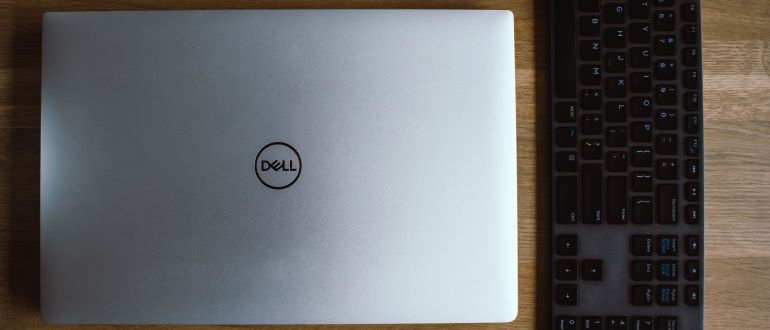
Dell Technologies and NVIDIA today revealed they are collaborating on a Project Helix initiative that, beginning next month, will make it simpler for organizations to build large language models (LLMs) that drive generative artificial intelligence (AI) models within an on-premises IT environment.
Announced at the Dell Technologies World conference, the Project Helix initiative is part of a Dell effort to make a case for building generative AI models in a local data center that is more secure than a public cloud computing environment.
Varun Chhabra, senior vice president for product marketing for the Infrastructure Solutions Group and Telecommunications at Dell, said organizations that are opting to build their own LLMs need to ensure that the IT environment being used to create them is secure. As such, many of them are going to opt to build them in an on-premises IT environment rather than opting to rely on a cloud service provider, he added.
Building generative AI models based on LLMs for specific domains requires much less data than the general-purpose model used to create, for example, ChatGPT. Data science teams are now also leveraging large LLMs to build medium-sized LLMs that will then be used to create small and even tiny LLMs that can be employed in use cases that have limited access to compute resources. NVIDIA feels generative AI has become the killer application for IT infrastructure that enables parallel processing, also known as accelerated computing.
Organizations, however, need to be able to trust the output of generative AI applications built using proprietary or high-value data sets is accurate and consistent, so there need to be guardrails in place, said Chhabra. In addition, most of those organizations are going to be subject to data privacy regulations, he added.
Dell and NVIDIA are pledging to assist organization-built LLMs across every stage of the generative AI lifecycle, said Chhabra.
It’s not clear how many LLMs might be built by the average enterprise but NVIDIA, in addition to needing GPUs to build them, is also making a case for using its GPUs to run the inference engines that AI models rely on to run. Most inference engines are run today on X86 systems, but NVIDIA is making a case for using parallel processing to both build AI models and run them.
Nor is it obvious how many generative AI models will be built in an on-premises IT environment but there are still many highly regulated industries where on-premises IT environments are still the default for building and running applications. One way or another, AI models are going to be pervasively deployed across enterprise applications. The challenge now is making it simpler to build those AI models and then integrate them within a wide range of applications that will be deployed everywhere from the network edge to cloud and everywhere in between.
The bigger challenge, of course, is recruiting and retaining the data science and application development teams needed to build and deploy those applications.