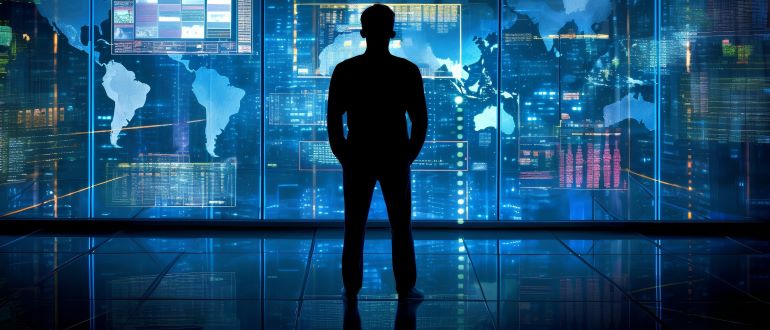
Networks and LLMs really aren’t on the same page. Perhaps, not even reading the same book.
To date, the results of most AI networking initiatives have been lackluster and only scratch the surface of what should be possible. We’ve seen plenty of AI providing answers to why a telco customer’s bill is $20 more this month, but the real value is surely in identifying – preferably proactively – issues in the network, creating suggested resolutions and where appropriate acting on them dynamically and in real-time.
The AI algorithms we are throwing at this problem are wrong; we often don’t have the right data and there is no automated way to apply fixes.
So, let’s unpack these issues a little more, and think through how we overcome each of these.
There is a commonly held view that AI agents are able to answer any question or resolve any issue. However, for an AI agent to be valuable in networking – let’s call it our “Networking Genie” for this example – it has to have two important powers: Omniscience – it has to know everything about your network, and the relationships between everything in your network along with a sense of what “normal” looks like over time.
The second important power is omnipotence. It has to have the power to affect changes in the network – maybe together with a human – in real-time. Without these two powers, it’s no more valuable than Clippy the paperclip, and about as annoying.
It turns out that throwing traditional LLMs at this problem doesn’t work out, because LLMs are simply not well suited to time-series data problems. This isn’t just a networking problem either. There are plenty of timeseries problems in medical data, chemical plant processes and car engines to name just a few. We need a different approach and one that doesn’t need to involve an LLM having an encyclopedic knowledge of the space program or how to make grandma’s special apple pie.
With the emergence of AI, it usually boils down to the ability to understand network logs in a way that’s compatible with AI models. Let’s break down where things stand with networks and LLMs stand right now and where we can go from here.
LLMs and Network Logs
It’s safe to say that there’s no singular AI method or design right now that can help network teams enhance their work productivity or enable their networks to help innovate with these modernization initiatives.
The methods, both foundationally and statistically, need to help LLMs get the right answers to be trusted. There’s a power to having these models and methods all together and tiering them based on need.
For example, imagine sitting in a call center of a major telco network and you get a call that someone can’t post a photo to Instagram because the network is down or incredibly slow. With AI, all we can do at this point is gather information about the person calling, their device and their phone number (and history). But AI alone can’t identify the actual network problem – the main purpose for this person calling.
Do we go down the street knocking on everyone’s house to resolve the issue? Whether it’s congestion, backhaul or nearby outages, each issue makes network teams go to individual elements to get to the bottom of the issue.
Right now, we can’t identify, confirm and isolate an issue like that, and LLMs by themselves can’t understand time (or time-series data) the way that network teams can.
The solution is applying AI technologies to time-series data to then have an LLM create a language around what happened through this combined technology. Ideally, after getting told what happened, we’d like the technology to contextualize why it happened and help resolve it.
If we go back to the call center example, the technology could tell us that a football game with 100,000-person attendance was nearby and slowed down the network – a summary surrounding the problem, context and a path to resolve.
Addressing the Data Problem
Networks, especially telco networks, dish out incredibly large amounts of data in a quick amount of time.
The challenge that comes with this is aggregating the volume of data in real-time – understanding it and making decisions at a massive scale.
Telcos have spent an insane amount of money building telemetry networks while not gaining the deep understand they thought would result. While network teams can’t take in massive volumes of data over a long period of time, they’ll need to have this data funneled in through a smaller format that’s been understood before reaching them.
This collection pre-process becomes very important to maintain a flow of data that is moving as fast as the networks are. Do we need to centralize and collect everything? To me, that creates a sense of bloating. However, the ability to run widely distributed AI algorithms will prevent the need from centralizing everything. Once there’s an understanding of the data, AI needs to take over.
What actions can be taken? Can these actions be pushed to a ticket or automatically resolved? If we have the ability of an LLM to understand different types of data to successfully question the network, we can apply automation to make configuration changes.
Applying Automation to AI-based Recommendations
Funny enough, telcos think the AI part of this idea is easier to figure out than the automation part.
Manual intervention to automation scripts and manuals in the telco industry is rampant, which defeats the purpose of true automation.
For this wonderful AI system to work, collection, telemetry and frameworks all need to be done properly. This is usually done through APIs to push out configuration changes. Whatever the mechanism is, it will be a focal point in the network to drive successful automations.
What’s different is that telcos think of APIs as an external event versus how we think of them – an internal process. This means APIs need to be created at every level in the network to achieve unity.
So now when network teams tell the system to create extra capacity or make a change because the network is busier, the change request is understood and automated to eliminate the one-off changes that naturally occur in the telco industry.
Finding a Happy Medium With Network Automation and LLMs
There’s a lot of work to be done in each of these areas to make this bridge a reality. All sides need to understand the obstacles and learn how each other work to perform at their best individually first. So far, neither side is talking to each other or can’t talk to each other in a way that makes sense.
Automation, telemetry, AI and models need to work together with a pure focus on helping the network teams reduce operational costs, time to resolve issues and to make their customers happy. That outcome will drive more ROI for AI networking in the years ahead.