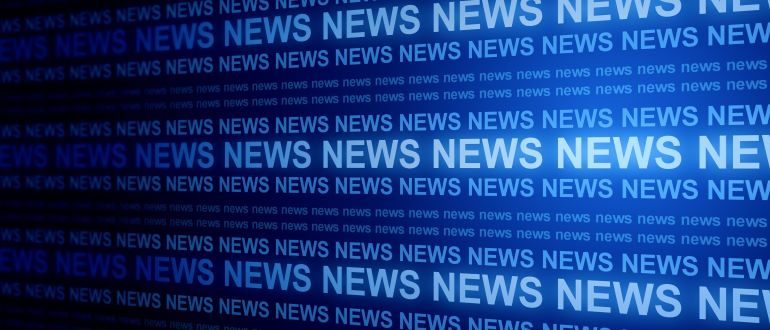
It’s getting easier to build artificial intelligence (AI) models as providers of data analytics and management platforms add development environments to simplify the process. SAS this week previewed a lightweight development environment dubbed SAS Viva Workbench that enables developers to use Python, R or the SAS programming language to build an AI model alongside SAS App Factory, a rapid application development environment based a cloud-native tech stack built on React, TypeScript and Postgres that is integrated with Jupyter Notebook, Visual Studio Code and SAS Enterprise Guide client tools.
Announced at the SAS Explore conference, both tools serve as the foundation for a suite of offerings the company intends to provide for use cases within multiple vertical industries starting with a SAS Energy Forecasting Cloud platform scheduled to be available next year.
SAS is moving down two AI paths. The first is to make it simpler for end users to invoke generative AI capabilities from within an analytics application. The second is to make it easier for organizations to leverage the data collected via SAS platforms to build AI models without necessarily having to acquire extensive prompt engineering expertise, says Shadi Shahin, vice president of product strategy for SAS. “We’re providing co-pilot capabilities,” he adds.
Organizations of all sizes are going to have to determine to what degree they will want to invest in data science skills such as prompt engineering versus relying on platforms that will increasingly automate many of the processes and tasks that today are needed to construct an AI model. That level of automation will be especially critical as AI moves beyond being a science experiment to a capability that is pervasively embedded within almost every application. The pace at which AI models can be retrained, replaced or simply updated will become more crucial as businesses become increasingly more dependent on them.
In the meantime, organizations should expect to see massive gains in productivity as it becomes simpler to use natural language interfaces to invoke analytics applications. In theory, businesses have been investing in analytics applications to make better decisions faster for years, but in practice achieving that goal required a level of expertise that is beyond the average business user. Now the average user will be able to frame a natural language query to, for example, understand the impact of an event and even model what-if scenarios. In effect, analytics applications are about to be utterly transformed as access becomes more democratized, notes Shadin.
Naturally, there is now a race on to take advantage of AI, largely driven by the fear of being left behind. That frenzy may lead to some irrational AI exuberance among business leaders, but over time it’s already apparent machines will be capable of automating an increasing array of tasks, including surfacing insights generated by analytics applications that are accessing large language models (LLMs) that enable generative AI.
The issue now is not only level-setting expectations, but also preparing a workforce for a brave new world where insights based on actual data are readily available to anyone.