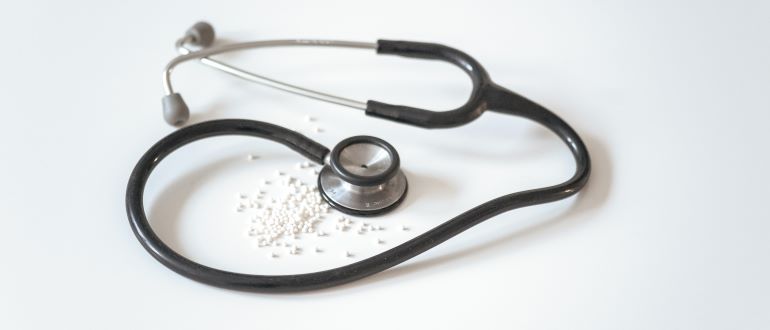
The costs of care are rising, and with them, the pressure on health care systems to manage resources effectively. Between 2019 and 2022 alone, overall hospital expenses surged by 17.5%, while hospital labor costs grew by 20.8%, according to a recent report from the American Hospital Association. This financial strain, coupled with ongoing resource constraints and staffing shortages, has created a perfect storm, making it increasingly difficult for health systems to maintain both financial health and high-quality care. Amid this challenge, AI has emerged as a promising solution, but with it comes the risk of “AI washing”—where vendors exaggerate or falsely claim their products’ AI capabilities.
The buzz around AI in health care is undeniable, with its potential to revolutionize efficiency and optimize operations. However, the rise of AI washing has made it difficult for decision-makers to discern genuinely valuable AI solutions from those that are all hype and no substance. As health care leaders navigate an ever-expanding market of AI offerings, they are often confronted with options that are oversold or misrepresented, making the task of finding truly effective AI tools increasingly complicated.
In this landscape, it has become more important than ever for health systems to establish a robust vetting process to guard against AI washing. By focusing on key factors, health care organizations can better navigate the AI marketplace, ensuring that the solutions they choose are not only effective but genuinely powered by AI. Here are some essential considerations to help you distinguish real innovation from mere marketing:
Expertise
- Team Composition (Technical Expertise and Domain Expertise): Evaluate the team’s AI expertise and credentials. A strong team should include roles like Chief Data Scientist or Chief Decision Scientist, and employees with doctoral degrees in STEM fields, indicating deep technical knowledge. Pay particular attention to how long they have been developing AI solutions and their experience in the health care sector.
A well-rounded team should include domain experts who understand the intricacies of health care. Ensure the team includes clinical experts who are relevant to what is being predicted or modeled. These domain experts should have a deep understanding of health care’s complexities, ensuring that the AI solutions are both technically sound and clinically meaningful. For example, inpatient nurses who can validate the right type of proactive patient alerts.
- Assess Logic and Use Case: Assess the logic and use case and confirm there is compelling reasoning to use AI as a solution. Make sure the vendor can articulate the benefits of AI beyond buzzwords, providing a clear and logical explanation of how their AI solution addresses specific problems and how the solution provides actionable insights.
Involve your technical team in evaluating the solution. Be wary of companies that have recently jumped on the AI bandwagon without substantial expertise in machine learning and AI in general. Typically a problem is a good candidate for a machine learning solution if current solutions require extensive manual hand tuning, or a long list of business rules that need to be maintained and updated.
Track Record
Ask for case studies and customer testimonials to verify that the models work in the wild and that reputable customers have benefitted from the solution. Speak to current customers about their experience to gain insight into the practical benefits and challenges of the AI solution. Inquire about how many customers the vendor serves, as it’s harder (and a sign of credibility) to replicate sustainable and scalable success across a larger customer pool.
Additionally, check for specific metrics that showcase the AI’s performance improvements, such as increased efficiency, cost savings or enhanced accuracy. Make sure that any metrics regarding the performance of the model can be compared to a viable baseline, demonstrating clear, measurable improvements over existing solutions.
Data and Application Infrastructure
- Data Quality: Understand the data requirements for the model to solve the problem. Check that the required data makes sense to generate a solution to the problem being addressed. If it does not, the vendor should be able to supply a satisfactory explanation, as there may be counter intuitive insights given by certain data sets. There should be a robust process for verifying and cleansing input data.
- Transparency and Ongoing Monitoring: Understand how the model learns from new data and how frequently it is updated. Model drift occurs when there has been a change in the properties of the underlying data on which the model has been trained. For example, think of a new practice being added to a unit that results in a different patient population to what has been seen before. Or construction has occurred that adds capacity to a unit. In both cases the old model will not work well as it has been trained on an older data set that does not capture the new characteristics. The vendor should have a rigorous process in place to protect against model drift.
Confirm the vendor has a robust system for monitoring the AI’s performance to ensure it continues to meet expectations. This includes regular evaluations and adjustments based on new data and changing conditions. There should be transparency into the accuracy trends of the system provided through dashboards or regular reports.
- Integration and Usability: Assess how well the AI solution integrates with existing systems and workflows. Usability is crucial, the solution should be user friendly for both technical and non-technical staff. Ensure the vendor provides adequate training and change management where necessary.
Security and Governance
- Regulatory Compliance and Security: Ensure the vendor has a solid approach to bias mitigation. Inquire about their methods for identifying and correcting biases.
Verify the vendor’s data privacy and security measures. They should comply with relevant regulations and best practices to protect sensitive information, ensuring the confidentiality and integrity of patient data.
- Governance and Safety: Ensure that the AI solution includes clear guidelines for responsible use, with results that are clearly labeled and expressed with appropriate degrees of certainty. It’s important that the system provides transparency about how confident it is in its predictions or recommendations. Additionally, establish clear lines of responsibility for any actions taken based on AI outputs. For instance, if the AI autofills a surgical form, it should be clear who is responsible for reviewing and approving these entries to prevent errors.
Inquire about the safety protocols in place to prevent the AI from making critical mistakes, particularly in high-stakes situations. This might include human oversight mechanisms or limitations on the AI’s decision-making autonomy. These measures are essential for ensuring that AI is used safely and effectively within your healthcare system.
In the crowded landscape of AI solutions for health care, distinguishing genuine AI products from those merely riding the hype is crucial. By focusing on the factors above, health care decision-makers can make informed choices and avoid falling victim to AI washing.
Implementing a thorough vetting process will help ensure that the selected AI solutions deliver real, measurable benefits and contribute to the overall efficiency and quality of care.