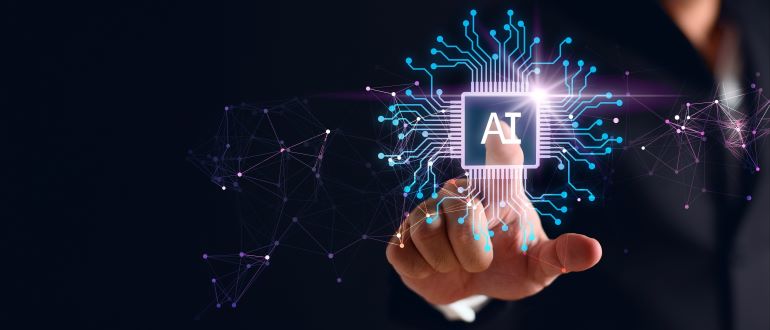
Enterprises are rushing to deploy GenAI, but are they using this new technology in the best areas for their business? “When an organization looks into deploying GenAI, it’s like they’re bringing a new team member on board,” says Pruthviraj Haral, cofounder of developer software and engineering analytics provider DevDynamics. “They need to find the right fit, areas where this new ‘digital colleague’ can shine. It’s not about replacing people but enhancing what they do best,” Haraj advises.
Where should enterprises choose to try to gain those potential enhancements through GenAI? Haraj says that’s like figuring out where the organization needs an extra pair of hands or a digital brain. “Some tasks are just made for GenAI – like crunching big data, automating routine stuff, or even being a creative spark in design or writing. The key is to play to its strengths without stepping on human toes,” Haraj adds.
Getting Strategic with GenAI
Wienerberger Group, the world’s largest brickmaker and a provider of sustainable building and infrastructure products, needed an extra pair of hands and a digital brain for its IoT analysis efforts to reduce energy consumption and improve product quality. To do so, Wienerberger used AI and IoT analytics services from SAS, running SAS Viya on Microsoft Azure Cloud. Wienerberger hopes to be climate-neutral by 2050.
Wienerberger collects data from various sources, including IoT edge devices and sensors within its factories and environmental data, to reach its goals. With this data, Wienerberger can identify shifts in weather and other fluctuations leading to inefficiencies and wasted energy consumption. Its kilns dry bricks running at 1,475 degrees Fahrenheit. By using this data, Wienerberger could reduce fluctuations that could impact production.
SAS worked with Wienerberger to develop a digital twin of its brick production processes. The AI-powered digital twin can identify ways to lower energy consumption and hit climate-neutral objectives for Wienerberger.
Florian Zittmayr, team lead for data science at Wienerberger, says the company uses SAS AI and IoT analytics to link its data streams and analyze its production process.
Wienerberger is expanding its use of advanced analytics to reach 149 facilities in 23 countries one day.
While each company is different, like Wienerberger, organizations should strive to find strategic areas to deploy GenAI that will help them reach their long-term goals. Success will depend upon multiple technical, ethical, and operational factors. Here are the key considerations:
Start with staff and skills. Evaluate existing talent for the right mix of technical skills, business domain experience and expertise. Organizations will be better positioned to develop and maintain their GenAI deployments by teaming domain experts with data scientists.
Lay the groundwork with data prep. Because effective GenAI requires context-specific, clean, accurate and available data, most organizations start their GenAI efforts in areas where they can access such data. “We generally see GenAI applications in areas where the client has access to – either internally or through external partnerships – significant amounts of data, and where applying GenAI on that data allows them to bring greater speeds, a better experience, and lower costs to their business outcomes,” says Maulik Bhagat, executive vice president of services and innovation at technology and management consulting firm AArete.
To date, Bhagat says the areas where companies have such data are customer service and experience, knowledge management, legal and contracting, and operations. “It is also moving quickly to areas like R&D and innovation, subject to appropriate IP compliance, transparency and protection,” Bhagat adds.
Build a scalable infrastructure. GenAI requires a rock-solid infrastructure that’s scalable and secure. Success here addresses the unique demands of data storage, computing power, network connectivity and security that are all critical to the effective deployment and operation of GenAI systems. Over the long term, this requires the right architecture, operating model and governance structure.
Address deployment challenges. GenAI deployments will come with unique challenges, and organizations should prepare to address them. This includes model size and latency, bias, fairness and safety.
Ongoing monitoring will ensure that, regardless of where GenAI is initially deployed, its performance remains within company policy and values and attains desired business goals.
Evaluate enterprise-specific considerations. Of course, enterprises should consider the business risk versus the rewards of GenAI deployment, including the use and security of potentially proprietary data and the security and privacy of the content the models generate. “Like any investment project, there should be a business case backing the introduction of GenAI, including identifying the business problem it will solve,” says Manfred Kügel, data scientist and IoT industry advisor for AI and analytics provider SAS.
Kügel notes that, as with Wienerberger, a strategy involving the integration of GenAI with a digital twin must be well planned and have all required elements before it goes live. “These include a robust, scalable data architecture that manages a diverse set of real-time and enterprise data; advanced analytics that process the data and uncover opportunities to optimize any anomalies; a way to use the analytics to test scenarios; and a method to efficiently transfer analytical findings to the right people within the organization,” says Kügel.
Governance and Security
Finally, a governance model should be developed over the GenAI model and the data that feeds into it. As it is monitored, lessons will be learned that can be applied to future GenAI deployments. While developing such a governance plan can be time-consuming, the process will make GenAI more manageable, and the policy can be regularly updated as the legal, regulatory and security landscape changes.
“Risk assessment is another major step. We must be mindful of ethical considerations and data security. It’s about being responsible and compliant as we embrace this powerful tech. Feedback from stakeholders – employees, customers, and partners – is also crucial. It helps us gauge the impact and refine our approach,” explains Monica Cisneros, product marketing for AI at data science and analytics software provider Alteryx.
Regarding initial deployments, Chandini Jain, founder, and CEO of Auquan, says the sweet spot for generative AI in the enterprise is the knowledge-intensive use cases that require significant manual effort or could benefit from speedier analysis. “These could be workflows central to the organization’s competitiveness, but today, they rely on expensive manual effort to process noisy data that could otherwise be invested in strategic decision-making. These could also be use cases that require big analyst teams to source, process, and analyze large volumes of unstructured data into actionable insights,” says Jain.
Cisneros adds that strategic thinking, careful planning and staying open to new ideas and feedback are vital ways to navigate the Gen AI journey in an organization. The first step is getting to grips with what the organization aims to achieve and currently needs. This means senior management and various departments need to come together, like a think tank, to pinpoint where GenAI can make the most impact in line with company goals,” Cisneros says.
Finally, Cisneros says to narrow the list of GenAI possibilities, considering what data and technologies already exist within the organization. This involves the chief data and analytics officer stepping in for a data check-up and ensuring that the data needed is high quality, relevant and on the right side of ethics and privacy laws. “The CIO also plays a key role in figuring out if the GenAI solutions you are eyeing can work smoothly with existing systems,” Cisneros explains.
“Once you have your data and infrastructure in place, consider starting pilot projects before a full rollout. They’re like test runs that give us a real-world sense of how GenAI will perform and scale in our organization,” says Cisneros.