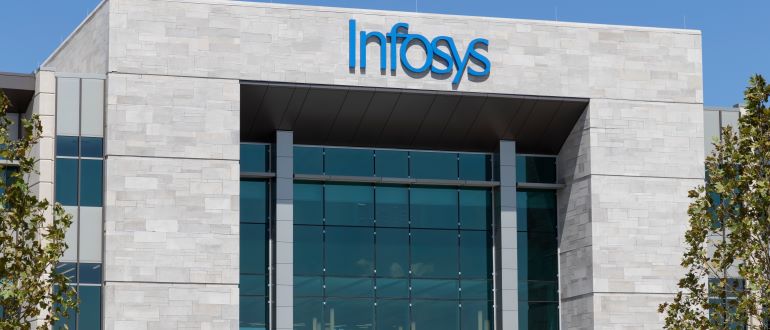
Infosys this week launched a Topaz portfolio of services and tools that promises to make it simpler for enterprises to build and deploy generative artificial intelligence (AI) applications.
Balakrishna D.R, senior vice president for Infosys, said Topaz will make it simpler for organizations to build and extend multiple large language models (LLMs) to automate a wide range of domain-specific processes. That “poly AI” approach will enable organizations to mix and match large and small LLMs and then deploy them on any cloud or on-premises IT environment as required, he said.
Based on the Infosys Cobalt cloud, Infosys is making available more than 150 pre-trained AI models running across more than 10 platforms with more to come. Infosys is also providing access to 12,000 use cases for generative AI alongside use cases for other forms of AI involving machine and deep learning algorithms and data science techniques.
Most organizations will need to rely on additional external expertise to best determine what mix of AI and data science technologies to apply for any given use case. The amount of data science expertise available is still fairly limited, an issue that is only likely to be further exacerbated as interest in domain specific LLMs increases. Each organization will also need to decide to what degree it might make sense to build an LLM from scratch versus using their data to extend an existing LLM to automate a task using processing known as “grounding.”
Of course, providers of cloud services such as Microsoft and Google may be garnering most of the attention when it comes to generative AI, but it may be consulting firms such as Infosys working with enterprise organizations that realize much of the full potential of generative AI within the context of a larger digital business transformation initiative. “We’re working with clients on everything from autonomous stores to predictive maintenance for tires,” said Balakrishna.
A recent Infosys Knowledge Institute report found that 63% of the AI models that have been deployed this far by enterprise only function at a basic level, and only 26% are highly satisfied with their data and AI tools. Generative AI may change that equation by employing natural language interfaces to simplify interactions between humans and machines. The one thing organizations would be well-advised to remember is that there will always need to be humans in the loop to ensure processes augmented by AI are performing as expected in addition to checking to make sure that some form of bias has not been introduced by an AI model, noted Balakrishna.
It may be a while before enterprises are routinely taking advantage of generative AI to automate processes, but there’s no doubt organizations are entering a new phase that will test the limits of generative AI. As these capabilities are made widely accessible the expectation in time will be that any process that doesn’t include some element of generative AI will appear to the average user to be antiquated so, ready or not, a generative AI arms race in the enterprise is now underway.