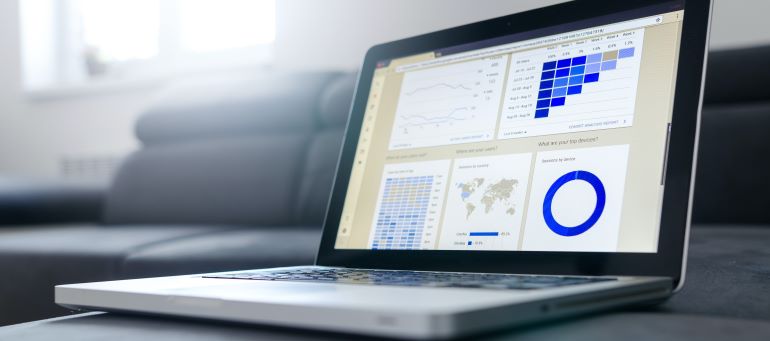
Organizations – like seemingly everyone else in the world – were quick to embrace generative AI when OpenAI launched its ChatGPT chatbot almost 13 months ago.
With large language models (LLMs) as their foundation, generative AI tools like ChatGPT, Google’s Bard and Anthropic’s Claude could be trained on the internet and other publicly available information, drawing on vast amounts of data to come up to the responses for even the most complex prompts.
However, executives and data scientists found that such technologies were limited in their usefulness if they couldn’t incorporate the proprietary corporate data of their users. Vendors over the last several months have started to answer the call. In March, Nvidia rolled out NeMo – for enabling companies to augment their LLMs with their own data – and NeMo Guardrails to include safety and security functions.
Two months later, Nvidia and ServiceNow announced a partnership that included this concept, OpenAI in November unveiled a new platform to enable companies to create custom versions of ChatGPT, and being able to train on proprietary data was a driver behind Amazon’s launch later in November of its Q chatbot.
“It’s critically important for companies to be able to combine their data, insights and strategies with the advanced capabilities of large language models and other AI [and machine learning] technologies to provide a simple user interface for tapping into these capabilities and generate actionable insights from their data that are relevant to their business context and use cases,” Pete Reilly, COO at AnswerRocket, told Techstrong.ai.
Making the Shift to LLMs
This 10-year-old company specialized in augmented analytics until the rise of LLMs, when it shifted to focusing on AI copilots, interfaces used to support generative AI users. AnswerRocket’s Max is an AI assistant for analytics workloads, and when users needed a custom AI offering, the vendor would create it and deploy it to Max, Reilly said.
AnswerRocket this week rolled out Skill Studio, a platform enabling customers to create custom AI analysts that understand their industries, can be personalized to specific businesses, departments and roles, and can run domain-specific analyses of their data.
Skill Studio addresses the needs of data scientists by democratizing their work and scaling it across their companies, and it enables business users to more easily run analytics workloads, Reilly said.
“Data scientists and analysts can create custom reusable Skills, incorporating their specific business rules, analysis techniques, and processes,” he said. “Max uses these Skills to run analyses and generate insights, all delivered via a user-friendly chat interface.”
AI Aligned with Business Needs
This level of customization results in an AI copilot for end users that can answer complex questions and provide data-driven insights aligned with how their company analyzes and talks about data, Reilly added.
Skill Studio enhances Max with such features as a full development environment that allows developers to user the Git provider and IDE of their choice, a low-code user interface for creating Skills for end users, reusable code blocks for accelerating development, and the ability for enterprises to deploy their own machine-learning algorithms in Max.
It’s also a single tool that analysts can use to run analyses with structured and unstructured data and multiple data sources, create copilots for specific roles, and validate the accuracy of answers generated by Skill via a testing framework.
Democratizing AI Analytics
It will give a boost to business users, who have struggled to adopt traditional analytics tools because of the technical learning curve and a lack of the necessary data literacy, Reilly said. Copilots let these users pull deep insights from data via a user-friendly chat experience enabled by LLM integration and designed for interacting with data and insights.
“The reality is generic AI tools often fail to provide the deep, actionable insights that businesses need,” Reilly said. “Skill Studio changes the AI copilot game, going from generalized genAI analytics to a customizable AI assistant that can take on highly specialized data analysis.”
He added that it “all comes together to enable an easy-to-use AI copilot that feels like it understands your business and ways of working, and that’s able to take on a broader set of analytical tasks than has been previously possible.”
Reilly expects the trend toward specialized generative AI-powered copilots for analytical tasks to continue into 2024, with the assistants becoming the preferred tool for non-technical business users.
“So far, many of the genAI tools on the market are not purpose-built,” he said. “They cater to a broad range of audiences as opposed to domain-specific tasks. This changes in 2024, when we can expect to see an increased demand for verticalized AI solutions.”