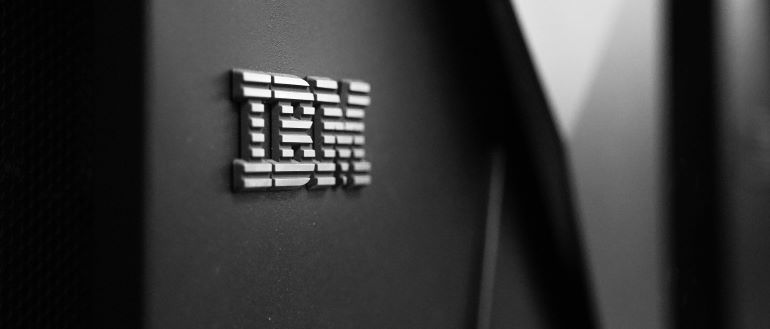
IBM next month will make generally available a data governance toolkit for artificial intelligence (AI) models to provide more transparency into recommendations being generated.
The goal is to reduce the level of risk that would otherwise be created when the data used to train an AI model is not validated using a wastsonx.governance toolkit, says Edward Calvesbert, vice president of product management for the watsonx Platform.
The watsonx platform provides access to a mix of proprietary, open source and custom large language models (LLMs) alongside a range of other types of AI models to build and deploy applications. The watsonx.goverance toolkit can be applied to any of the models to explain how results were generated and surface any potential bias, says Calvesbert.
As organizations move to operationalize generative AI models, they are looking to reduce the chances an AI model will create erroneous answers, otherwise known as hallucinations, by making sure the data used to train the AI in the first place is accurate.
IBM is addressing that issue via a toolkit that makes it simpler to first validate and then monitor models as they are built and continuously updated, says Calvesbert. The wastsonx.governance toolkit provides a framework for managing that process across the lifecycle of an AI model, he adds.
Those types of tools will soon be crucial as governments around the world increasingly regulate how AI models can be applied, says Calvesbert. Complying with those regulations will prove challenging as organizations not only invoke foundational models, but also find themselves managing models that were created as a subset of another model. In addition, organizations will also find themselves managing models optimized for specific tasks that have been networked together to drive a business process.
It’s still early days so far as the building of applications infused with AI models is concerned but, ultimately, teams of AI builders that have multiple types of expertise will need to collaborate, notes Calvesbert. Those teams will include data governance specialists to validate the AI models built, because the same individuals that created a model can not also be the ones to evaluate whether it meets transparency requirements, he says.
In essence, organizations will need to ensure best practices for building and deploying AI models by making sure separation of duties are maintained, adds Calvesbert.
Each organization will need to determine for itself how to construct workflows spanning data scientists, application developers, software engineers, cybersecurity teams and compliance specialists. The one thing that is certain is it won’t be long before organizations find themselves managing hundreds of AI models that either they or a third-party developed. The challenge, as always, is bringing order to a potentially chaotic process that, in addition to wasting limited resources, represents a significant risk to the business – given the potential for regulatory fines to be levied.
Hopefully, most organizations will get ahead of this issue before fines are imposed, but if history is any guide, it usually requires one entity or another to be made an example of before business leaders understand what is absolutely required (whether they like it or not.)