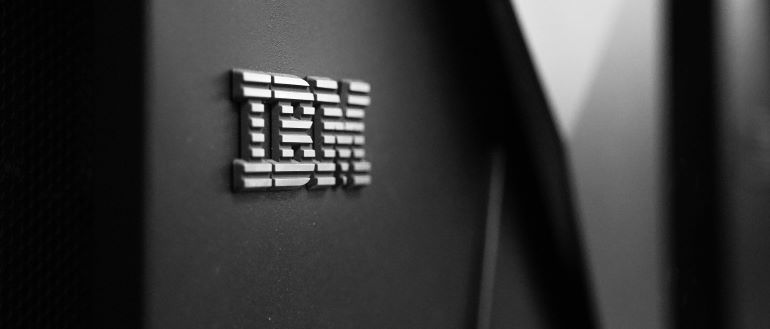
IBM has confirmed its decision to channel elements of DeepSeek’s artificial intelligence (AI) models into its WatsonX (watsonx.ai) platform. The company says the move to work with the Chinese firm’s technology is validated by a commitment to open source innovation in AI. With an eye on the undeniably higher cost of US-originated and maintained AI models, IBM says that this action will broaden watsonx.ai’s ability to perform secure reasoning going forward.
To integrate with Deepseek, IBM will use what it defines as “distilled versions” of DeepSeek-R1 to include the “best open source models” available from anywhere around the world.
What Is Knowledge Distillation?
Model or knowledge distillation is an accepted practice in machine learning (ML) undertaken to extract a selected pool of data from a larger AI model that is more expansive – and typically not fully utilized – and more computationally expensive to operate.
Not to be confused with retrieval augmented generation (RAG) for domain-specific narrowing or model compression often found in edge environments, IBM’s model distillation here is designed to give it a degree of additional control on various levels.
This decision by IBM underlines a widening epiphany across the IT industry that in AI circles, if not elsewhere, big expensive proprietary systems do not always win the day. Referencing its own research lab work, IBM says that by focusing on work with “fit-for-purpose” AI models (i.e. not as generalized or bloated), it has seen up to a 30-fold decrease in AI inference costs for certain training procedures.
Writing on LinkedIn, IBM CEO Arvind Krishna stated, “For too long, the AI race has been a game of scale where bigger models meant better outcomes. But there is no law of physics that dictates AI models must remain big and expensive. The cost of training and inference is just another technology challenge to be solved. We’ve seen this play out before. In the early days of computing, storage and processing power were prohibitively expensive. Yet, through technological advancements and economies of scale, these costs plummeted. AI will follow the same path.”
Misleading Economics?
DeepSeek’s latest AI model known as R1, which followed DeepSeek-V3, arrived in January of this year. Described as a highly performant but lower-cost alternative to domestic US model technologies, some have questioned the perhaps “misleading” economics at play here. A study from Bank of American Securities said that the claimed $5.58 million cost of training DeepSeek’s model does not necessarily include a full consideration for research, algorithm creation, refinement and management as well as other data expenses.
In a wide-ranging analysis made in January of this year, Anthropic CEO Dario Amodei called out some of the related misgivings he and others have over DeepSeek’s cost to develop. He said the that work carried out is impressive, “but not anywhere near the ratios people have suggested” and so needs to be viewed at a high level and as an innovation cycle that has happened over a period of time.
“All of this is to say that DeepSeek – V3 and other models – are not a unique breakthrough or something that fundamentally changes the economics of large language models (LLMs); it’s an expected point on an ongoing cost reduction curve. What’s different this time is that the company that was first to demonstrate the expected cost reductions was Chinese,” wrote Amodei, on his own blog.
On and On Jevons
Notwithstanding the naysayers (and there would arguably be some layer of already absorbed production costs that are made less prevalent by DeepSeek), most commentators agree that AI costs can now be more adroitly reduced. In a clear nod to the Jevons Paradox (i.e. when a resource becomes more efficient, usage and demand increases), IBM’s Krisha appeared on Bloomberg television to say, “We will find that the usage will explode as costs come down. I think it is a validation — we have been on the point that you do not have to spend so much money to get these models,” he said.
Krisha’s onward mantra states that the cost of AI model development should be “in the millions and not in the hundreds of millions” and that IBM is eager to apply fit-for-purpose, domain-specific AI across the watsonx.ai platform.
Without question, this is a time of great change in AI model development. As the DeepSeek website tagline says: Into the unknown.