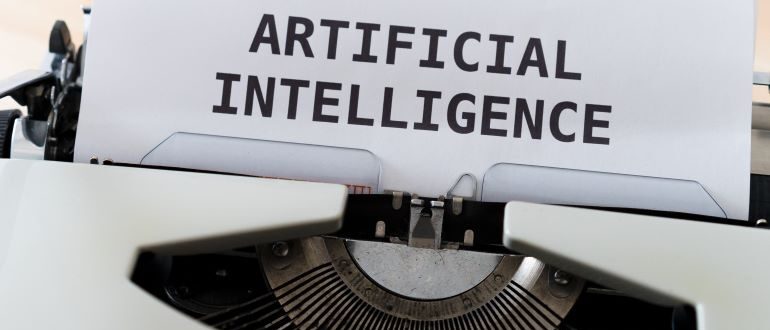
For enterprises, the first phase of generative AI has been about content creation and answering questions. These capabilities have become pervasive across many applications.
Then what’s the next phase of generative AI?
It’s AI agents.
“Our customers want their systems to take actions,” said Abhi Maheshwari, who is the CEO of Aisera. His company develops agentic systems for many large enterprises.
“Customers want deep integrations with their enterprise applications. For example, if an employee asks about benefits, this person can also make changes, say by adding a beneficiary or including a new benefit. This can be completely self-serve. Basically, with agentic systems, enterprises can realize significant outcomes, in terms of cost savings, efficiencies, productivity and even revenue opportunities.”
Consider a recent survey from Capgemini. About 10% of the respondents say that they are using AI agents. But this is expected to increase significantly. The survey shows that 82% of the respondents are likely to deploy AI agents within the next one to three years.
So in this article, we’ll explore the role of AI agents in transforming enterprise operations, diving into how these advanced systems will drive the next phase of generative AI.
The Basics
The term “AI agents” is somewhat vague. Then again, the technology is nascent and undergoing much change.
“An AI agent can mean anything from an LLM or more complex orchestration involving multiple LLMs, RAG systems, or even traditional ML models,” said Julia Neagu, who is the CEO and Co-Founder at Quotient AI. “Companies tend to not publicize what’s under the hood of AI agents.”
Yet there are still some common components of an AI agent. According to Ritu Jyoti, the Group Vice President of WW AI and Automation Research Practice at IDC, they include the following:
- Planning: AI agents can plan and sequence actions to achieve specific goals.
- Tool usage: AI agents can use various tools, such as code execution, search and computation capabilities, to perform tasks effectively. AI agents often use tools through function calling.
- Perception: AI agents can perceive and process information from their environment, to make them more interactive and context-aware. This information includes visual, auditory and other sensory data.
- Memory: AI agents have the ability to remember past interactions (tool usage and perception) and behaviors (tool usage and planning). They store these experiences and even perform self-reflection to inform future actions. This memory component allows for continuity and improvement in agent performance over time.
The Use Cases
A key to AI agents is autonomy, which sets themselves apart from copilots. That is, a user can ask a system to achieve a goal. “A generative AI agent can break down complex tasks and lean on purpose-built sub-systems,” said Steven Hillion, who is the senior vice president of data & AI at Astronomer.
What this means is the potential use cases are seemingly endless. But currently, there are certain areas where generative AI agents have shown to be most useful for enterprises. “Just some include customer service agents, service desk agents. automated software engineering, automated vulnerability testing, automated codebase migrations and HR help desk,” said Jyoti. “From an industry use cases aspect, they can automate KYC compliance and insurance claims status.”
One notable use case is from Klarna, which is a top fintech company. It partnered with OpenAI to create an AI agent for customer service. It not only answered questions but provided for actions like refunds and returns.
Within a month of the rollout, the generative AI agent did the work of about 700 full-time human agents. The rate of repeat inquires dropped by 25% and resolutions took less than 2 minutes, versus 11 minutes. There was also no drop in satisfaction scores.
Klarna expects that the AI agent will lead to $40 million in profit improvement for 2024.
The Risks
The “brain” of a generative AI agent is an LLM (Large Language Model). This means there are risks of hallucinations, biases and challenges with integrations with proprietary data. But techniques like fine tuning and RAG (retrieval augmented generation) can help to mitigate the problems. Moreover, the use of multi-agent systems can do so as well. This is where different LLMs work together to improve outcomes.
However, the complex flows of agentic systems can easily lead to problems. “Ensuring that the generative AI agents follow their task can be difficult,” said Sandi Besen, who is an applied artificial intelligence researcher at IBM. “They have a tendency to want to do everything which can lead to inefficient agent teams. This can mean wasted tokens. Also, the reasoning capabilities of the AI models are sometimes not strong enough, depending on the complexity of the task. It can result in the inability to call the right tools in the right sequence.”
These are certainly major concerns. But the fact is that AI agents continue to make significant strides, fueled by substantial investment – whether by megatech firms or VCs. These technologies are evolving at a remarkable pace, and the potential they hold for transforming industries is immense.