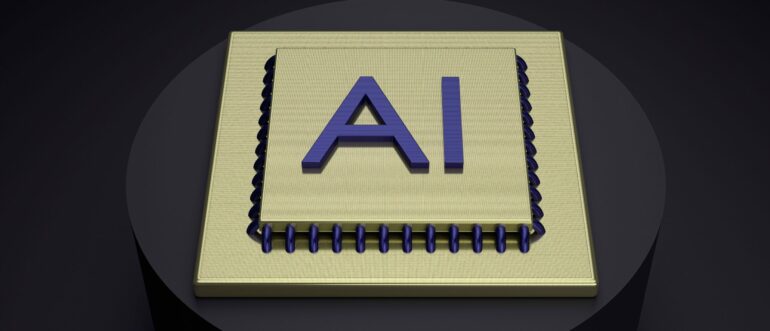
SambaNova in February announced its Samba-1 generative AI model as the first to break the 1 trillion parameter barrier, a claim that was validated last week by testing firm Artificial Analysis and gives another hardware option to an industry that is seeing rapid enterprise AI adoption and tight availability of Nvidia GPUs.
Nvidia’s chips, particularly the Tensor Core H100s, are the go-to accelerators for generative AI workloads, but the booming demand from enterprises to hyperscale cloud providers has made them hard to get and expensive. According to the Motley Fool, there are instances where customers have waited as long as 11 months to receive their orders of the GPUs.
Public cloud giants Microsoft, Amazon Web Services (AWS), and Microsoft are developing their own AI processors to ease the crunch, chip players like Intel, AMD, and Arm are offering portfolios of products for AI workloads, and smaller companies – not only SambaNova, but also Groq, Hailo, Graphcore, and others – are creating accelerators to run large language models (LLMs).
OpenAI CEO Sam Altman reportedly has plans for the company to not only design its own chips but also to build facilities to manufacture them.
The Rewards Can Be Huge
It’s a fast-growing business, with market research firm Statista predicting the global market to expand from $53.66 billion in 2023 to $91.96 billion next year. Companies like SambaNova expect their offerings to give enterprises alternatives to Nvidia’s GPUs that can quickly and efficiently run AI workloads at a lower cost.
“For today’s workloads, this speed [delivered by SambaNova’s Samba-1 Turbo model and its newest chip, the SN40L] will result in immediate efficiencies in application chains used today to solve complex business problems without compromise on quality,” Keith Parker, the company’s director of product marketing, wrote in a blog post. “Today, SINGLE models aren’t able to conclusively solve business problems with quality. Applications delivering real business value are making many model calls as part of an application. These model calls add up to unacceptably slow performance for high-quality answers.”
SambaNova’s SN40L, announced last fall, is the company’s latest reconfigurable dataflow unit (RDU), which can be used for AI training and inferencing in the cloud or on-premises. The Samba-1 model was launched in February as a “composition of experts,” or COE, which means it includes a growing range of AI models. Artificial Analysis ran its test on the Samba-1 Turbo, an API version of the model. The tests showed it could handle 1,000 tokens per second with the Meta’s Llama 3 8 billion parameter model.
Nvidia Alternatives are Expanding
It’s the latest evidence that organizations and developers will continue to see a growing range of AI hardware and software options. In announcing Samba-1 in February, SambaNova co-founder and CEO Rodrigo Liang said the SN40L is the smartest AI to rival Nvidia and that Samba-1 competes with OpenAI’s GPT-4 LLM, adding that “it’s better suited for the enterprise as it can be delivered on-premises or in private clouds so that customers can fine-tune the model with their private data without ever disclosing it into the public domain.”
How this will play out remains to be seen. Nvidia executives more than a decade ago bet the company’s future on AI and it’s worked out. The company has built a range of chips and hardware systems to run AI workloads along with software, developer tools, and a broad array of ecosystem partners. In the first quarter, Nvidia generated $26 billion in revenue, up 262% year-over-year.
In March, CEO Jensen Huang introduced its upcoming Blackwell GPUs for AI workloads and at Computex over the weekend introduced more AI software and services. Others, including AMD and Arm, announced plans for more AI hardware, with Arm CEO Rene Haas reportedly saying he expects 100 billion Arm-based devices to be available for AI workloads by the end of the year.
Challenges and Opportunities
Bob O’Donnell, chief analyst with TECHnalysis Research, told Techstrong.ai that fast AI chips like those from SambaNova and Groq “are certainly cool on a technical level.”
“But the biggest challenge with AI is about software and that’s where Nvidia’s CUDA is arguably even more important than the hardware,” O’Donnell said, referring to Nvidia’s vast software and development platform. “That’s why other big competitors like AMD and Intel, as well as these startup chip companies, are having such a difficult time making a big dent into Nvidia’s huge market share.”
However, the rising demand for more AI compute capacity is creating an opening for other players, according to David Nicholson, an analyst with the Futurum Group.
“The enterprise market has been convinced that the safe bet is Nvidia, but that is being weighed against time-to-market considerations,” Nicholson told Techstrong.ai. “The point of spending money on AI is to create value as soon as possible. Companies that would spend money with Nvidia today are forced to consider options. That pause allows Nvidia competitors to make the case that they are disrupting the incumbent before the incumbent even has a chance to be the incumbent. Enterprise companies are still captured by Nvidia, but I expect margins and share of wallet to move in the direction of alternatives.”
What those companies need to do is demonstrate better ROI for enterprises, in terms of work done per dollar and kilowatt per hour.
“As long as models are sufficiently abstracted from the hardware, Nvidia will not be able to sustain its lock on market share,” he said. “The pace of disruption is greater than ever before and that works in favor of the market in a big way and against Nvidia maintaining margins.”
Nicholson also noted that Nvidia’s ecosystem partners are keeping their options open, either through partnerships with others to building their own chips. In addition, SambaNova is looking to become a platform player through its own bespoke architecture, which would allow it to create its own ecosystem.
That said, the key problem facing the AI industry is the inability to generate enough electrical power.
“Every discussion about AI should immediately sound like a person talking about having a backyard swim party in a pool that has no water,” he said. “We don’t have the power generation capability to support AI. The conversations about building data centers to house these AI factories are almost comical at this point. The future of the economy is based on the success of AI. AI requires power we can’t produce without dramatic changes to energy policy. It would be hilarious if it were not so shocking. Pun intended.”