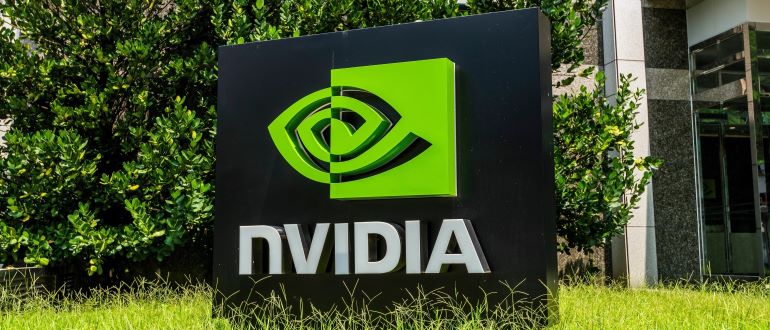
A little more than a decade ago, Nvidia co-founder and CEO Jensen Huang said the GPU maker was going all-in on AI, and that the emerging technology would be foundational to its future plans for products and services.
Nvidia’s GPUs had already made the jump from gaming systems to the data center, giving the company entry into the world of servers and supercomputers. Now Huang’s bet on AI and machine learning is paying off in a big way, supercharged by the explosive emergence over the past 16 months of generative AI and large-language models (LLMs).
That was seen last month, when Nvidia announced financial numbers that showed fourth-quarter revenue jumping 265% year-over-year – reaching $22.1 billion – and yearly revenue growing 126%, to $60.9 billion. Huang attributed the sharp increases to Nvidia’s investments in accelerated computing and generative AI.
Such investments were on display during this week’s GTC 2024 developer conference in San Jose, California, when Nvidia unveiled a new generation of AI-focused GPUs and accompanying network capabilities and software. At the heart of that is Blackwell, a GPU architecture designed to meet the skyrocketing demand for AI processing power.
Enter Blackwell
Nvidia was leading in the AI space GPU with its Hopper H100 chips, which saw high demand and – due to the rapid enterprise adoption of generative AI – steep shortages. However, these new chips – in particular the B200 GPU and the GB200 Grace Blackwell Superchip, which combine two Blackwell GPUs with a Grace CPU connected by an ultra-fast 900GB/s NVLink interconnect – address the need for larger, more powerful and more efficient accelerated computing, according to Huang.
“We are sitting here using synthetic data generation,” the CEO said during his GTC keynote. “We will use reinforcement learning, practice it in our mind, have AI working with AI, training each other just like student and teacher debaters. All of that will increase the size of our model, increase the amount of data that we have, and we will have to build even bigger GPUs. Hopper is fantastic, but we need bigger GPUs.”
The numbers are big as well. The B200, packed with 208 billion transistors, can deliver up to 20 petaflops of FP4 compute power. Huang noted that it would take 8,000 Hopper GPUs – consuming 15 megawatts of power – to train a 1.8 trillion-parameter model. Such a job will take 2,000 Blackwell GPUs and consume only four megawatts.
Meanwhile the GB200 Superchip will deliver 30 times the performance of a H100 GPU to run LLM inference workloads, with the CEO saying it will reduce the cost and power consumption by as much as 25 times. For a model with 175 billion parameters, the GB200 will offer seven times the performance of a Hopper GPU, through it also will deliver up to four times the speed for training.
The Cost of AI Processing
None of this will come cheap. Huang told CNBC that Blackwell GPUs will cost between $30,000 and $40,000, saying that Nvidia needed to “invent some new technology to make it possible” and spent about $10 billion in R&D to make it happen. Such advancements include a second-generation transformer engine that uses four bits per neuron rather than eight, which essentially doubles the compute and bandwidth capabilities as well as the model size. In addition, the newest NVLink switch vastly improves the communication between 576 GPUs via 1.8TB/s bandwidth.
However, Nvidia plans to sell Blackwell chips as part of larger infrastructure stacks, such as its newest DGX SuperPOD supercomputer powered by 36 GB200 GPUs, which will come with a liquid-cooled architecture and deliver 11.5 exaflops of compute performance, so the cost of the actual GPUs will likely vary.
Into the Cloud
In addition, Blackwell will find its way into the cloud. Top cloud providers Amazon Web Services, Microsoft Azure, Google Cloud and Oracle said at the Nvidia show that they will incorporate the GPUs into their infrastructures.
This makes sense, given that the bulk of AI work is being done in the cloud, with most enterprises being unable or unwilling to spend the massive amounts of money it would take to bring the infrastructure, software and talent in-house. According to a report in January by cloud security provider Wiz, more than 70% of organizations use managed AI services and that self-hosted AI software development kits (SDKs) and tools are found in cloud environments. In addition, 42% also are choosing to self-host AI models.
“Blackwell is not a chip,” Huang said. “Blackwell is the name of a platform. People think we make GPUs. And we do. But GPUs do not look the way they used to.”
Nvidia Leans Further into Microservices
Another key AI capability Nvidia introduced was the Nvidia Inference Microservice – or NIM – which is designed to help developers more quickly deploy generative AI applications on their own platforms by bundling everything they need, from AI models to code. NIMs follow other microservices that Nvidia has introduced for AI workloads.
The aim is to help companies that increasingly are moving beyond just testing LLMs and are now deploying them. NIMs put a lot of the integration needs –such as SDKs, libraries, and tools – into microservices for everything from data processing and high-performance computing (HPC) to guardrails and retrieval-augmented generation (RAG), with the microservices offered through Nvidia’s CUDA-X program.
NIMs take “all of the software work that we’ve done over the last few years and puts it together in a package, where we take a model and we put the model in a container as a microservice,” Manuvir Das, Nvidia’s vice president of enterprise computing, told journalists in a briefing before GTC started. “We package it together with the optimized inference engines that we produce every night at Nvidia across a range of GPUs.”
The cloud-native microservices that are optimized for inference work on more than two dozen AI models from both Nvidia and various partners. The NIMs are built atop Nvidia’s CUDA software platform. The company unveiled more than two dozen NIMs for the health care field and as CUDA-X microservices.