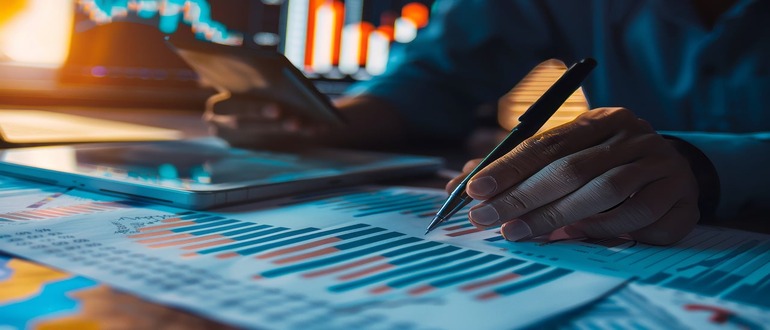
Artificial intelligence (AI) has been revolutionizing business analytics processes for years now. Revenue forecasting, customer sentiment analysis, and conversational data querying are a few of the many AI-enabled capabilities of a modern business analytics workflow. Teams can automate administrative tasks like reporting and reduce the time to insights. Even non-technical users can run their analyses in natural language.
And there’s more to come. AI researchers across the globe are still pushing the limits of this technology by upgrading models, introducing new hardware, integrating services across apps and data sources, and discovering novel approaches. These can further elevate any brand’s analytics process.
In this article, let’s look at five upcoming innovations that AI can bring to business analytics workflows.
The Future of AI-Enhanced Business Analytics
1. RAG and Vectorization
Retrieval-Augmented Generation (RAG) connects large language models (LLMs) with external data sources, like word documents, spreadsheets, and folders, to give context-rich responses.
Vectorization aids in this process by converting the unstructured data in files and documents into numerical vectors. These vectors capture semantic meaning, allowing the LLM to “understand” the content.
These technologies can be used by modern businesses to elevate data access and usage. For instance, teams can quickly find specific facts or data points without going through the files on their drive. They can query the AI chatbot, which will fetch the details for them. They can also run queries that involve merging internal data with external sources.
RAG and vectorization generate source-backed answers swiftly, reducing the time to insights. This empowers professionals to perform dynamic analyses to extract on-demand insights.
Traditionally, organizations had to convert data from their files into a structured format so that their analytics software could “understand” it. Now, they no longer need to manually convert the files into a structured format.
As AI models continue to improve, these technologies will lead to more responsive and intuitive analytics tools for companies. Teams can query in natural language, expediting the process further.
2. Embedded Analytics with Deeper Integrations
Business analytics often requires its own dedicated software packages. Traditionally, professionals had to manually upload data from whatever app they work in to their analytics tools. Thankfully, this has been largely solved by APIs and third-party software integrations.
However, teams still need to go to a different interface to make sense of their data. It can lead to back and forth, which is inefficient. AI-enabled analytics can tackle this by bringing analytics into the tools organizations use on a daily basis.
For instance, companies can spot high-intent prospects in their sales pipeline by embedding analytics into their CRMs. Professionals will instantly know who to message first when they open the CRM interface.
The good news is that it isn’t that far away. Other business processes, such as customer support already leverage this embedded analytics to a certain extent. Support executives, for example, get reply recommendations from LLMs when messaging with a customer.
Industry leaders realize the importance of this technology and are working actively to make it a reality.
Avi Perez, CTO and co-founder of Pyramid Analytics, explains that “The process of embedding analytics is now a top-tier demand, and it comes with its own set of problems and complexities,” by underscoring the requirements of organizations.
“Organizations have been striving heavily to put analytics in the context of their business applications and workflows rather than treat it as a separate set of tools and functions running in its own sandbox,” he says.
3. Transparent AI
AI is already in multiple business processes and workflows. Professionals are becoming increasingly reliant on AI-enabled solutions to make decisions and complete tasks. Most importantly, AI helps companies determine how to deal with people.
Therefore, it is critical that all stakeholders trust the efficacy of the AI solutions, understand the corresponding processes, and help uphold ethical standards through active participation.
Various organizations are starting to invest in this area through employee education. Teams can easily learn how their AI-powered analytics tools operate within their workflows. Another key element of transparent AI implementation is ensuring that the clients, customers, or users know how their data is collected, used, and managed. Apart from earning the trust of the buyers, this is important for legal compliance.
Researchers and developers working on these AI analytics tools for businesses are working toward this objective as well. AI-enabled analytics software can offer explainability by default in the future.
Teams can see why an LLM suggested an action, the confidence level, and the suggestion’s historical accuracy. People-facing industries, such as finance, healthcare, or HR, can greatly benefit from these capabilities to support better decision-making.
The above initiatives — employee and customer education, and built-in explainability — can bring transparent AI into business analytics workflows in the near future.
4. Agentic Capabilities for Taking Action
There are plenty of administrative tasks in modern business analytics processes. Generating recurring reports, sending alerts and updates, documenting progress, and managing tools in the tech stack are a few of the many “boring” action items.
While these tasks are simple and follow a pattern, they demand keen attention to detail. It costs analytics teams a chunk of their day, which could’ve been spent on strategic initiatives.
AI agents can now perform these operations by following predetermined workflows. For instance, an AI agent can analyze a revenue report to extract insights relevant to each department in an organization. Then, it can send alerts to corresponding professionals in a data-backed context.
Teams just have to specify the conditions to guide the AI agent correctly.
Typical automation processes involve static triggers. It is quite common in email marketing processes. “If the form is filled, send a welcome email.” However, agentic AI automation goes beyond that. It can consider dynamic triggers and conditions as well. This makes it capable of detecting anomalies and solving unexpected problems on the fly.
5. AI-Driven Metrics Layer
The “metrics layer” refers to the relative importance of different parameters in a dataset.
Consider a real estate company estimating the price of some properties. The agents will look at various parameters, such as the location, area, and design. However, not all these parameters are weighed equally.
Similarly, when analyzing business data, each parameter on the dataset is valued differently. This is known as the metrics layer.
The metrics layer is applied to the dataset before the actual analysis begins. Often, business analytics teams manually create the metrics layer. They consider different factors, such as the nature of insight and available data, while creating one.
Creating a metrics layer is a resource-intensive process. Organizations have to spend days, if not weeks, to map a layer with high confidence. This limits the diversity of analysis professionals can run.
AI can expedite this process by standardizing metrics definitions across assets, suggesting relevant KPIs based on historical data, and spotting inconsistencies and anomalies. Organizations can then run all sorts of analyses to extract innovative insights. Moreover, thanks to conversational LLMs, non-technical stakeholders can create and use new AI-driven metrics layers as well.
Wrapping Up
AI has already transformed business analytics in many ways. As technology continues to improve, anticipating upcoming advancements can help companies adopt and implement them seamlessly.
In the near future, teams can connect LLMs to organizational datasets for contextual analytics in real time. Embedded analytics can streamline this further by giving insights right within the daily operational tools’ interfaces.
Considering the growing role of AI in handling data, there’ll be more emphasis on transparency and ethics. Another AI innovation in business analytics is AI agents that can perform routine tasks to free teams up for strategic initiatives.
Finally, the AI-enabled metrics layer will enhance the breadth and scope of analytics for organizations across departments.