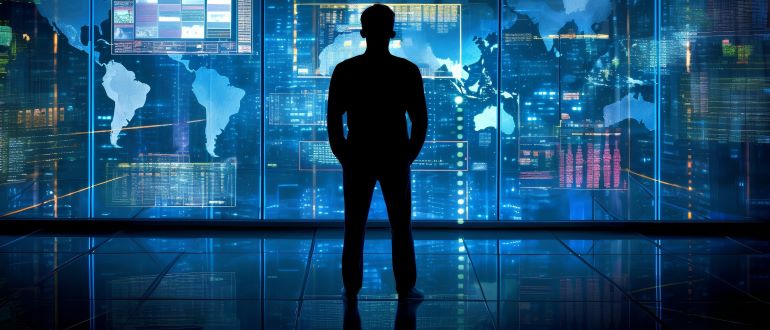
Almost every major organization is committing to generative AI. According to recent Gartner data, GenAI will drive a significant 8% growth in worldwide IT spending in 2024. While some have deployed the technology in full production, most are still in the phases of planning, strategizing and experimentation. Their vision, however, is often clouded by an incomplete understanding of generative AI: Many assume that generative AI simply encompasses LLMs. But there are other forms of generative AI aside from LLMs – namely, LGMs – that can do what LLMs can’t: Analyze tabular, time-series data to forecast business outcomes.
LLMs have certainly shown some clear business value. They’re useful for things like content development, research, document summary and translation. But as many organizations are beginning to discover, LLMs have a number of limitations and challenges.
LLMs Raise Concerns About Cost, Hallucinations, and Privacy
LLMs are resource-hungry. Because of this, running them at scale is extremely costly. One analysis found that for a large organization using GPT-4 to analyze longer documents and ask a million questions per day, yearly costs would range between $28.47 million and $56.94 million per year. More and more organizations have LLM projects stuck in pilot mode after they discover that the cost of running them far exceeds expectations.
LLMs are so resource-intensive because they need to train on massive volumes of data to effectively learn and provide accurate answers. From an infrastructure perspective, collecting and analyzing all this data results in high computing and storage fees. LLMs like ChatGPT had to be trained on the entirety of the web before they were released to the public, costing hundreds of millions of dollars.
LLMs’ need for lots of training data doesn’t just mean higher costs. It also leads to inaccuracies and hallucinations when organizations try to employ them without feeding them sufficient data. Any model will perform badly if not well trained. And LLMs, by their very design, can only gain sufficient training and reliably deliver accurate insights after analyzing and iterating on incredible volumes of data.
Another challenge with LLMs is data privacy and compliance. A survey from Malwarebytes found that 81% of respondents were worried about the security and safety risks of ChatGPT. There are many concerns here. From an enterprise perspective, the biggest issue is that organizations have no way of knowing for certain if the data they’re sharing with LLMs is being kept safe and secure.
Could that data flow into another enterprise’s environment that’s using the same shared cloud infrastructure? Is it being incorporated into the foundational LLM to help further refine it?
The Tech Wasn’t Built for Most Business Data
The shortcomings outlined above are notable. But eventually, they’ll be fixed as major players introduce new innovations to the technology. But once LLMs become cheaper and safer, they’ll still be unsuited for a critical application: Analyzing the tabular, time-series data to predict and optimize business outcomes.
LLMs are designed to model mostly unstructured text data (i.e. web content and social media posts). They aren’t able to effectively collect and analyze highly structured data sources like transaction data, tax data, sensor data, statistical data and IT event data. These types of data sources – not unstructured text data – are essential to describing and modelling business trends.
LGMs Fill the Gap
Luckily, there are alternative generative AI technologies. An LGM (large graphical model) is a model that uses a graph to represent the conditional dependence structure between a set of random variables. LGMs are probabilistic in nature – their goal is to describe and explain the entire joint distribution between all variables of interest. LGMs visualize the complex relationship between different data points. They can then be used to determine how those relationships may change in the future as the data changes.
Boiling it down, LGMs differ from LLMs in three fundamental ways:
- Small data, low costs: LGMs require less data than LLMs and therefore have lower compute and storage requirements, driving down costs. This also means that organizations can get accurate insights from LGMs even with limited training data.
- Modelling tabular, time-series data: Unlike LLMs, LGMs are designed to train on tabular, time-series data. LGMs were created specifically for the purpose of forecasting and making predictions, making them ideal for use cases in industries like retail, finance, health care, and other sectors with lots of tabular, time-series data.
- Safety: LGMs support better data privacy and security. They train only on an enterprise’s own data – with supplementation from select external data sources (such as weather data and social media data) when needed. There is never a risk of sensitive data being shared with a public model.
To bring the benefits of LGMs to life, consider this example: The holidays are approaching. A large retailer with both brick-and-mortar and e-commerce operations is looking to plan for staffing and supply chain logistics for the season. To execute effectively, they need insights for questions like, “How many temporary workers do we need this season?” and “How long on average will it take to get packages to customers?”
In order to answer these questions, they leverage an LGM to analyze sales data, labor data, weather data, postal data and other forms of tabular, time-series data. Based on this analysis, the LGM provides a model that tells the retailer how many employees they should bring on board and how long they should anticipate deliveries will take. It also describes how confident it is in these answers.
Generative AI is More Than LLMs
LLMs have a role within businesses. But there are some hurdles that need to be overcome before they’ll be cheap and practical enough to truly deliver on their promise. And even when those challenges are solved, there will still be tons of use cases that are unserved by LLMs and will never be served by LLMs, as the technology simply wasn’t built for these purposes.
There’s more to generative AI than LLMs, though. LGMs were created specifically to analyze tabular, time-series data to predict outcomes and forecast complex trends. Moreover, LGMs have already solved the problems plaguing LLMs and are ready for full deployment today. The technology is cost effective, secure, requires minimal training data and only needs an organization’s own data to work.