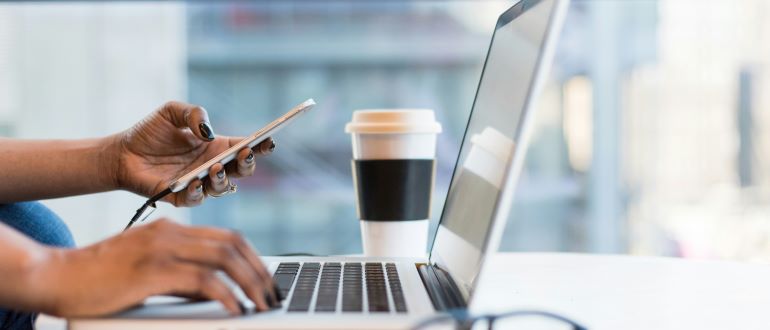
In quality engineering, generative AI has emerged as a transformative force, fundamentally shifting classic testing paradigms. Traditionally, AI models focused on utilizing existing data for classification and prediction, often applied in test prioritization and consolidation. However, the rapid integration of generative AI this past year or two has shifted the entire base toward new testing solutions, a next level in the race for AI aptitude.
Generative AI has Changed Testing to Actively Engineer Quality
In the past, testing quality was a big concern, necessitating early integration into the development life cycle. Now, with generative AI, the focus has advanced beyond simply assurance to actively engineering quality. The key distinction lies in the approach: Classic AI involves human intervention and manual processes, while generative AI automates and innovates testing methodologies.
Consider the scenario of dealing with requirement quality early in the Software Development LifeCycle. Using classic AI, a business analyst might define requirements to cover various interpretations, leading to ambiguity and potential failures. With generative AI, this type of requirement ambiguity is not only just identified; it is remediated too. For instance, if you’re a retailer testing for different types of footwear, perhaps you’d want testing for different footwear. Now if I were to ask you, “What does the retailer mean by different footwear?” this could be different shoe types, like sneakers, heels, or perhaps sizes, or details like plushiness, width — essentially, it could mean so many different things. But now, generative AI models can automatically understand the context of the customer and industry, and remediate the requirement to remove ambiguity.
Another example is the consolidation of similar test cases. In the past, traditional AI models could identify duplicate or similar test cases, but human intervention was still required to remove or merge those duplicate or similar test cases. Generative AI takes this a step further by automating the consolidation process, reducing the need for manual intervention and ensuring more efficiency in testing.
Generative AI Moves Into Auto-Creating Test Cases
Pushing the boundaries beyond predicting or analyzing, generative AI extends now to auto-creating test artifacts like test scenarios, test cases, features files and even automation scripts. While the world of developers was fairly advanced with smart Integrated Development Environments (IDEs), and code generators, the world of QA was still limited to automation with not too much play for AI in testing. With the introduction of GenAI mid-last year, adoption for AI in testing has grown at a rapid speed.
GenAI has democratized AI in testing. If someone feeds a validated, non-ambiguous, and high-quality requirement into current generative AI models, test scenarios along with well defined test cases, feature files, and automated selenium scripts can be generated with a simple click. This transformation has significantly accelerated testing processes, turning what used to take weeks into a quick automated task done in days.
Using Generative AI is Not About Replacing Human Testers
It needs to be reiterated often to the masses: Using generative AI is not about replacing human workers, but about enhancing their capabilities. The shortage of senior automation testers often results in lost business revenue. However, with generative AI, junior engineers can now harness the power of GenAI enabled automation, performing tasks with the built-in knowledge of a seasoned architect.
Generative AI’s prowess is not arbitrary; it has learned from billions of data points. By combining traditional knowledge with AI capabilities, new solutions bring scalability and speed to testing, putting it on steroids. This transformation is not just an enhancement; it’s a total revolution that opens up new possibilities in testing. Beyond automated test case generation, generative AI has extended its impact to synthetic data creation, bringing a new dimension to the whole testing process.
It’s Time for Testers to Embrace Generative AI
From resolving ambiguity to automating test case creation, generative AI has advanced testing into a new era that will only continue to accelerate. The time and cost savings, along with increased efficiency, make it a transformative force in the testing landscape.
While the future implications of generative AI are vast and somewhat unknown, we do understand how it has not only elevated testing but has also opened up avenues that once seemed impossible. The connection between human expertise and generative AI capabilities is reshaping the testing landscape, promising a future in which testing is not just about assurance — but about generating quality with speed and at scale.