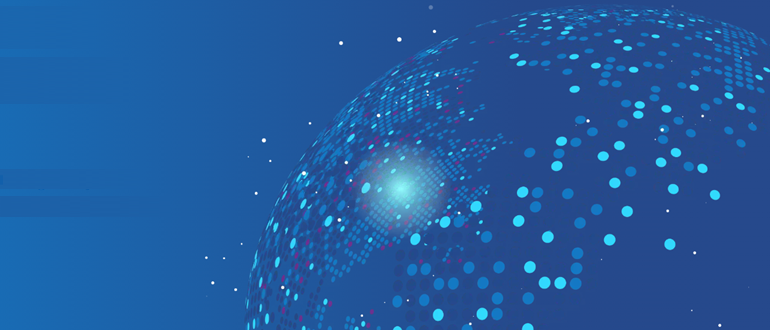
Catchpoint has just released its first generative AI tool benchmark report in an effort to try and establish what elements of the increasingly intelligence-automated IT stack AI engineers and their DevOps teams should be most alert to.
Known for its Internet Performance Monitoring platform, Catchpoint’s vested interest in the effectiveness of AI tools stems from its approach to not only Service Level Objective (SLO) monitoring, but its wider approach to focus on Experience Level Objectives (XLOs) monitoring.
Cost Of Connectivity
Conducted internally using its own analysis methods, Catchpoint’s generative AI benchmark seeks to calculate what the company calls the “true cost of AI connectivity” and examine failures that manifest themselves as performance gaps in AI tooling and toolsets.
“As AI tools become integral to everyday business operations, the stakes of their underperformance grow higher. Connectivity issues, sluggish response times and poor regional infrastructure — like delays in IBM Watson’s performance or server issues at Meta AI — pose serious threats to global productivity. When millions of workers depend on AI for decision-making and task management, even brief disruptions can result in billions in lost revenue and opportunities,” details Catchpoint.
In its quest to find the real cost of AI in both positive and negative terms, Catchpoint reminds us that analyst house McKinsey reported that nearly 50% of organizations now use AI in at least one business function. That said then, when poor performance surfaces (such as Google Gemini’s 12x slower wait times in regions like South Africa), there could be implications for businesses and entire markets that lead to financial losses.
World Economic Forum
Catchpoint’s affable and approachable CEO Mehdi Daoudi reminds us that AI tools are essential to job creation and innovation. The World Economic Forum estimates that as many as 97 million new jobs could emerge from AI and automation. Underperforming AI tools could therefore impact (and perhaps hinder) this job growth.
From the report itself, H2O.ai emerged as the fastest generative AI platform across global benchmarks. For background here, H2O is a fully open source, distributed in-memory machine learning technology with linear scalability that supports widely used statistical and machine learning algorithms including gradient-boosted machines, generalized linear models and deep learning. H2O.ai’s optimization in user interaction, with a response time of 216 milliseconds, places it ahead of competitors like IBM Watson and ChatGPT.
Not So ChattyGPT?
Not afraid to call the shots, Catchpoint says that ChatGPT exhibits a “painfully slow authentication process” to produce what the company says could be a user experience nightmare.
“ChatGPT struggles with user authentication speeds, especially in regions like South Africa, where login times can exceed 3,000 milliseconds [that’s 3 seconds, if you don’t speak millisecond-speak] and multiple redirects during the process contribute to these delays, frustrating users who expect quick access to AI services. For businesses relying on AI tools, such inefficiencies could make ChatGPT a less viable option, particularly as companies juggle multiple third-party systems. In time-sensitive operations, even a one-second delay can make an AI tool impractical for real-time decision-making,” reports Catchpoint, in its analysis.
In total, Catchpoint focused on ChatGPT, Google Gemini, H20.ai, watsonx.ai and Meta AI. To evaluate the end-to-end user experience, the company monitored the following aspects for each AI tool: Homepage load time; user authentication process; and time time taken by each AI tool to respond to user questions.
The benchmark included measurements across multiple Internet Service Providers (ISPs) and cities in the following countries: Australia, Canada, India, Italy, Mexico, Singapore, South Africa and the United States.
Where Latency Is Laborsome
Much of the analysis here made use of so-called “connect time” i.e. a measure used to assess the duration taken to establish a Transmission Control Protocol (TCP) connection with the server. This metric is crucial for understanding network latency. A high connect time can create a ripple effect, negatively impacting other metrics and ultimately leading to a poor user experience. Importantly, this shows that it is not the AI function necessarily harboring latency per se (although that can happen too depending on cloud workloads and a plethora of backend systems management issues), so a rounded and holistic view of the market is needed.
“Our GenAI Platform Performance Benchmark Report underscores the critical role that performance plays in the success of generative AI tools. As companies increasingly integrate these platforms into their operations via APIs, ensuring optimal performance at every touchpoint becomes vital. That’s where Catchpoint’s Internet Performance Monitoring (IPM) comes in. As the demand for seamless AI integration grows, companies must monitor generative AI platforms to deliver fast, scalable and reliable user experiences,” stated the company.
With products like Tracing and WebPageTest, Catchpoint suggests that its IPM technology enables in-depth analysis of generative AI platform performance, helping to pinpoint bottlenecks in API interactions, authentication flows or query response times. If we follow all the arguments laid down here, we might be at a point where we can say that integrated Internet telemetry might be where AI smartness needs to start.