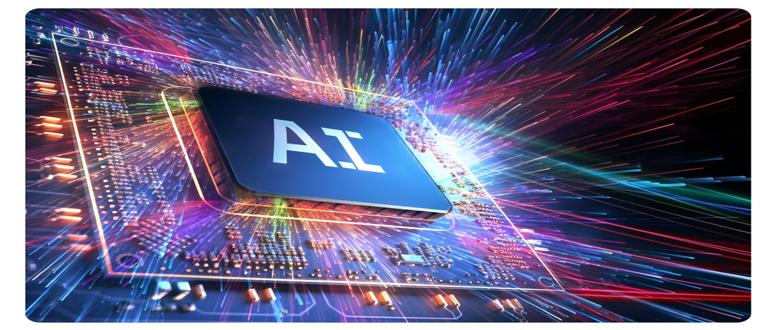
AI innovation is advancing at lightning speed. LLM-powered chatbots already seem quaint; we are entering the agentic era, where AI systems can navigate complex workflows, make decisions autonomously and leverage other software.
Enterprise AI adoption is speeding up, as well. Open-source models, cheaper inference costs and low- and no-code AI developer tools are allowing organizations to finally move from pilot to production.
By comparison, it can seem like enterprises’ AI governance initiatives are standing still. And sometimes, they are – businesses use the same informal and ad hoc governance approaches they had in place at the start of the decade.
This is a troubling trend. Outdated AI governance does not just invite risks like harmful outputs and compliance violations. It also hampers progress, because governance plus innovation is the best formula for scaling responsible AI.
Antiquated Governance
Imagine if today’s aviation industry operated with guardrails from another era: No mandatory black boxes, no Aviation Safety Reporting System (ASRS). Or imagine if today’s pharmaceutical industry operated without contemporary policies like trial transparency and robust pharmacovigilance.
It is an alarming thought. But that is how some enterprises treat AI governance.
Many organizations do not take a lifecycle approach to governing AI systems. Instead, they monitor performance and outputs primarily during deployment – and not on an ongoing basis. This means significant issues like latency and accuracy are less likely to be spotted during the development and testing stages, making them costlier to address downstream. Meanwhile, an ad hoc approach means staff need to pull reports manually and for specific periods. This undermines explainability and transparency in addition to wasting precious time and energy.
Poor AI performance is not the only issue exacerbated by incomplete lifecycle management. Harms like bias and drift can proliferate, too. These harms are even more problematic in agentic AI systems, which act with greater autonomy and have the potential to make high-stakes business decisions.
Another antiquated governance habit is a static approach to compliance. Not long ago, enterprises had to comply with fixed regulations about technology and data. That is not the case anymore. The regulatory landscape is evolving fast: The AI Act is rolling out across much of Europe, and many other governments are following suit. The regulatory landscape is also deeply fragmented: Many U.S. states are introducing and enacting unique AI regulations. In this dynamic environment, a static, monolithic approach to compliance cannot succeed.
Advanced Governance for Advanced AI
How can enterprises ensure their AI adoption and AI governance are progressing at the same speed?
First, organizations need to govern AI across its entire lifecycle. This means starting an audit trail at the time the use case is introduced and capturing governance information through use case review and approval, engineering and deployment. As a best practice, AI governance requires always-on observation, guardrails and fact sheets. Further, emerging agentic systems require agentic system metrics for monitoring and alerts. Organizations should create a single view of all agentic applications in development and use, and include experimentation tracking and traceability in the engineering phase. Embedded evaluation nodes directly within the technology can also monitor metrics like answer relevance, context relevance and faithfulness.
Organizations also need a dynamic approach to AI compliance. This means leveraging technology that tracks the regulatory environment and automates the mapping of new mandates onto relevant enterprise technology, and flags potential violations.
Breaking down walls between governance and other disciplines is equally important. For example, AI governance and AI security are complementary, but often exist in silos. When the teams and tech are integrated, the sum is greater than its parts – the security side can detect shadow AI and automatically route it to the governance side to align with the relevant use case(s), perform risk and compliance assessments, and ensure effective controls are in place.
As AI innovation and adoption gain momentum, AI governance must, too. Enterprises that invest in one but neglect the other invite real risks – including lost opportunities.