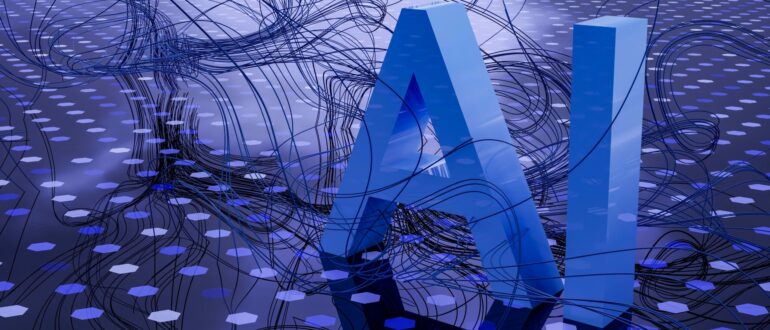
A computational tool leveraging machine learning has been developed and used by a team of art scholars and AI experts to authenticate the paintings of the renowned Italian Renaissance artist Raphael, according to a paperĀ in Heritage Science Journal.
Focused on a restricted set of artworks by Raphael, the study utilized advanced techniques in image processing and deep learning, achieving what the research team said was 98% accuracy in classifying authentic paintings.
The tool targeted visual analysis and authentication of a specific class of paintings, particularly those attributed to Raphael.
Transfer learning methods were applied to a deep neural network, facilitating feature extraction for authentication purposes. Edge detection algorithms, pivotal in capturing Raphaelās distinctive artistic style, were integrated into the authentication process.
While the results revealed a high degree of accuracy in classifying authentic Raphael paintings during validation, the study emphasizes that this computational tool contributes only a segment to a full authentication protocol.
Other factors typically considered include provenance, historical context, material studies, and iconography.
Professor Hassan Ugail, director of the Centre for Visual Computing and Intelligent Systems
at University of Bradford and lead author of the paper, explains the driver of these studies is to try to address the challenges and limitations of traditional art attribution methods.
“Traditional techniques, often based on expert connoisseurship, can be subjective and are sometimes hampered by incomplete historical records,” he says. “The objective here is to introduce a more scientific and objective approach to art attribution.”
He adds Raphael’s paintings were chosen for this study because of the team’s previous study on another work.
“Our results strongly suggest that the Tondo is a painting by Raphael, while some art historians do not believe so,” he says. “Raphael is a central figure in Renaissance art, and his works have been subject to various attribution debates. Therefore, it forms an interesting case study to demonstrate the use of AI as part of the art authentication process.”
The significance of the study lies in its potential to supplement traditional art authentication methods with AI-driven computational tools.
“Given the significant consequences to art scholarship and the commercial world of art forgery, misattribution, and the natural ageing of artworks, establishing a paintingās authenticity is of paramount importance,” the report noted.
The study could hold promise for broader applications across various artists and artistic styles.
As machine learning and image processing continue to advance, this method could become an essential tool in the array of approaches for artwork analysis and verification, supplementing existing scholarly analyses and imaging techniques used by art historians and collectors worldwide.
Building on the knowledge gained from specific case studies, the focus of current (and future) research is to focus on building a versatile, general tool that can be applied across a broad spectrum of artists and artworks.
“The goal is to create a system that can be trained and tested on paintings from almost any artist, not just limited to a specific case or individual,” Ugail says. “This adaptable approach will make the tool immensely valuable in the field of art authentication.”
He admits the use of AI in art authentication is viewed with a mix of intrigue and caution by art historians–while some see it as a groundbreaking tool that can offer new insights and support traditional methods, others are wary of its over-reliance and potential to overlook the expertise of human connoisseurs.
“AI can process large data sets, identify patterns and anomalies, and provide objective analyses that human experts can interpret,” he points out. “Therefore, it can be a very useful tool for human experts to use alongside other established techniques.”
From Ugail’s perspective, the primary risks inherent in using such technology include over-reliance on AI.
“Potential errors in AI algorithms can occur. AI requires sufficient and clean training data,” he cautions. “Conservation professionals, art historians, and academics are likely to view AI as one tool which is part of a more extensive toolkit.”
He says he thinks many in the art world are beginning to understand the value of combining scientific analysis with historical and stylistic expertise.
“The legitimacy of AI in this field will grow as its accuracy and utility are proven through successful case studies,” he adds.