underscoring the importance of flexibility in their GenAI strategies.
As teams leverage current AI models to develop applications, chief information officers (CIOs) are advised to prioritize architectures conducive to swift API updates, ensuring adaptability to emerging technologies.
Caroline Carruthers, CEO of data consultancy firm Carruthers and Jackson, said it’s important to be clear about the purpose of building and maintaining LLMs in-house – if this is unclear new projects will often end up abandoned.
“Costs, complexity and other factors can all be managed as long as the purpose is clear,” she says.
She explains it’s essential for businesses to look at their intentions and break them down into the smallest possible components before diving in.
“For instance, when learning to cook, begin with the basics, not a gourmet dinner; start small and build from there,” Carruthers advises.
The Gartner report cautioned that with the landscape of AI constantly evolving and new techniques and models emerging regularly to enhance cost-efficiency and accuracy, organizations must remain vigilant.
Even those leveraging vendor solutions face significant technical debt potential, necessitating a proactive approach to maintain agility.
The study also revealed that despite the risks associated with early adoption, generative AI initiatives offer potential solutions to mitigate technical debt.
Gartner forecast that by 2027, enterprises will harness generative AI tools to craft suitable replacements for legacy applications, significantly reducing modernization costs by up to 70%.
Carruthers says the steps organizations can take to begin adopting GenAI tools with purpose involves defining what the business is going to do and what it will not, which is an equally crucial aspect.
“Secondly, there’s performance, which entails measuring relevant metrics,” she says. “Then there’s perception, which involves assessing your agility and flexibility in your approach.”
She adds that perhaps most importantly, in preparing to adopt GenAI tools, there’s perseverance as it’s crucial to maintain an experimental mindset and keep pushing forward.
Dragan Gajic, CTO of Nortal, says he agrees companies need to accept this is an emerging technology and focus on the usage and adoption of LLMs.
“They are also called foundational models because you can build on top of them rather than build your own,” he says.
He explains operating LLMs in-house requires the development of new capabilities like LLMOps, as well as specialized hardware needs.
In the era of SaaS and cloud services, companies need to carefully assess the reasons to operate LLMs in-house due to the high total cost of ownership (TCO).
“We also advise adopting an LLM-agnostic approach and ensuring there is no vendor lock-in, even in the case of an in-house developed LLM,” Gajic says. “Technology evolves rapidly, and LLMs are just a part of the AI architecture; principles like decoupling and encapsulation are still valid.”
He notes compliance and regulation are picking up, but in the meantime, organizations can concentrate on data usage, which is a typical case.
“Understanding what AI is doing with your company’s data assets is the key, and in that sense, AI can be considered just another component in your data landscape,” he says.
When it comes to balancing AI ambition with risk tolerance, Carruthers says now is not the time to remain stagnant and wait for someone else to bear the brunt of potential regulation or compliance issues.
“It’s actually the perfect opportunity to be bold, but bold in the right way,” she says. “Take action, learn, conduct experiments and figure out how AI works for your organization.”
The best way forward, from her perspective, is to start by addressing the smallest problems and solving them.
“Through this process, organizations will learn how AI fits into their own framework,” Carruthers says. “Don’t feel compelled to tackle only the most significant challenges.”