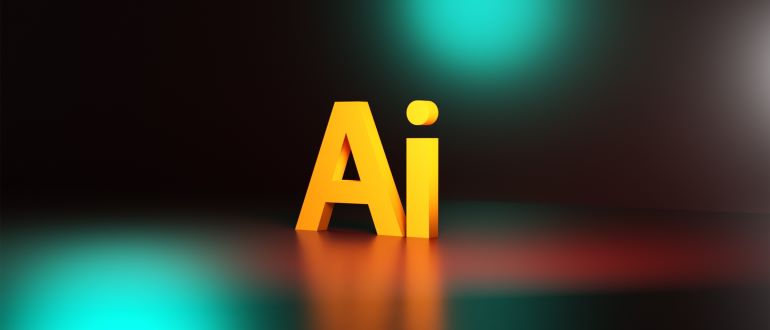
Artificial intelligence (AI) is poised to lead the tech landscape in the year ahead, with 58% of technology leaders identifying it as the most critical area of technology by 2025, according to an IEEE report.
The findings reflect a continued surge in AI adoption, with 91% of all survey respondents anticipating that AI innovation will accelerate as organizations deepen their commitment to leveraging AI’s transformative potential.
While enthusiasm for AI continues to grow, the report underscores the importance of keeping human-centric considerations at the forefront.
The report cautioned generative AI (GenAI) has the potential to create harmful, biased or offensive content unintentionally.
As organizations rely more heavily on AI, the need for responsible and ethical AI use grows equally pressing, with leaders urged to ensure safeguards and transparency in AI-driven processes.
The IEEE study highlighted a broader sentiment that AI will not only drive innovation but will also redefine how organizations operate, engage with customers, and make data-driven decisions.
However, balancing technological advancements with responsible governance remains a fundamental challenge as AI adoption deepens worldwide.
Houbing Herbert Song, IEEE Fellow, explained among the specific challenges organizations will face are safety, security and trustworthiness in developing and using AI systems incorporating GenAI.
He said there are steps organizations can take to address accuracy issues, transparency and the ethical implications of deepfakes.
“The first step is identifying deepfakes,” he explained. “Effective and efficient deepfake detection techniques are crucial.”
From his perspective, biased AI will pose a detriment to the projected growth of AI in 2025.
“To mitigate these bias issues in organizational use, organizations should understand why bias exists in AI and its impacts, beware of challenges to address bias, develop a strategic plan and execute strategic actions,” Song said.
Considering the growing importance of transparency and public perception around AI, he said he sees a role for organizations to educate their stakeholders about AI’s limitations and potential biases.
“Organizations should take the lead in promoting AI’s operations alignment with societal expectations and human intentions in AI’s operations,” he said.
Song said the overarching strategy should the safe, secure and trustworthy development and use of AI.
“Organizations should keep this overarching strategy in mind in balancing excitement and need,” he said.
David Ly, CEO and founder of Iveda said to ensure fairness and minimize AI bias, leaders adopting and deploying AI technologies must adopt proactive measures including diverse and representative data collection.
“Collecting diverse and representative datasets is crucial to training unbiased AI systems,” he explained. “Data should be inclusive, encompassing different demographic groups and perspectives.”
He noted efforts should be made to avoid underrepresented or marginalized groups from being excluded from the data.
Organizations must also undertake rigorous data preprocessing, with leadership and teams thoroughly examining training data to actively identify and mitigate biases.
“This could involve removing personally identifiable information and conducting statistical analyses to uncover hidden biases,” Ly said. “Additionally, data augmentation techniques can help create more balanced and representative datasets.”
He added the regular monitoring of AI systems is essential when it comes to detecting and rectifying bias.
“Implement mechanisms that allow for ongoing evaluation of algorithms’ performance, specifically with respect to fairness and bias,” he recommended.
Bias audits, transparency reports and third-party audits can be valuable tools throughout this process.
Ly said transparency and explainability in AI systems should also be prioritized.
“Users and stakeholders should be able to understand how decisions are made, providing them with an opportunity to challenge or correct biases when identified,” he said. “Not to mention, transparent processes instill trust and allow for accountability.”
Diverse and Inclusive Development Teams: Foster diverse and inclusive teams responsible for developing and deploying AI systems. Diverse perspectives can help identify and rectify potential biases early in the development process. Encourage interdisciplinary collaboration to incorporate ethical considerations throughout the AI lifecycle.
Regular Bias Testing: Incorporate bias testing throughout the development cycle. Evaluate AI systems using diverse and representative test datasets to identify any discrepancies or disparities. Continual monitoring and testing can help maintain fairness and address biases that may emerge over time.