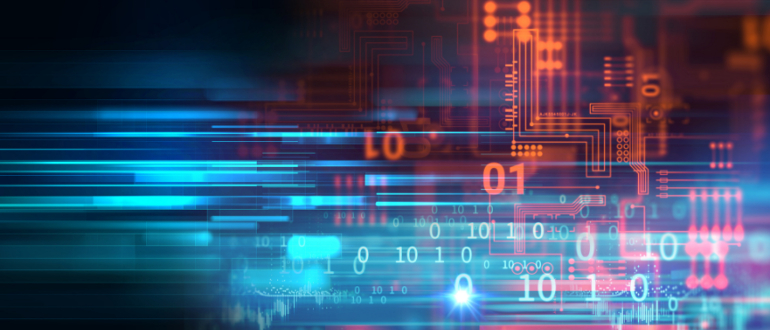
Although AI is rapidly becoming a crucial tool for data analysis, shaping some of the most significant trends in data programs over the past year, just 12% of businesses say their data is AI-ready.
Findings from Precisely’s survey of 565 data and analytics professionals, conducted by the Center for Applied AI and Business Analytics at Drexel University’s LeBow College of Business shed light on the most pressing challenges businesses face in achieving AI-readiness.
These include data quality, data governance and data enrichment, and how they’re prioritizing investments to overcome them.
While more than three-quarters (76%) said the ability to make data-driven decisions is a top goal, 67% reported they don’t fully trust the data their organization is using.
Insufficient Data Governance
The report also revealed insufficient data governance as the top barrier to AI initiatives, while among the emerging trends are data management architectures driven by the demand for democratized data.
Other factors affecting AI readiness include regulatory variations, privacy concerns and compliance requirements.
Respondents report that inadequate tools for automating data quality processes (49%), inconsistent data definitions and formats (45%) and data volume (43%) are the top factors keeping them from achieving high-quality data.
The shortage of skills and resources needed for data management, analytics and AI has grown this year – 42% said a shortage of skills and resources continues to be one of their biggest challenges to data programs, and 61% cited a lack of employee skills and training in AI as a primary reason for their unpreparedness for AI initiatives.
Tendu Yoğurtçu, Ph.D, CTO at Precisely, said to combat these data quality and trust issues, more organizations are prioritizing data governance–with 71% reporting that their organization has a data governance program, compared to 60% in 2023.
“This increased investment is paying off,” she said.
Organizations that invested in data governance programs report benefiting from improved quality of data analytics and insights (58%), improved data quality (58%), increased collaboration (57%), increased regulatory compliance (50%) and faster access to relevant data (36%).
Yoğurtçu said with macroeconomic headwinds at play, many organizations struggle with a lack of skills and resources to implement and manage data programs.
“To combat these challenges, it’s important to get leadership bought into the need to invest in data programs,” she said.
She added the good news is making the case could be easy – the survey results indicate that investments in data initiatives are paying off in helping organizations achieve their top priorities.
Organizations that invested in data programs saw significant returns in data-driven decision-making (64%), operational efficiency (60%) and cost reduction (51%), mirroring the top three data program goals that organizations are most hoping to achieve.
“It’s encouraging to see more businesses are garnering support for data programs, with fewer respondents reporting executive support as a critical challenge compared to last year,” Yoğurtçu said.
She explained that based on the size of the company and the type of industry – for example a highly regulated industry or not – the organization may consider a steering committee or a cross-functional AI council including representation from legal, data privacy office and security office.
“This cross-functional council can help define best practices, provide oversight for all AI initiatives, and help accelerate them by identifying common use cases and patterns across teams,” she said.
“Organizations that promote data and AI literacy can achieve net gains in data quality, collaborative communication, innovation and work process efficiencies,” Yoğurtçu explained.
From her perspective, data-literate business users should understand the importance of proactively monitoring health of their data and actively managing metadata to drive real business value from their data programs and their AI implementations.
She said increasing data literacy includes training business users to understand what data is available to them, how it can be used, and what its limitations may be.
“From there, training should include how data from different sources can be combined, or how it might be enriched with trusted information from third parties and geospatial context,” she said.