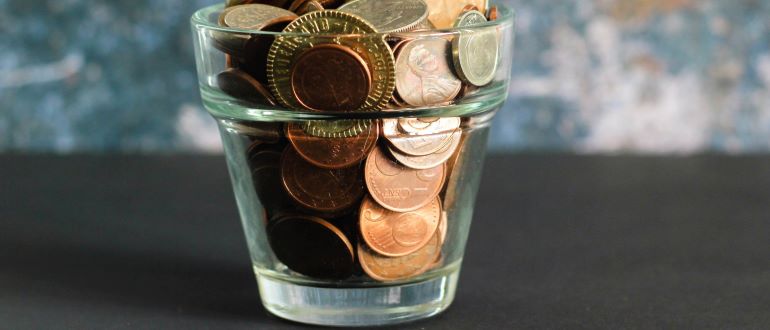
Many experts agree that cloud spending will increase this year, primarily due to the increased adoption of AI and GenAI. Forward-looking companies are already exploring using these technologies to better understand the costs. Companies must develop and implement management strategies to maximize investments for long-term growth.
Synergistic Relationship Between Data and AI
What is the connection between data and AI? High-quality data—and lots of it—is necessary to reap the benefits of AI. When large amounts of data are analyzed using an AI algorithm, the results will be more impactful. Yet, analyzing large amounts of data can be costly, especially if the information is stored in the cloud.
AI solutions differ from one another. To illustrate, consider natural language processing (NLP). NLP taps into a large amount of data and can access numerous applications, including automating customer service, summarizing documents and generating emails. The complexity of each task increases significantly when businesses need contextualized responses, mainly when dealing with sensitive data in heavily regulated industries such as utilities or telecommunications.
Newer AI models can be anywhere from ten to 100 times larger than recent AI models. These larger models are highly intricate, and with more data comes more analysis and computing power, which increases cloud costs.
Another issue to consider is integrating content from the cloud into existing IT infrastructure. Many companies used a lift-and-shift migration a few years ago, sometimes called rehosting. However, that proved quite costly, and businesses frequently paid for more services than they could use. When looking into cloud usage, companies should identify ways to eliminate overspending.
Optimizing Costs: A Strategic Approach to Cloud Economics
The company must set proper expectations for AI and GenAI to deliver value. Success largely depends on collaboration between departments involved with AI-based projects. Having a shared framework is critical.
One way to ensure that costs for AI initiatives are under control is to do a cost-benefit analysis. Doing so helps align the business’s priorities with cloud investments. While cost reduction and improved efficiency are common goals, the key is delivering business value and ensuring that AI initiatives align with long-term business goals. Utilizing intelligent workload management, which helps automate resources, is another way to open resources for other projects.
Establish a Cloud CoE
Another effective approach is to create a dedicated team focused on cloud economics, even creating a cloud center of excellence to serve as the central source for all cloud information. When businesses decide where to allocate funding for cloud initiatives, a central team should examine the entire company’s AI landscape to ensure that objectives and best practices are met.
One crucial strategy centers around variable cost models to support AI investments, forecasting and adjusting over time. A variable cost model can make cloud-based AI investments more financially manageable and adaptable to changing business needs by aligning costs with actual usage. Understanding variable cost models is critical as our research indicates that 34% of organizations in North America need a coordinated or centralized approach to managing their cloud investment. Fixed cost models allow companies to pay an established amount regardless of usage, compared to a variable cost model with a pricing structure to understand where costs fluctuate based on usage and demand. Key elements to understand variable cost models include:
- Pay-as-you-go: Pay for resources, including computing power, storage and data transfer.
- Scalability: Costs rise as AI workloads increase, while costs decrease if you reduce your workload.
- Flexibility: Variable cost models allow you to scale up or down based on your needs without being locked into a long-term contract or upfront payment.
- Cost Efficiency: This model can be cost-effective for businesses with fluctuating workloads or those in the experimental phase of AI projects since you avoid over-provisioning and paying for unused resources.
- Resource Management: This model requires careful monitoring and management of resources to avoid unexpected cost spikes, which can occur if AI tasks suddenly require more computing power or storage.
- Usage Metrics: Cloud providers typically offer detailed usage metrics and cost management tools to help you track and control your expenses.
Reaping the Benefits of Cloud Management
Leveraging cloud management technology improves decision-making, forces companies to examine resource allocation and aligns AI investments with broader business objectives. Increasing investment in cloud management technology puts companies in a better position to transform business operations, reduce waste and improve operational efficiency.
Examining and understanding cloud economics delivers the highest level of business value and goes a long way toward securing long-term success.