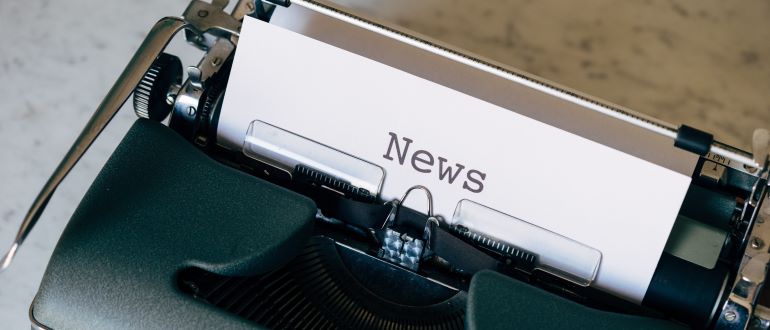
While there is naturally a lot of focus these days on generative artificial intelligence (AI), one of the unappreciated aspects of AI is how large language models (LLMs) will be used to make predictive AI models more accessible.
Pecan AI today announced it has added a Predictive GenAI tool to a platform through which it already makes available predictive AI models. The goal is to make it simple for end users to employ a generative AI tool to invoke predictive AI models using a natural language Predictive Chat tool that defines the model required. It then uses that model to enable a Predictive Notebook to launch a SQL query against a predictive model to, for example, predict customer churn.
The overall goal is democratized data science using a unified framework that combines generative and predictive AI, says Pecan AI CEO Zohar Bronfman.
While generative AI platforms can be used to automate a wide range of tasks, they are not designed to make predictions. That capability requires access to a different class of AI models. The challenge is those models have historically been accessible to a relatively small number of specialists that have data science expertise. The Predictive GenAI tool, for the first time, enables end users to self-service their own predictive AI needs with having any AI expertise, says Bronfman. “It’s a no-touch platform,” he adds.
In effect, Pecan AI has created a composite Al framework to integrate multiple Al models to create a more comprehensive and robust set of capabilities that complement each other. That approach leverages the strengths of different types of AI models optimized for different tasks.
As is the case with any IT platform, organizations will need to decide for themselves to what degree they will want to build AI models versus consume their output as a service. Regardless of approach, generative AI is democratizing access to a wide range of technologies that previously required specialists to invoke. Predictive AI models are only going to be one in a series of technologies that, thanks to natural language tools, are becoming more accessible.
Less clear is to what degree organizations are relying on predictive AI models that are constructed using machine learning algorithms. The reliability of the output of those models is directly tied to the quality of the telemetry data being collected. Fortunately, the quality of that data continues to steadily improve as more organizations embrace modern data engineering processes.
In the meantime, as the number and types of AI models that can be employed continues to increase more organizations might want to consider appointing a chief AI officer to help operationalize them. After all, while building and deploying AI models still represents a significant technical challenge, the bigger issue is going to be overcoming the cultural hurdles that will inevitably arise as AI models are embedded into workflows. After all, launching a query against an AI model is one thing; trusting the output that comes back is often quite another issue altogether.