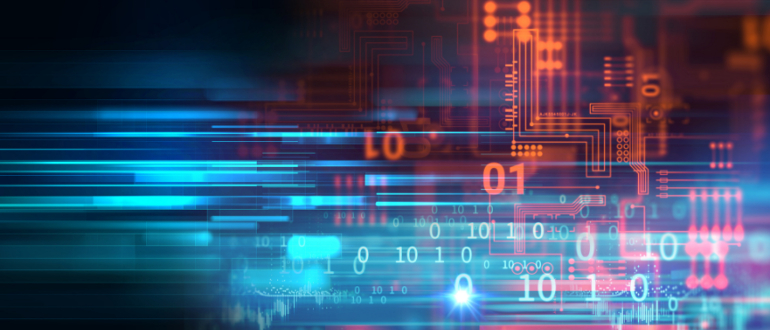
In today’s fast-paced business environment, artificial intelligence (AI) is no longer a luxury — it’s a competitive necessity. Whether it’s elevating customer experiences (CX) or making smarter business decisions, the success of AI initiatives hinges on one thing — data infrastructure.
Explore how infrastructure can handle the demands of advanced AI and learn the foundational steps to set an organization up for scalable, sustainable AI success.
The Importance of Data Quality
AI algorithms need high-quality data to learn patterns more effectively. Poor data quality can lead to inaccurate predictions, flawed insights and costly mistakes. For businesses aiming to scale their AI efforts, ensuring data accuracy, consistency and completeness is paramount. Here are some key considerations for data quality:
- Data accuracy: AI systems rely on accurate data to make correct predictions. Inconsistent or erroneous data can skew results, leading to suboptimal decisions.
- Data completeness: Incomplete datasets may not provide AI models with enough information to recognize patterns or draw reliable conclusions. Professionals must ensure comprehensive data collection.
- Timeliness and relevance: Real-time data is crucial for AI applications that require up-to-date information, such as chatbots, personalized customer interactions and dynamic pricing models.
Data Integration and Interoperability
The complexity of AI systems increases as businesses scale, necessitating seamless data integration from various sources. A well-integrated data infrastructure allows AI applications to draw from a wide range of structured and unstructured data, improving their performance and scalability. For effective data integration, take note of these strategies:
- Data silo elimination: Break down silos to create unified data sources that feed AI systems. When data is centralized, it enables faster processing and better decision-making.
- API-driven architecture: Application programming interfaces (APIs) ensure seamless data flow between systems, making it easier to integrate third-party applications and datasets.
Data Governance: Ensuring Compliance and Accountability
As AI initiatives expand, maintaining control over data governance is essential for compliance and accountability. Poor governance can lead to data breaches, regulatory noncompliance and loss of customer trust. Here are the components of a strong data governance framework:
- Data privacy and security: With growing concerns around data privacy, especially in regions with strict regulations such as the General Data Protection Regulation (GDPR) and the California Consumer Privacy Act (CCPA), businesses must implement data governance practices to ensure compliance.
- Data ownership and stewardship: To ensure accountability, assign clear ownership for data assets. This includes creating policies for data access, modification and deletion.
- Audit trails: Maintain comprehensive audit logs to monitor data use and ensure adherence to governance policies.
Scalable Storage and Computing Power
The ability to manage vast amounts of data — as well as high-performance computing resources — is critical for AI applications to function effectively. As AI workloads increase, businesses need scalable storage solutions and computing resources:
- Cloud storage: Cloud platforms like Amazon Web Services (AWS), Microsoft Azure and Google Cloud offer scalable storage that grows with data needs. Cloud solutions provide elastic storage capacity, ensuring businesses can store large datasets without overinvesting in physical infrastructure.
- Data lakes and warehouses: Data lakes allow businesses to store both structured and unstructured data at scale. When paired with data warehouses, which are optimized for query processing, this setup offers flexibility in handling diverse AI use cases.
- GPU and TPU accelerated computing: AI models — especially deep learning algorithms — require significant computational power. To handle intensive workloads, businesses should invest in high-performance computing resources like graphics processing units (GPUs) or tensor processing units (TPUs).
- Serverless computing: Cloud-based serverless computing allows businesses to scale their processing power automatically based on demand, providing flexibility and cost-efficiency.
Leveraging Cloud and Edge Computing
Cloud technologies and edge computing are essential for businesses to develop scalable AI infrastructures. They provide storage and processing power, as well as the flexibility and security needed to handle real-time data and decision-making processes.
- Elasticity and cost efficiency: Cloud services enable businesses to scale up or down their resources based on usage, allowing them to manage costs effectively while meeting AI demands.
- Global availability: Cloud platforms offer global reach, letting businesses deploy AI applications closer to their users, which improves response times and enhances customer experiences.
- Decentralized processing: Edge computing enables data to be processed closer to its source, reducing latency and bandwidth usage. For applications that require real-time insights — such as predictive maintenance or personalized customer interactions — edge computing is essential.
- Enhanced security: By processing data locally, edge computing minimizes the risk of exposing sensitive information during data transmission, offering additional security layers for AI applications that handle private data.
The Market Impact of AI: Why Scalable Infrastructure Matters
Recent market trends demonstrate the growing importance of AI. In the second quarter of 2024, the S&P 500 Index advanced by 4.3%, mainly due to the strong performance of AI-driven mega-cap technology stocks.
However, broad market participation was weak, with many sectors and regions experiencing negative returns. This disparity emphasizes how AI-focused investments fuel growth while businesses that fail to scale their AI infrastructure risk falling behind.
A Scalable Foundation for AI Success
Building a scalable data infrastructure is critical for businesses aiming to capitalize on AI-driven innovation. Prioritizing data quality, integrating diverse sources, enforcing strong governance, adopting scalable storage and computing resources and leveraging cloud and edge computing can help businesses ensure their AI initiatives are poised for growth.