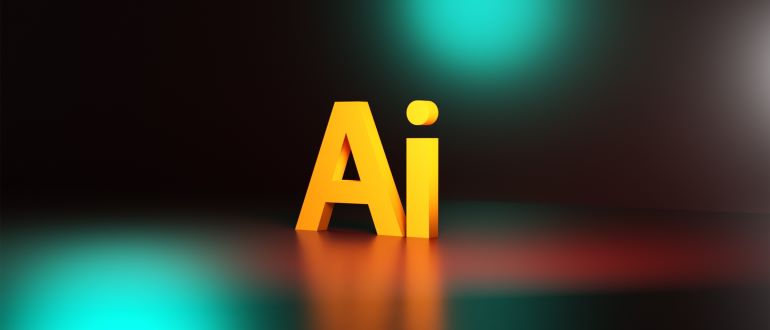
As organizations adopt AI, scaling from pilot programs to full production presents a new set of challenges. For both startups and enterprises, the transition from early experimentation to mature AI operations—often referred to as “crossing the chasm”—requires overcoming significant infrastructure bottlenecks. Without the right strategies, growing AI workloads can outpace capacity, delay innovation and drive up costs, making scalability a daunting challenge.
Whether dealing with GPU shortages, complex resource management, or the evolving demands of multiple teams, projects, and security requirements, companies must recognize these bottlenecks early and plan their infrastructure accordingly. Understanding these challenges is essential for ensuring that AI investments don’t stall before reaching their full potential.
Key Bottlenecks That Slow AI Growth
- Constrained Access to High-Performance GPUs
The demand for high-performance GPUs—critical for AI model training and inference—has surged across industries. However, supply constraints and long lead times make it difficult for enterprises to get the hardware they need, leading to delayed projects. This creates a bottleneck at a critical point in AI development, where having the right compute resources directly impacts time-to-market and competitiveness. For example, this benchmark comparison highlights the substantial performance differences across successive iterations of NVIDIA’s flagship models. These improvements translate into greater cost efficiency, offering more value for the same or lower operational expenses. - Resource Management Across Teams and Departments
As companies expand AI use cases, managing compute resources becomes more complicated. Enterprises need to balance multiple workloads across teams—each with different demands and timelines. Without effective management, resource fragmentation and underutilized infrastructure can occur, resulting in inefficiency and increased costs. This problem is exacerbated in multi-tenant environments, where multiple projects must share infrastructure seamlessly. - Unpredictable Workload Growth
Startups and enterprises alike struggle with unpredictable AI workloads. A sudden increase in data processing or a large-scale model rollout can overwhelm existing infrastructure. Over-provisioning resources to prepare for peaks can be wasteful, but under-provisioning can result in system crashes or bottlenecks during high demand. Navigating this balance is a major challenge, particularly for organizations scaling rapidly. - Reliability Risks During Growth Phases
As companies move from AI pilots to production, ensuring reliable infrastructure becomes essential. Unexpected downtime, performance degradation, or delayed resource availability can cause projects to stall. For enterprises operating across regions, infrastructure failure at critical moments can erode confidence in AI initiatives. In fast-moving industries, any reliability issue can translate to missed business opportunities and lost momentum. - Security and Compliance Challenges
Expanding AI operations across regions and teams introduces new security risks and regulatory hurdles. Role-based access controls, data privacy, and compliance requirements become more complex to manage. Without secure infrastructure and clear governance frameworks, companies risk data breaches, compliance fines, and reputational damage, all of which can slow adoption and delay growth. - Internal Expertise Gaps
Many enterprises lack the in-house expertise needed to manage large-scale AI infrastructure effectively. AI operations require orchestrating complex hardware and software systems, a skill set that’s often scarce. As companies scale, these expertise gaps can lead to inefficient use of resources, slower deployment cycles, and higher operational costs.
Preparing for Sustainable AI Growth
The path to sustainable AI growth requires recognizing these bottlenecks early and building infrastructure that can handle evolving demands. While each organization’s challenges may vary, addressing these core issues will be key to crossing the chasm and unlocking AI’s full potential.
- Build Flexibility into Infrastructure: Companies need infrastructure that can scale dynamically based on workload demands, avoiding both over-provisioning and system overloads.
- Optimize GPU Utilization: Intelligent workload management is essential to reduce idle time and increase efficiency, especially in environments with limited GPU availability.
- Ensure Reliability Through Expert Support: Downtime during growth phases can be costly, making 24/7 expert support and fault-tolerant infrastructure
- Implement Secure, Multi-Tenant Controls: Enterprises must have the tools to manage access securely and comply with regulatory standards across distributed teams and regions.
With the right infrastructure strategy in place, businesses can avoid the bottlenecks that often plague growth phases—empowering them to cross the chasm confidently and unlock the full potential of their AI investments.